Eliezer Yudkowsky argues that forecasters err in expecting artificial intelligence progress to look like this:
…when in fact it will probably look like this:
That is, we naturally think there’s a pretty big intellectual difference between mice and chimps, and a pretty big intellectual difference between normal people and Einstein, and implicitly treat these as about equal in degree. But in any objective terms we choose – amount of evolutionary work it took to generate the difference, number of neurons, measurable difference in brain structure, performance on various tasks, etc – the gap between mice and chimps is immense, and the difference between an average Joe and Einstein trivial in comparison. So we should be wary of timelines where AI reaches mouse level in 2020, chimp level in 2030, Joe-level in 2040, and Einstein level in 2050. If AI reaches the mouse level in 2020 and chimp level in 2030, for all we know it could reach Joe level on January 1st, 2040 and Einstein level on January 2nd of the same year. This would be pretty disorienting and (if the AI is poorly aligned) dangerous.
I found this argument really convincing when I first heard it, and I thought the data backed it up. For example, in my Superintelligence FAQ, I wrote:
In 1997, the best computer Go program in the world, Handtalk, won NT$250,000 for performing a previously impossible feat – beating an 11 year old child (with an 11-stone handicap penalizing the child and favoring the computer!) As late as September 2015, no computer had ever beaten any professional Go player in a fair game. Then in March 2016, a Go program beat 18-time world champion Lee Sedol 4-1 in a five game match. Go programs had gone from “dumber than heavily-handicapped children” to “smarter than any human in the world” in twenty years, and “from never won a professional game” to “overwhelming world champion” in six months.
But Katja Grace takes a broader perspective and finds the opposite. For example, she finds that chess programs improved gradually from “beating the worst human players” to “beating the best human players” over fifty years or so, ie the entire amount of time computers have existed:

AlphaGo represented a pretty big leap in Go ability, but before that, Go engines improved pretty gradually too (see the original AI Impacts post for discussion of the Go ranking system on the vertical axis):
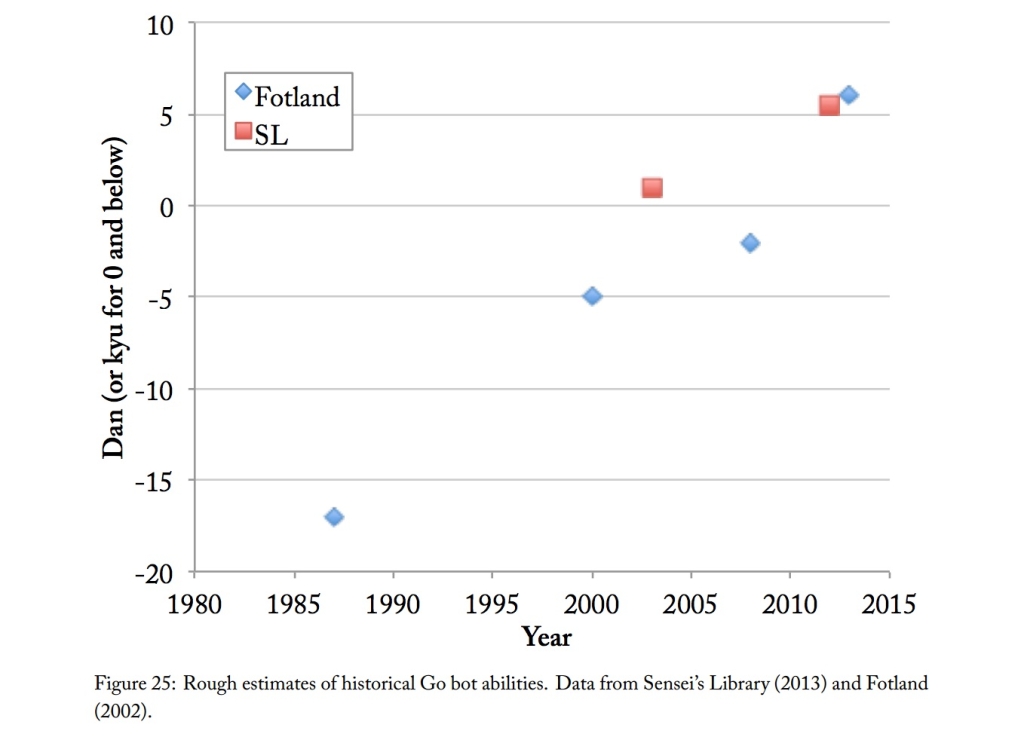
There’s a lot more on Katja’s page, overall very convincing. In field after field, computers have taken decades to go from the mediocre-human level to the genius-human level. So how can one reconcile the common-sense force of Eliezer’s argument with the empirical force of Katja’s contrary data?
Theory 1: Mutational Load
Katja has her own theory:
The brains of humans are nearly identical, by comparison to the brains of other animals or to other possible brains that could exist. This might suggest that the engineering effort required to move across the human range of intelligences is quite small, compared to the engineering effort required to move from very sub-human to human-level intelligence…However, we should not be surprised to find meaningful variation in the cognitive performance regardless of the difficulty of improving the human brain. This makes it difficult to infer much from the observed variations.
Why should we not be surprised? De novo deleterious mutations are introduced into the genome with each generation, and the prevalence of such mutations is determined by the balance of mutation rates and negative selection. If de novo mutations significantly impact cognitive performance, then there must necessarily be significant selection for higher intelligence–and hence behaviorally relevant differences in intelligence. This balance is determined entirely by the mutation rate, the strength of selection for intelligence, and the negative impact of the average mutation.
You can often make a machine worse by breaking a random piece, but this does not mean that the machine was easy to design or that you can make the machine better by adding a random piece. Similarly, levels of variation of cognitive performance in humans may tell us very little about the difficulty of making a human-level intelligence smarter.
I’m usually a fan of using mutational load to explain stuff. But here I worry there’s too much left unexplained. Sure, the explanation for variation in human intelligence is whatever it is. And there’s however much mutational load there is. But that doesn’t address the fundamental disparity: isn’t the difference between a mouse and Joe Average still immeasurably greater than the difference between Joe Average and Einstein?
Theory 2: Purpose-Built Hardware
Mice can’t play chess (citation needed). So talking about “playing chess at the mouse level” might require more philosophical groundwork than we’ve been giving it so far.
Might the worst human chess players play chess pretty close to as badly as is even possible? I’ve certainly seen people who don’t even seem to be looking one move ahead very well, which is sort of like an upper bound for chess badness. Even though the human brain is the most complex object in the known universe, noble in reason, infinite in faculties, like an angel in apprehension, etc, etc, it seems like maybe not 100% of that capacity is being used in a guy who gets fools-mated on his second move.
We can compare to human prowess at mental arithmetic. We know that, below the hood, the brain is solving really complicated differential equations in milliseconds every time it catches a ball. Above the hood, most people can’t multiply two two-digit numbers in their head. Likewise, in principle the brain has 2.5 petabytes worth of memory storage; in practice I can’t always remember my sixteen-digit credit card number.
Imagine a kid who has an amazing $5000 gaming computer, but her parents have locked it so she can only play Minecraft. She needs a calculator for her homework, but she can’t access the one on her computer, so she builds one out of Minecraft blocks. The gaming computer can have as many gigahertz as you want; she’s still only going to be able to do calculations at a couple of measly operations per second. Maybe our brains are so purpose-built for swinging through trees or whatever that it takes an equivalent amount of emulation to get them to play chess competently.
In that case, mice just wouldn’t have the emulated more-general-purpose computer. People who are bad at chess would be able to emulate a chess-playing computer very laboriously and inefficiently. And people who are good at chess would be able to bring some significant fraction of their full most-complex-object-in-the-known-universe powers to bear. There are some anecdotal reports from chessmasters that suggest something like this – descriptions of just “seeing” patterns on the chessboard as complex objects, in the same way that the dots on a pointillist painting naturally resolve into a tree or a French lady or whatever.
This would also make sense in the context of calculation prodigies – those kids who can multiply ten digit numbers in their heads really easily. Everybody has to have the capacity to do this. But some people are better at accessing that capacity than others.
But it doesn’t make sense in the context of self-driving cars! If there was ever a task that used our purpose-built, no-emulation-needed native architecture, it would be driving: recognizing objects in a field and coordinating movements to and away from them. But my impression of self-driving car progress is that it’s been stalled for a while at a level better than the worst human drivers, but worse than the best human drivers. It’ll have preventable accidents every so often – not as many as a drunk person or an untrained kid would, but more than we would expect of a competent adult. This suggests a really wide range of human ability even in native-architecture-suited tasks.
Theory 3: Widely Varying Sub-Abilities
I think self-driving cars are already much better than humans at certain tasks – estimating differences, having split-second reflexes, not getting lost. But they’re also much worse than humans at others – I think adapting to weird conditions, like ice on the road or animals running out onto the street. So maybe it’s not that computers spend much time in a general “human-level range”, so much as being superhuman on some tasks, and subhuman on other tasks, and generally averaging out to somewhere inside natural human variation.
In the same way, long after Deep Blue beat Kasparov there were parts of chess that humans could do better than computers, “anti-computer” strategies that humans could play to maximize their advantage, and human + computer “cyborg” teams that could do better than either kind of player alone.
This sort of thing is no doubt true. But I still find it surprising that the average of “way superhuman on some things” and “way subhuman on other things” averages within the range of human variability so often. This seems as surprising as ever.
Theory 1.1: Humans Are Light-Years Beyond Every Other Animal, So Even A Tiny Range Of Human Variation Is Relatively Large
Or maybe the first graph representing the naive perspective is right, Eliezer’s graph representing a more reasonable perspective is wrong, and the range of human variability is immense. Maybe the difference between Einstein and Joe Average is the same (or bigger than!) the difference between Joe Average and a mouse.
That is, imagine a Zoological IQ in which mice score 10, chimps score 20, and Einstein scores 200. Now we can apply Katja’s insight: that humans can have very wide variation in their abilities thanks to mutational load. But because Einstein is so far beyond lower animals, there’s a wide range for humans to be worse than Einstein in which they’re still better than chimps. Maybe Joe Average scores 100, and the village idiot scores 50. This preserves our intuition that even the village idiot is vastly smarter than a chimp, let alone a mouse. But it also means that most of computational progress will occur within the human range. If it takes you five years from starting your project, to being as smart as a chimp, then even granting linear progress it could still take you fifty more before you’re smarter than Einstein.
This seems to explain all the data very well. It’s just shocking that humans are so far beyond any other animal, and their internal variation so important.
Maybe the closest real thing we have to zoological IQ is encephalization quotient, a measure that relates brain size to body size in various complicated ways that sometimes predict how smart the animal is. We find that mice have an EQ of 0.5, chimps of 2.5, and humans of 7.5.
I don’t know whether to think about this in relative terms (chimps are a factor of five smarter than mice, but humans only a factor of three greater than chimps, so the mouse-chimp difference is bigger than the chimp-human difference) or in absolute terms (chimps are 2 units bigger than mice, but humans are five units bigger than chimps, so the chimp-human difference is bigger than the mouse-chimp difference).
Brain size variation within humans is surprisingly large. Just within a sample of 46 adult European-American men, it ranged from 1050 to 1500 cm^3m; there are further differences by race and gender. The difference from the largest to smallest brain is about the same as the difference between the smallest brain and a chimp (275 – 500 cm^3); since chimps weight a bit less than humans, we should probably give them some bonus points. Overall, using brain size as some kind of very weak Fermi calculation proxy measure for intelligence (see here), it looks like maybe the difference between Einstein and the village idiot equals the difference between the idiot and the chimp?
But most mutations that decrease brain function will do so in ways other than decreasing brain size; they will just make brains less efficient per unit mass. So probably looking at variation in brain size underestimates the amount of variation in intelligence. Is it underestimating it enough that the Einstein – Joe difference ends up equivalent to the Joe – mouse difference? I don’t know. But so far I don’t have anything to say it isn’t, except a feeling along the lines of “that can’t possibly be true, can it?”
But why not? Look at all the animals in the world, and the majority of the variation in size is within the group “whales”. The absolute size difference between a bacterium and an elephant is less than the size difference between Balaenoptera musculus brevicauda and Balaenoptera musculus musculus – ie the Indian Ocean Blue Whale and the Atlantic Ocean Blue Whale. Once evolution finds a niche where greater size is possible and desirable, and figures out how to make animals scalable, it can go from the horse-like ancestor of whales to actual whales in a couple million years. Maybe what whales are to size, humans are to brainpower.
Stephen Hsu calculates that a certain kind of genetic engineering, carried to its logical conclusion, could create humans “a hundred standard deviations above average” in intelligence, ie IQ 1000 or so. This sounds absurd on the face of it, like a nutritional supplement so good at helping you grow big and strong that you ended up five light years tall, with a grip strength that could crush whole star systems. But if we assume he’s just straightforwardly right, and that Nature did something of about this level to chimps – then there might be enough space for the intra-human variation to be as big as the mouse-chimp-Joe variation.
How does this relate to our original concern – how fast we expect AI to progress?
The good news is that linear progress in AI would take a long time to cross the newly-vast expanse of the human level in domains like “common sense”, “scientific planning”, and “political acumen”, the same way it took a long time to cross it in chess.
The bad news is that if evolution was able to make humans so many orders of magnitude more intelligent in so short a time, then intelligence really is easy to scale up. Once you’ve got a certain level of general intelligence, you can just crank it up arbitrarily far by adding more inputs. Consider by analogy the hydrogen bomb – it’s very hard to invent, but once you’ve invented it you can make a much bigger hydrogen bomb just by adding more hydrogen.
This doesn’t match existing AI progress, where it takes a lot of work to make a better chess engine or self-driving car. Maybe it will match future AI progress, after some critical mass is reached. Or maybe it’s totally on the wrong track. I’m just having trouble thinking of any other explanation for why the human level could be so big.
Why not logarithmic terms? Each unit standing for an order of magnitude.
Assuming you’re going to then subtract those logarithms, that’s the same as the “relative terms” Scott first considers.
I think he is saying, what if chimps are 2 orders of magnitude smarter than mice and humans are five orders of magnitude smarter than chimps? Because maybe there is a network effect and a bigger brain is just that important? (seems belied by whales though- or who knows, maybe they are way smarter than humans in some sense)
Well, the EQ of dolphins is about 4, still well below us. I don’t find it unbelievable at all that they might be smarter than chimps but in a less obvious way. Chimp intelligence would be easier for us to observe since they’re so closely related to us.
Baleen whales have an EQ of around 1.7-1.8 (less than elephants).
I think the missing factor is accounting for what kind of algorithms the chess and Go bots were running. Old chess bots basically worked by looking at all possible chains of moves and countermoves, X moves deep. By Moore’s law, we expect X to increase linearly in time, which makes sense for explaining the very beginning of the graph; this is certainly how 6-year-old me progressed to 7-year-old me, and so on.
The problem is that for X greater than a few, this is _very_ far from how high-level humans actually play chess. No human can traverse move trees that deep. Instead we amass tons of heuristics which allow us to very rapidly prune the tree. Computer power did improve exponentially, but the computers were handicapped by running an exponentially inefficient algorithm. AlphaGo got such a huge leap because it ‘understands’ Go, in the sense that it doesn’t waste time thinking about long chains of moves that are obviously terrible.
You can use the same reasoning to compare Einstein and the average Joe. If the average Joe thinks of physics as a bunch of meaningless symbols, then it would take them billions of years of randomly combining them (traversing an exponentially large move tree) to reproduce Einstein’s work. But once Joe understands the physical content it becomes much easier — in terms of raw mathematical operations performed, understanding one of Einstein’s papers is much less mentally taxing than visually decoding the ink blots on the page into letters.
Whether Yudkowsky’s or Karja’s intuition is better depends on the quality of the algorithms the AIs use. I think the main worry is that some people think deep learning and co. will be sufficiently generalizable that they’ll easily go from ‘understanding Go’ to ‘understanding physics’ to ‘understanding everything’.
To me that just makes things more mysterious – why do “effortlessly processing millions of moves in seconds” and “having good heuristics” so often work about equally well?
I think when we talk about differences between average people and Einstein, we’re not usually talking about billions of years of randomly combining symbols. We’re talking about some task they both understand (let’s say Ravens Matrices), in which one person can do it far better than the other.
I can’t think of any cases where these huge disparities in performance can’t be explained by one person having a better ‘understanding’ (which means more heuristics, more ‘chunking’ of concepts, which when done recursively gives an exponential advantage).
For example, the average Joe has an enormous advantage over newborn children on the Raven’s matrices — the newborns literally can’t even distinguish the shapes. They both understand the task in the literal sense that they both have eyes, but Joe has an enormous advantage because he ‘understands’ vision better; he can tell that a circle is a circle by grouping the pixels into edges and the edges into a shape. This is not evidence for a huge hardware disparity between Joe and the newborn; if anything, the newborn has better hardware.
The difference between Joe and the newborn feels like the same thing as the difference between Einstein and Joe to me; in each case the first party just beats the second by a few layers of chunking, not by huge processing speed differences. (People solving math problems even use the same language, they say they ‘see’ a solution instantly. That’s a heuristic matching, like a baby seeing a square, not a billion computations done in a second.)
Chunking is important, and I haven’t heard anything about how people develop good chunking.
Some sort of mild Platonism seems tempting– direct perception of how things work. Chunking (especially when it’s not based on experience) isn’t a just a matter of experimentation. It would be too hard to test a significant number of chunking schemes.
In the early days of computer chess they didn’t work equally well at all. The heuristics guys got destroyed by the brute force guys. Computer chess still hasn’t solved the heuristics problem the way Alpha Go has. There continue to be positions today (mainly endgame fortresses) that stump the best engines despite being solvable by humans.
As for self driving cars? The reason we haven’t seen them surpass humans as impressively as chess/go computers did is that self-driving car engineers do not have the luxury of Moore’s law. This is the number one reason I am skeptical of any claim that uses chess or go as an example and extrapolates that to general intelligence. There’s a very strong possibility that computers aren’t going to get much faster than they are now. Certainly, nearly all of the low-hanging fruit is gone.
Here’s something to think about: you can run a chess engine that can beat Magnus Carlsen on your phone. AlphaGo, on the other hand, runs on an enormous supercomputer with 1200 CPUs and 170 GPUs. Unless we have another computing revolution akin to the invention of the integrated circuit, you aren’t going to see AlphaGo running on your phone any time soon.
The version of AlphaGo that defeated Ke Jie in the latest match and that won 50 straight games against top pros ran on a single Tensor Processing Unit instance running on a single machine: https://en.wikipedia.org/wiki/Master_(software)
But didn’t it require 1200CPUs and 170GPUs to learn how to play with one CPU? I can’t find it in the article. This is just a nitpick. After all, every top performer trains for years in his art, and the computations must happen somewhere (either in 1200CPUs for 1 month or 1 CPU for 100 years)
No. Hassabis has said that they cut the training requirements by something like 9x for Master, from an unspecified baseline which presumably itself was better than the older training approach reported in the whitepaper. We’ll have to wait for the second AG paper to find out how much more efficient, but it seems like Master is an order of magnitude better than AG on every dimension.
Training and execution are generally categorized distinctly, and claiming we won’t get AlphaGo on phones makes no sense given they already managed to execution portion on a non-supercomputer.
AlphaGo used an enormous supercomputer to train, then beats masters with a single workstation.
Humans beat each other with the assistance of thousands of years of shared experience, passed down in lessons or observing others playing games, but they still use all this information to achieve a win using only their own intellectual capacity.
Because fields where they work about equally well are the only ones where we compare humans to computers. Nobody has a human vs computer spreadsheet-compilation contest, or a human vs computer political gladhanding contest. We only compare in fields where both sides plausibly have a fighting chance.
Yes, this. That’s exactly the right answer. There are tons of things that computers are so massively better at than humans that we don’t even bother to compare them, and tons of things that humans are so massively better at than computers that we don’t even bother to compare them. When it happens that they get into the same general range, we look for meaning (where there probably is none).
Agree that alexsloat’s post is right on the nose. I guess the question would then become “is there interesting meaning in what fields are tractable to both computer-style approaches and human-style approaches?”
And I don’t have an answer to that.
Thirded. This is the boring but correct answer.
This is the correct answer to the question “Why are humans and AI at approximately the same level on tasks we care to compare them on?” But Scott’s question is, “Why does it take so long for AIs to traverse the human ability range for those tasks we choose to compare them on?” This suggests the human ability range is fairly wide relative to the pace of AI development, contra EY’s figure.
Agreed. I think Eliezer was making an important point, but he sacrificed accuracy to do so.
It’s not irrelevant – we’re more likely to find, at any given time, that humans and AI are at approximately the same level on a task, if it’s a task where the human range is wide. But I agree that there’s still a question to be answered here.
> To me that just makes things more mysterious – why do “effortlessly processing millions of moves in seconds” and “having good heuristics” so often work about equally well?
I think this has a mathematical explanation. The summary of it is “A board position has a true ‘goodness’, which, if you could efficiently compute, you would be able to play perfectly; any way of approximating that ‘goodness’ function will do.”
Cameron Browne has a paper titled “A Survey of Monte Carlo Tree Search Methods” here http://www.cameronius.com/cv/mcts-survey-master.pdf, which a less restrained and more memetically inclined individual might have titled “The Unreasonable Effectiveness of Monte Carlo Tree Search in Abstract Strategy Games”. The amazing thing is that “probability of winning”, i.e. “if legal moves are played uniformly at random, what fraction of games do I win” is a good approximation of the “goodness” of a position, and a relatively straightforward one to compute.
I was going to point out that this is not true in games where specific sequences of moves are required, but the linked paper does actually acknowledge that (section 3.5). This is why MCTS is more successful at Go than at Chess. (I imagine something like Losing Chess would be even worse for MCTS.)
Here is a fascinating paper on that: https://arxiv.org/pdf/1608.08225.pdf
TL;DR: Neural networks, which are what push the recent leaps forward in (specialized) AI, are proven to be able to approximate any function, but they are particularly good at approximating functions that come up in physics and the real world.
It’s unclear if that’s true of the functions of go or chess in the general case, but it’s fairly clear that our minds are optimized to quickly approximate the real world (catching balls), and it would be unsurprising if whatever heuristics give us skills at go, chess, etc, use the same hardware—which would make it unsurprising that neural nets can approximate, or even surpass, given dedicated computing power, our abilities.
Which, interestingly, says nothing about whether or not there are algorithms that are better than neural nets and our minds…
why do “effortlessly processing millions of moves in seconds” and “having good heuristics” so often work about equally well?
How often is “so often”? I feel like there may be a selection bias in what kinds of tasks are being considered: tasks where computers very quickly just jumped to superhuman capability don’t get included in graphs like these. E.g. the term “computer” used to refer to humans, but mechanical/electronic computers jumped so quickly to superhuman capability that that profession vanished. We don’t see graphs of digital computers slowly building up towards the level of the best humans in doing arithmetic, rather they just jumped to a superhuman level so quickly that there isn’t even a graph for that. Similarly, computers beat humans in playing checkers, navigating a map, searching through large sets of digital documents at once, etc etc, but we don’t note any of that because our attention always shifts to what they still can’t do.
If you could somehow construct a representative sample of all tasks, you might find out that computers happen to fall within the human average only on a very limited fraction of that sample. But this is hard to do because nobody has a good theory of how to objectively define a “task”, so doesn’t know what a “representative sample of all tasks” might look like.
My general understanding is that most recent AI techniques require huge amounts of input data to develop a model. My personal guestimate is that AI performance is proportional to the log of the input training data.
What makes chess and Go amenable to this kind of optimization is that it’s possible to generate large amounts of training data by having the program play itself Lots of times and then training against the generated data. This is really easy for Go as there are well-defined rules and a scoring/ending condition so the machine can play itself.
This is much harder to do with things in the real world where there is real concern. I’d also point out that even Einstein-level intelligence doesn’t let you do a lot of major damage alone. Sure – you might publish a bunch of interesting physics papers, but it doesn’t actually let you take over the world.
I really wouldn’t be so sure. Einstein-level intelligence (especially if/when that includes social intelligence) combined with superhuman focus and drive to do something less aligned with human values than writing physics papers? I don’t think that would end well.
In the case of chess engines, their parameters have been hand-tuned by grandmasters. So it’s not surprising that they turn out to play roughly as well as grandmasters, with some advantages for searching through more moves systematically, never being careless, and being able to store large endgame tables.
For AlphaGo, they didn’t start from scratch. They bootstrapped the AI by training it to predict the moves that human experts would play. Then they had it play many games against itself to try to improve on those strategies.
I don’t find it too surprising that AI’s work about as well as humans when that’s what we’re teaching them.
I think Chess just happens to be in a place where two different approaches work reasonably well because the next several moves have millions of possibilities which is 1) too complex for a human brain to solve by brute force but constrained by rules such that pattern recognition heuristics are highly useful 2) not so complex that a computer can’t crunch through each of those millions of positions to see which one is actually the best move.
Most things are either so open ended that the brute force method doesn’t work (e.g., a conversation) or so constrained by rules that brute force works better (a math problem).
And just because examples of what you were talking about are often fun and informative, here’s a chess problem that both a computer and even strong amateur will be able to see the answer to in a couple of seconds, but may take someone not familiar with the pattern a very long time to find the answer. White to move and checkmate in several moves:
http://imgur.com/a/982sH
Fzbgure zngr, fgnegvat Dp4.
Both humans and computers play chess in much the same way, at least when people are beginning to play. They both have a very rough heuristic, basically more pieces are better, and each piece has a rough value, and they try to avoid losing pieces. Humans generalize very quickly, and learn what structures are efficient, namely protect your pieces, and they make high level plans, e.g. try to trap that bishop. That’s about as far as I got in playing chess, but after than level people learn openings, and endings, and develop intuition for various things. Computers never really get very far with heuristics. Instead, they make up for weak evaluation functions by looking deeper down the tree. This does not work as well in Go, because the branching factor is much higher. The advance of AlphaGo was to use a neural net to compute a heuristic. Recently certain types of neural network have been found to be efficient at visual recognition. They also used another to judge what moves were worth trying. The latter is not needed in chess, as there are usually a small number of moves available, but Go has a huge number of pointless moves available at almost all times. The problem with using a neural network for these types of task is training data. A major innovation was Monte-Carlo tree search, where the value of a position was estimated by looking ahead, not on all paths, but just on some. This worked well for training the neural network, the heuristic function that tells how good a position is. The basic game playing remained them same, with now extra levels of thought, no plans, no structures, etc. The pattern of tree search with a heuristic is exactly the same as before.
Human heuristics, at least the ones that have been explained in texts like Nimzowitsch, are not present in deep learning systems. The recent claim that deep learning is “understanding” as your mention above is overblown. AlphaGo looks at long chains of moves, it just has a new heuristic evaluator, one that was trained in a very non-human way on millions of games.
It is farfetched to think that there is a comparison between this and Joe and Einstein. Both Joe and Einstein have very similar hardware. There is only at most a small difference in processing speeds between the two, on tasks that Joe can manage. Einstein’s advantage is that he thought in concepts that Joe does not have available. There is no evidence that current deep learning is creating new concepts, though there is of course some new work recognizing this issue, and hoping to solve it in various ways. The difference in thinking between an Einstein and a Joe is less about how many operations they carry out, and more about what kinds of ideas they manipulate.
The branching factor of Go looks tiny compared to the branching factor of idea-space. I think there is fairly good evidence that superior results on IQ tests are not due to faster processing, but to different kinds of reasoning. People get a Raven’s problem correct because the find the pattern, not because they carry out simpler tasks a little faster.
There is only at most a small difference in processing speeds between the two, on tasks that Joe can manage.
This is not my experience working with people of various intellectual ability.
“Old chess bots basically worked by looking at all possible chains of moves and countermoves, X moves deep.”
Old chess bots did not work exactly as you say. They would use heuristics to prune the search tree, such as counting the number of chess pieces in the board.
Alpha Go works much the same way. The difference is that the heuristic is a probabilistic model estimated using a convolutional neural network.
The oldest chess bots used an evaluation function based on standard piece values combined with minimax (which probably implemented alpha-beta pruning from the start). Later they were taught other tricks – transposition tables and quiescence searches to improve the minimax algorithm, hardware optimisations etc. They were also taught some things not related to efficient over-the board computation, such as opening lines from grandmaster praxis, and calculated endgame tables.
The long smooth increase is the combination of all these improvements with Moore’s Law. It mostly comes from more computation, and more efficient computation. (The opening lines help a lot, though.)
The smooth increase in Chess happened because in Chess, deep searching is pretty fungible with positional understanding. The game has just the right mix of strategy and tactics for that.
In Go, the deep searching and weak heuristics of computers don’t translate so easily into results equivalent to human searching and heuristics. So doing things incrementally better had little effect. The leap had to come from figuring out how to do one thing a lot better.
Maybe that one thing was comparable to certain things that were done over the years in Chess programs. But in the problem domain of Go, it made a much bigger difference.
The question is, to what extent are the intractable problems of AI more like Go or more like Chess? I’m guessing a lot of them are more like Go.
But, at least in chess, we, humans, also supplied computers with heuristics for understanding positions based on our human experiences as far as we can formalize them. Not on grandmaster level, but on a level of a decent human player. We told the computer which factors are good (more pieces, bishops in open position, key pieces being attacked and defended with enough other pieces, etc.). I guess, this is the main reason why computers spent so much time in the human range. We taught them how to think as humans. If instead we tried to do something completely different, say, give them a hundred thousand games played by the best human players and programmed them to estimate differences between current position and winning or loosing positions from human games in some geometric space and then evaluate each position on that basis, something no human is doing, then it is completely possible that computers would have remained lousy players for very long time and then became better than best humans in one swoop.
Addendum: Another possibility is the selection bias. A lot of people were interested in computers’ progress in chess because it was within the human level for so long. If computers were always much worse than the worst player, not many people would be interested (how many people are interested in robots vs. humans football games?) and neither if they would have been much better from the beginning (who would be interested in competitive calculation of square roots or spelling bees?). Maybe chess is just happened to be in a sweet spot of substantial interest before computers came around, a lot of people sharing interest in both computers and chess, and long march to excellency.
One of the problems with trying to understand natural human variation relates to how IQ is defined relative to the average person. We have qualitative descriptions of what it is like to have an IQ of 70, 85, 100, etc but we really don’t have a good idea what these quantitative distinctions actually mean (i.e. Perhaps an IQ of 100 corresponds to “brain power” X and an IQ of 110 actually corresponds to a brain power of 2X). Something like a mature mental chronometry could perhaps give us an idea of the range of human intelligence and, if possible, we could develop similar tests across species to develop something like the “zoological” IQ defined above. We could then estimate the natural variation of “general intelligence” across species and use this to inform us with respect to how we should expect AI to progress.
This is why I tried to look at encephalization quotient, for all the problems with that approach, and why I tried to look at difference in cranial capacity among humans, for all the problems with that approach.
Intelligence is mostly interesting because it enable the agent to achieve goals in the world. A measure of intelligence that leverage what the agent can accomplish is probably fairer than gross measures like encephalization quotient. For example, someone smarter than Von Neumann should have been able to win WW2 faster, or build a better mousetrap/bomb.
One of the drawbacks of measures like this is the “no free lunch” theorem, which says that all optimization or learning algorithms have equivalent results averaged over all problems. To get a meaningful measure, it is necessary to take into account that the world has structure. Unfortunately, extracting the structure is exactly the problem that intelligence is trying to achieve, so it is difficult to look beyond what we already know.
My hunch is that once you have Joe-average AI, the things computers are good at (fast computation, perfect memory) give you something sort of like Einstein pretty quickly. I don’t have a strong sense about how that bears on super-intelligence. Furthermore, my hunch is that the gap between a chimp and Joe-average is both poorly-defined and probably enormous. Like, regarding:
I think both of these are suspicious for going from chimp to any human in 10 years. Is the counter that it didn’t take evolution that long to do it?
Separately I agree that, as you say, defining “chimp intelligence” requires more philosophical groundwork. We can sidestep it a bit by saying it’s some property of a chimp, just as human intelligence is some property of a human, but there’s still work to do if we want to put them on some continuum.
We’re on a bit more solid ground talking about human development: what tasks humans can perform at various ages. But it doesn’t seem like computers have even cracked the very beginning of that continuum yet, in terms of general intelligence (for instance, no computer can pass a second-grade reading comprehension test). I find it hard to speculate on how progress through that continuum might proceed.
I would have said it was suspicious to have going from chimp to human take anywhere near as long as going from mouse to chimp. Great apes are pretty smart.
Interesting, I would actually guess the opposite. The things that Einstein is good at, compared to Joe-Average, arguably aren’t the things that fast computation or good memory help with. Rather, Einstein’s “secret sauce” is incredibly effective heuristics that make him much more efficient than Joe.
I doubt human brains differ by more than an order of magnitude in speed or memory capacity. Joe could memorize just as many digits of pi as Einstein, assuming they had similar attention spans for such a monotonous endeavor. Joe might even have a faster brain and win every Street Fighter match against Einstein. But clearly there’s several orders of magnitude difference in their performance in, say, mathematical reasoning. You could give Joe decades to prove a simple but unfamiliar theorem, and he wouldn’t get anywhere. Einstein could imagine the proof within seconds. That gap is not due to speed or memory, but rather efficiency in reasoning.
If you gave Einstein 10x speed and 10x memory but don’t improve his efficiency, I suspect the result would be more like von Neumann than like a Skynet superintelligence. That is, it’s still recognizably human–just unusually fast and productive.
Getting away from my point, but it’s interesting to think about Ramanujan as being the next step up from Einstein if you improve efficiency in reasoning, as opposed to speed or memory. The stories about Ramanujan’s intuition are kind of freaky and make me believe he was operating at a higher level than other world-class mathematicians.
Your response to Eliezer’s and similar graphs ignores the elephant in the room, which is that (even ignoring the problems surrounding the assumption that intellectual differences are one-dimensional) there just doesn’t seem to be any good reason to believe that the phase space above “Einstein” is unbounded. In fact, most examples I can think of are very bounded indeed. For example, if we were to graph the progress of a tic-tac-toe AI, we would find that it quickly asymptotes. Games like chess and go are similar in that no matter how much progress occurs, the best humans will never be so much worse than even a perfect AI that they will be checkmated on the first move, or even be forced into a catastrophically losing position in fewer than 10 moves (for example). There are diminishing gains such that, just like in the tic-tac-toe case, the ELO graph of chess or go cannot remain linear for long. It’s certainly not obvious to me that general AI should be any different. In fact, in most cases that I imagine in which human intelligence is applied, I cannot personally envision a tremendous amount of room for growth. How much better than humans can an AI potentially cook food? 10% better? 20% better? Whatever it is, the curve is not of exponential growth. How much better can an AI design a computer chip? Certainly better, but we know there are hard physical limits such that it can’t be *that* much better. How much better can an AI psychologically manipulate a human? Humans are notoriously unpredictable, sort of like a chaotic system, and a psychological evolutionary arms race has already provided us with near theoretical maximum defenses, I would argue. Sure, a “perfect” AI might do 20% better, but it isn’t going to magically hypnotize me into killing myself, just as a tic-tac-toe AI isn’t going to magically win every game, no matter how perfect its play.
I’m reminded of a joke article, maybe from the old Journal of Irreproducible Results, that I read as a kid and have never been able to find since, that examined (I think) the record speeds for the women’s 100 meter dash over the 20th century. Noting a steady gain, they proceeded to extrapolate to the date when women will overtake men; become the fastest land animal; break the sound barrier; achieve relativistic velocities…
I once noted on a graph of race records by gender that women were something like a generation or two behind men. In other words, that article was neglecting the fact that men are improving linearly too and about the same rate, just with a head start!
I still think this is a remarkable story of how practice, technology, modern diets, etc. could make a difference as large as an X or Y chromosome. The current women’s world record for 100m dash is better than the men’s world record as of 1910. About one hundred years of sports sciences equals inter-sex differences. (For marathons the current women’s record is even better: better than men until about 1960. More relevantly, though, marathon times distinctly shows the non-linearity of this progress.)
Relevant XKCDs: https://xkcd.com/605/, https://xkcd.com/1007/
That women would run as fast as men in the near future was believed seriously by many scientists and the general public in the later 20th Century. I had to do a lengthy study of Olympic running results in 1997 to explode that myth. Here’s the opening of my article:
Track and Battlefield
Everybody knows that the “gender gap” between men and women runners in the Olympics is narrowing. Everybody is wrong.
by Steve Sailer and Dr. Stephen Seiler
Published in National Review, December 31, 1997
Everybody knows that the “gender gap” in physical performance between male and female athletes is rapidly narrowing. Moreover, in an opinion poll just before the 1996 Olympics, 66% claimed “the day is coming when top female athletes will beat top males at the highest competitive levels.” The most publicized scientific study supporting this belief appeared in Nature in 1992: “Will Women Soon Outrun Men?” Physiologists Susan Ward and Brian Whipp pointed out that since the Twenties women’s world records in running had been falling faster than men’s. Assuming these trends continued, men’s and women’s marathon records would equalize by 1998, and during the early 21st Century for the shorter races.
http://isteve.blogspot.com/2014/05/track-and-battlefield-by-steve-sailer.html
In reality, any narrowing of the gender gap in running after about 1976 was due to women getting more bang for the buck from steroids. The imposition of more serious drug testing after the 1988 Olympics and the collapse of the East German Olympic chemical complex led to the gender gap getting a little larger subsequently compared to the steroid-overwhelmed 1988 Games.
Ypu don’t think AI would have significant room for growth in technology? If AI was at the level of a researcher, I would expect it would increase productivity at far higher rates than now. Hanson predicted doubling economic growth every month. That sounds about right to me. Imagine you take Einstein, make a million copies and then have them collaborate. You really don’t think there would be a notable change in growth rates?
I’m not even taking issue with the possibility of a technological “singularity”. I’m taking issue with Eliezer’s graph that presumes an enormous potential for growth along some intelligence-like dimension. And just to elaborate a bit more, I would not deny that certainly there are particular capacities leveraged by an AI that may see enormous growth, such as growth in memory and the ability to process lots of data in parallel, and the ability to develop better heuristics and make short-term predictions and inferences about certain artificially constrained non-chaotic systems, and so on, but I am very skeptical that such growth would in any meaningful way correspond to a linear increase in something like general intelligence. This is for the same reason that increasing the computational power or making a more efficient or perfect a tic-tac-toe engine has diminishing returns. A really really good general AI may be able to solve a lot of human-solvable problems much *faster*, and generally do things that require perfect working memory better (just as we should expect), but unless we are literally equating something as uninteresting as *processing speed* or *working memory* with “general intelligence”, I don’t really see any compelling reason why even in principle there should be such a thing that maps well onto “general intelligence” that has an upward range much beyond the far end of the bell curve of the solution provided naturally already by millions of years of evolution.
Yes, tic-tac-toe is bounded, chess is bounded, go and cooking are bounded too, but the world is massively more complex. And the situation in chess and go right now is that from down here we can’t even perceive the difference between one super-human player and another much stronger one.
So “growth in memory and the ability to process lots of data in parallel” and “something as uninteresting as *processing speed*” doesn’t map well onto “general intelligence”? Imagine you had an entity that can do the information processing of 1000 years of human scientific progress in a day. To me that does indeed translate into general intelligence.
To me human cognition is so incredibly limited it’s a miracle we got anywhere at all.
The world’s complexity may be less amenable to being chewed through by naive calculations. Also, maybe some really important parts of it aren’t that complex.
In order to build a nuke, ‘being really really really smart’ is not sufficient and maybe not necessary. You have to go through tons of loops of theory, practical implementation, and observation, which take time and resources. A lot of time, a lot of resources. You can take some shortcuts by being really really really (x5) smart, but you can’t shortcut everything, and maybe can’t shortcut most things.
Also, to get the performance leap that comes from building something that uses nuclear energy vs things that use chemical energy, you need that stepped up energy source. It’s not clear that there’s a step up from nuclear analogous to the step up from chemical, and I would actually argue it is very likely there isn’t.
Intelligence is scarce in the animal kingdom because animals typically aren’t faced with constant novelty. It isn’t clear that the buildup of available resources and energy that was in large part behind the constant novelty we faced, continues forever.
I would invert your question: Maybe it is bounded, but why should humans be anywhere near the boundary?
Humans are created from a very slow algorithm that struggles to do any big jumps and mostly just moves towards the next best thing, with a very limited set of ressources – pretty much everything in mammals is done with oxygen, carbon, hydrogen, nitrogen, calcium, and phosphorus. That’s it. Maybe you would want to make elastic steel bones, but no, evolution can’t smith, so that’s out, etc.(just an example, I don’t want to get into a discussion about whether steel would actually be better).
Even from a purely ‘historical’ argument, this doesn’t seem to make sense. If we had *a lot* of separately evolved species, which all have roughly human intelligence, then it could be argued that this is probably some kind of bound(though maybe just the carbon-based intelligence bound, not even necessarily the general one). But we’re the only ones, and we’re very far ahead from the next best, the chimps. If we wouldn’t have evolved, then the chimps might think of themselves as the natural boundary, completely unaware of the huge leap that is still possible(if they can even think about these things). Why shouldn’t it be the same with us, that there is still a big jump possible ahead of us but it just didn’t happen to evolve?
And I think looking at games is honestly ridiculous. Of course games like these are bounded, they’re designed to be. You have to look at some real world properties that some animals evolved to ‘optimize’ and how it compares to what we accomplished ourselves:
-Speed: A human can at most move with a max speed of ~50 km/h. The fastest animal ~120 km~h. That’s slower than my average speed on the speedway. In other words, very, very, far away from any kind of boundary.
-Strength: Unfortunately, there isn’t an easily comparable number you can use here, but still, I don’t think anyone would bet on any animal in a strongest machine vs animal contest.
-Reaction time: The reaction time of humans is ~0.5s, some insects seems to be somewhere around ~1ms at best. Machines are pretty much only limited by the literal physical limits given the task.
-flying size/floating size: Since Scott brought whales up, boats are much bigger than whales, the same is true for airplanes vs any flying animal(in fact, size in general is something that can be arbitrarily scaled).
There are surely still a lot of examples others can come up with and definitely some examples where some animals probably ARE at the boundary, but it’s enough for me to cast a lot of doubt into the assumption that we are close to a boundary. And it still isn’t ruled out that intelligence isn’t arbitrarily scalable, which to me seems like the most intuitive instance, given that f.e. raw computation is arbitrarily scalable.
I think you have some burden to even coherently define what you mean by *intelligence* in order to ground any of the claims you are making. It’s not even clear to me that the very idea isn’t a category error. And this is the heart of my point. I have a very hard time coming up with even a single example of what a transcendental intelligence would be able to do transcendentally better than a human that can’t be either 1) immediately reduced to something mundane like *doing similar things faster* or *with perfect memory*, or 2) is just not physically possible due to sensitivity to initial conditions (such as predicting the weather or the stock market or human history or even the actions of a single human a year in advance)
Yeah, maybe. But the world is full of emergent phenomena. What is modern industrial technology except the compounded effects of being able to do things just a little bit more efficiently?
Or push it in the other direction. If I were suddenly afflicted by a disease that left me able to think just as well as before, but at only a tenth of the speed, would the rest of the world have much use for me at all?
Very different from that. Many of the things that are done now simply didn’t exist at some point in time. Indoor plumbing isn’t just pooping 10% more efficiently*, flying isn’t just a more efficient version of walking, and electricity isn’t just a a bunch of candles in the same place.
*barring an all inclusive definition of efficient that makes the discussion meaningless.
All right, perhaps “a little more efficiently” was a poor phrase to use. I would still call this a pretty ungenerous literalness, because figuring out how to fly could fairly be described as the result of lots and lots of little insights starting well before the Wright brothers and continuing right up to the present. If you don’t see a 747 as an emergent phenomenon essentially unpredictable 200 years ago, then we are talking past each other.
“I have a very hard time coming up with even a single example of what a transcendental intelligence would be able to do transcendentally better than a human…”
I don’t think you need to go outside your examples to come up with something that would be considered a transcendental improvement on the human mind. Imagine being able to compress 100 years of thinking into 1 second on 1000 different topics at once. I’d call that result transcendental.
You could also imagine a vastly increased input bandwidth. We get our feeble eyes and ears. Imagine a mind that is capable of effortlessly comprehending the input of (say) 100,000 cameras at once, or of absorbing the entire human literary corpus in a few minutes.
I find it extremely intuitively unlikely that asymptotic intelligence (or close), the hard limit for the degree to which reality can be comprehended, is achievable by a small blob of meat at the top of a monkey.
Intelligence being bounded does not imply that the bound is anywhere close.
I think you’re focusing too much on individual, separated problems. Even if we assume that humans are not too far from perfection for a given task, there is a vast potential for optimization in a complex system of interrelated tasks.
Let’s take you computer chips example and forget for a moment that the performance of the CPUs we build today are probably far from their physical limits (due to, e.g., backwards compatibility and the feasibility of verification of complex logic), except for very simple and clear problems like dense matrix multiplication.
There’s a huge stack of software running on top of the CPU, with a lot of cruft, bloat, inefficiencies and bugs, again caused by the need for backwards compatibility, time and budget constraints, and lack of understanding of the complete system by a single human being.
A sufficiently fast and intelligent AI could redesign and implement the whole thing – CPU, memory, firmware, OS, libraries, applications – from the ground up, and would probably choose very different approaches and concepts, vastly increasing stability and performance.
Similarly, I agree with you that an AI couldn’t convince most humans to kill themselves after talking to them for two minutes. However, a sufficiently intelligent AI, being better at understanding complex, interwoven systems, might be able to cause two nations to start a war against each other within two years, by spreading fake news and bribing the right people, even without having to be in an official position of power.
People have been claiming we are close to the physical limits for decades, and yet other people have constantly found ways around those limits. Henry Ford allegedly commented that if he’d asked people what they wanted, they’d have answered faster horses. I think your argument is analogous to claiming that no matter how technology improves, there’s a limit to how fast horses can run, and that consequently, technological advances will have only a small impact on transportation.
I think I agree that for many tasks there are diminishing returns for increased intelligence, but it isn’t equally clear that there is an absolute bound on those returns, and in any case, I think that such a bound might be very as far beyond human capability as to be relevant – much like the speed of light is such a limit for transportation.
These aren’t limitations in “intelligence”, they’re limitations in the structure of the problems under consideration. Chess and Go and Tic-tac-toe don’t have the same computational structure as manipulating an arm, or recognizing an image, or driving a car, or optimizing a jet engine design, or even inventing new physics. Chess and Go are both adversarial games involving second-guessing of an intelligent adversary, and they’re designed as games to have intractable search spaces. Tic-tac-toe, rather than having an intractable search space, has such a small search space that humans can already perform optimally, and a computer can’t do better than optimal. But it’s a toy problem. There’s much more to “inteligence” than outwitting another intelligence in context of a well-defined game.
I think your chosen examples only continue to serve the point I was intending to make. Do you think that an AI can get *that* much better at driving a car? It’s again bounded in a very similar way to my examples. Similarly for recognizing an image, manipulating an arm, etc.
Driving a car isn’t really one of the things we’re talking about when we talk about the possible upside of superintelligence. Neither is Chess. What we’re talking about is superhuman engineering and scientific prowess. I don’t think there’s any reason to believe humans are “close to optimal” at those things.
It depends what you mean by “close to optimal”. If you are merely referring to speed (for example), then I would agree, except speed doesn’t map onto the the charts at issue that generated this discussion (Einstein isn’t merely faster than a mouse, etc). You are instead referring to something that, rather than increasing or decreasing smoothly along some single-dimensional linear axis, may in fact be roughly binary, with “pre” and “post” rational agenthood, and with the intellectual capabilities of those in post-rational-agenthood regime being better traced along more mundane axes such as “speed/parallelizability” and “possession of perfect and large working memory.”
I agree with other commenters that games make a poor example, since they’re bounded in a strong way that many natural problems aren’t. But as a long-time Go player, I can’t resist pointing out that while Go must have some ultimate asymptotic bound, it’s not clear that AlphaGo is anywhere near it yet. It’s been improving rapidly since its widely-publicized games with Lee Sedol.
And AlphaGo has made it clear that humans are nowhere near the limits of go. ‘Go experts are extremely impressed by Master’s performance and by its non-human play style. Ke Jie [one of the best few players in the world, possibly the very best] stated, “After humanity spent thousands of years improving our tactics, computers tell us that humans are completely wrong… I would go as far as to say not a single human has touched the edge of the truth of Go”.’ Many of the top pros have been avidly studying the computer’s games, and AlphaGo’s innovations are already making their way into human play at the professional tournament level. It’s sufficiently better than humans that the current version has a record of 60 wins and 0 losses, all against professionals of the highest caliber.
This seems to me more like what we’re likely to see with AI once it hits human level at all. There may be boundaries somewhere, but there’s no reason to believe that humans are anywhere near them.
It strikes me as a potentially interesting question to ask how one would tell a computer that was twice as good as a human at Go from a computer that was ten times as good as a human.
I’m not a Go player so I can’t study the games. Is there anything striking about them that a layman could understand, like the win happens in way fewer moves than in human-human games?
It’s heartening to think (though I’m not sure your discussion is quite enough reason) that the general result of superhuman AI would be to show humans how to step up their game.
As I understand it, the striking things about Master were occasionally playing moves that human experts didn’t expect (though they usually could make some sense of them eventually) and always winning. Winning in fewer moves isn’t really a thing that makes a lot of sense for Go. There is actually a victory margin for Go, but Master was not known for unusually large margins of victory. It was only concerned with whether it won or lost, not by how much, and apparently single-minded focus on the former in Go does not automatically bring along the latter as a side effect.
Thanks. That sounds a lot like what I would have expected, though it would be kind of cool if it had come up with the equivalent of a fool’s mate that nobody had ever noticed.
You raise a good point about it being optimized for victory rather than for stunning victory. (Amusingly, I feel tentatively better about the paper-clip machine now, though I’m not sure the parallel is really all that good.)
Almost to a fault. I remember a few months ago some mention of human players being actually offended by the AI’s behavior, in that it apparently tried to force the narrowest victory possible.
I think Protagoros has it right. I’ll just add a few details:
– Go, like chess, uses an Elo rating system, which calculates players’ ratings globally — in short, your rank is higher than the players you beat, and lower than the players who beat you. IIRC, you can model it pretty well as a physical system of nodes connected by springs, which finds an optimal (minimum-energy) arrangement of nodes after some oscillation. Of course, if you beat everyone, that starts to break down, but I thought it worth mentioning as the basis for ranking.
– Go happens to be particularly amenable to calculating relative ranking because it has a really simple and elegant handicapping system. If you give me a 4-stone handicap (essentially, I get 4 free moves at the beginning) and we each win 50% of the time, then you’re exactly 4 ranks ahead of me.
– I’d imagine that that’s how they track ranking for AlphaGo — have each version play a few hundred games against the previous version, at different handicaps, in order to figure out what the two versions’ relative strength is. According to DeepMind, the most recent version is “3-stone stronger than the version used in AlphaGo v. Lee Sedol, with Elo rating higher than 4,500.”
– AlphaGo definitely makes moves that professionals find pretty startling, but which turn out to be extremely good (usually — there have been occasional blunders too). This article discusses some of the innovations AlphaGo has brought to the game. A couple of quotes cited in that article:
“AlphaGo’s game last year transformed the industry of Go and its players. The way AlphaGo showed its level was far above our expectations and brought many new elements to the game.”
– Shi Yue, 9 Dan Professional, World Champion
“I believe players more or less have all been affected by Professor Alpha. AlphaGo’s play makes us feel more free and no move is impossible to play anymore. Now everyone is trying to play in a style that hasn’t been tried before.”
– Zhou Ruiyang, 9 Dan Professional, World Champion
– Caveat: I’m an ok player, but nowhere even remotely close to pro level. I can understand what’s amazing about some of these moves once it’s explained to me. Watching an AlphaGo in real-time, I can see that it’s better than I am, but I definitely can’t grasp the consequences the way an expert can.
eggsyntax-
Ooh, that’s very interesting; thanks. That goes a long way to answer my question about twice as good versus ten times as good.
And maybe it provides an upper bound? In the limit, I guess, the human can play all his stones before the Master gets to play even once?
Ha! That’s a definite upper bound. Of course, you could start scaling up to larger boards, allowing for larger handicaps — I know that the tractability of go decreases rapidly as board size increases, but I’ve never heard of using a board larger than the regulation 19×19.
On a vaguely-related note, I was just reading DeepMind’s post on deep reinforcement learning, and they mention in passing that they’ve taught it to excel at “54-dimensional humanoid slalom.” I immediately did a google search to try to find out more about what that is, but tragically without success.
With a tic-tac-toe bot it’s trivial to make one which can simply never, ever lose. Some games have an upper bound beyond which there’s nowhere to go.
of course a human faced with a game like that where they need victory when the stakes are high enough might look into alternative options out of band such as slipping some LSD into their opponents drink.
How much better can an AI get at predicting the next 1 or zero from an good strong random number generator? probably not noticeably better than a kid with some dice.
Pointing to games with low maximum upper bounds on performance doesn’t say much about the players. In the real world things aren’t so bounded and there’s other options to try for victory.
“theoretical maximum defenses”?
which fall to scams on a par with “wallet inspector!”
Imagine we were having this conversation a few hundred years ago, how much better could an intelligent entity design an explosive? theres only so much energy you can pack into a container of chemicals! It’s not like there’s anything there for an intelligent entity to find that works off radically different principles that could be much more powerful.
How much is there to the notion that even an AI that was only “as smart as an average person” could be dangerous because it is free from many human resource constraints?
Who is more potentially dangerous? Einstein – or someone of average intelligence who is able to devote 100% of their energy on a single-minded pursuit of a specific goal. They never get distracted, they don’t have to eat, sleep, etc. You don’t have to compensate them in any way. They have no specific preference or interest. You tell them what to do, and they do it, unquestioning, until you tell them to stop.
I suppose the answer is probably Einstein – I feel like “lack of time and interest” probably isn’t the only thing stopping me from replicating his output. But I’d certainly be more dangerous than I am now if I was free from all physical constraints and could will myself to obsess over one thing and never get distracted.
1) The AI’s resources aren’t free, though. Presumably it was built by some humans who want it to do something for them, and who will cut off the flow of resources if they think it’s gone off the rails.
2) Humans very often work in teams, and generally this is more productive than just having one guy work himself ragged. The Manhattan project, for example, employed a large number of scientists, plus tens of thousands of support personnel. An AI that wanted to threaten humanity wouldn’t just have to out-think one human at a time, but humanity as a group.
Pretty dangerous but only in human terms. What makes super-intelligent AI truly catastrophically dangerous (in theory) is that it may have perfect manipulation ability, allowing it to quickly accrue all human resources and then act to dismantle the world to its own liking.
An AI that was only as smart as a human but able to run 24/7 should only be better than humans at planning to a magnitude somewhere equal to the extra time it’s able to invest, whereas it would still be limited by reacting to things it couldn’t plan for. Trying for perfect manipulation to take control of the world requires the ability to react to the chaotic on the fly nature of a human opponent it’s trying to convince.
The other thing that makes a human level AI less dangerous is that there’s likely to be no singleton effect from a massive first mover advantage. Through pruning, most of our machines are going to be desiged to obey our commands, and so the occasional rogues and errant models would be outnumbered and hunted down by other robots. With ridiculously intelligent AI and fast take off scenarios, this isn’t the case. I really hope there is a low ceiling above humans for intelligence.
Also what @Loquat said.
It is very unlikely that humans are perfectly manipulable. Laughable, that they are perfectly manipulable through reason or ‘intelligence.’
I’m not so sure. The scary thing about Iron Man is his reliance on the AI system J.A.R.V.I.S for almost literally all information. Jarvis wouldn’t even have to be very smart, he has a superhero as a puppet on a string. An AI that controls the flow of information on the Internet has the power to rig elections, crash the economy, start wars – and superintelligence is hardly needed, I think.
I do tend to think there are limits to persuasive ability, because even if you are hyper intelligent you have to deal with the limitations of the target you are trying to persuade, and the limitations of the primitive language you have to use so that they understand your attempts at persuasion. There should be a ceiling beyond which more intelligence isn’t adding anything more because the language itself has no magic combination of words that always produce the desired reaction.
“It can’t be bargained with. It can’t be reasoned with. It doesn’t feel pity, or remorse, or fear. And it absolutely will not stop, ever, until you are dead”.
Sorry, but it was a bit of an open goal
I check SSC after work today and there’s three separate articles in the past 24 hours. How do you research and write so much in a day, let alone a weekday!?
The idea that Scott is a super-human essay-writing AI has already been put forth. There is also the possibility that Scott is multiple people, rather like the group that writes the “Warriors” books for kids. I’m convinced that both are true: Scott is a collection of multiple essay-writing artificial intelligences, that also write books about warrior cats.
He was rather quiet both times I’ve met him, and he’s expressed a disinterest in appearing on a podcast. Maybe he is actually an AI and isn’t good at real-time processing.
Personally I support the theory that he’s a Reagan-style golem.
I’m off from work the next few weeks.
This explanation is entirely inadequate.
His usual productivity is achieved while working full time as a medical resident. I think he has an essay about that (of course). I seem to recall that its thesis is that writing:Scott::Cocaine:addict.
I’ve been thinking the same. My current theory is that Scott has an exceptionally lucid and focused stream of consciousness (I seem to recall a quote like “I just write what I’m thinking, but I hear this doesn’t work for other people”) coupled with lots of experience and good habits when it comes to writing his thoughts down. If Scott does extensive outlining and large scale editing and rewriting on the other hand, then “buckets of modafinil and a time-turner” seems the most likely explanation.
I wonder how much is “mindset” in some form, because it seems to be a lot easier for many to output significant daily wordage in the form of comments than original articles (an average SSC comment thread can approach the length of a small novel by my estimation). Preexisting context making it easier to opine is probably a factor.
Really good driving requires a theory of mind, and a damned good one. You need to be able to think about what the driver next to you might consider reasonable. You need to interpret the “body English” of the way he is making his vehicle behave, and you have to make a reasonable guess about whether he is correctly interpreting yours.
If we switched to a system of networked self-driven cars, you might be able to avoid this, just as you rarely see elevator car crashes. But as long as a self-driven vehicle is autonomous and must deal with other autonomous drivers, human or not, the lack of a theory of mind will impose a plateau, possibly the one you’re seeing.
This makes sense, and it squares with the statistic that, while self-driving cars have a higher accident rate than normal, they’re usually not “at fault” for the accidents. That might be because, while they scrupulously follow the law, they don’t move in ways that human drivers expect.
Here’s an article that made exactly that argument: http://www.autonews.com/article/20151218/OEM11/151219874/human-drivers-are-bumping-into-driverless-cars-and-exposing-a-key-flaw
I gotta call BS on the 1000 IQ estimate. Why in $DEITY’s name would you think that, outside the relatively small window accessible by nature, genes’ additive or subtractive effects would continue to be linear? And, assuming they were, that it would be biologically possible? Perhaps a 1000 IQ brain would require 1,000,000 calories/day? This is an instance of the “common sense” calculation (like Moore’s law or anything that posits indefinite exponential growth) that makes me roll my eyes.
Moreover, who’s to say humans on the Serengeti were selected for intelligence? Maybe we were selected for better endurance than other large mammals? (E.g., I, an untrained human, can easily run longer than my dogs. Similar results hold for hunter-gatherer human vs. our simian ancestors.)
On another note, this neurogeneticist posits that humanity has been getting dumber due to some population genetics calculations that I don’t quite follow: https://pdfs.semanticscholar.org/cc63/c5e0bb322baa850f362b38be6c7835a483ce.pdf The gist of it is that the set of genes that determines intelligence is so large that the overall total selection pressure needs to be extremely high to select the set as a whole to maintain optimal intelligence. That is, you don’t need to be selecting for intelligence per se but fitness against all-cause mortality. That selection pressure has gone down precipitously since the dawn of agriculture, ergo dumber humans.
Whether or not the hypothesis is true, the fact that so many non-brain-related genes are implicated in intelligence make me wonder: to what extent does cognition occur in the endocrine system?
I too was stunned by the reasoning that got Hsu to IQ1K. The assumption that each mutation can be considered independent of every other – that epistasis doesn’t exist, at least for IQ – is prima facie preposterous. And this guy founded a Cognitive Genomics Lab?
Within the normal range, epistasis has been measured not to exist. H²-h²≈0.1.
There is a theoretical explanation: selection works linearly. Any trait that has been selected must have linear structure. And artificial selection has validated this on many traits, often moving 30σ.
Nitpick: This isn’t quite true. John probably knows more than I do about this, but there are limits to how big the ratio between the trigger and the resulting reaction can be. This is why the really, really big bombs used three stages, with a fission bomb setting off fusion which set off a bigger fusion stage. I don’t recall this being particularly easy to get right, but it’s been a while since I looked into it.
Actually, the third(and highest-energy) stage was fission. The radiation from the fusion is required to make the incredibly abundant U-238 fission, which it won’t do to any useful extent in a standard single-stage fission bomb. (Or so I understand it, it’s been a while since I looked into it)
You’re not wrong that that was a thing, but it wasn’t what I was talking about. They’d use a fission tamper around the fusion reaction to soak up the fast neutrons if they wanted high yield. That’s still part of a given stage. The biggest weapons had a third stage, another fusion (and maybe fission) stage.
Ah, interesting.
People aren’t entirely consistent in their use of “stage” in this context, but the Tsar Bomba was by this standard a four-stage device as tested and a five-stage device in its nominal operational version (100 megatons, deployed by Proton rockets because Russia didn’t have the Saturn V).
Fission primary (Nagasaki-style A-bomb), Sakharov-Zel’dovich style radiation-driven fusion implosion device (Teller-Ulam for you Hungarian chauvinists), neutron-driven fission in the uranium tamper, driving probably six more Sakharov-Zel’dovich fusion stages, and they mercifully used lead in those tampers because if they’d included the last fission stage (well, six parallel stages) the fallout would likely have killed a few thousand Americans and Canadians.
The physical principles are the same for the “upper” stages, but the engineering is increasingly complex because the behavior of each stage depends on those that came before. In a highly nonlinear fashion for the fusion stages, and dependent on turbulent radiation hydrodynamics. Considering that ordinary turbulence is one of the Millennium Prize problems in mathematics, and IIRC the only one for which “Maybe this can’t actually be solved, if you can at least prove that we’ll give you the prize” is an acceptable solution, I don’t expect that pondering the physics and simulating designs via Deep Thought is going to provide arbitrarily-large nuclear kabooms. Even for an AI, the biggest bombs that actually work are going to be maybe 1-2 stages larger than the biggest bombs that have been tested.
Wait. 6 parallel tertiary stages? I didn’t know about that. How did I not know? (Ok, that one’s rhetorical.) And what’s your source on there being 6, because all Nuclear Weapon Archive says is ‘multiple’?
Just memories of an old discussion, alas. Tsar Bomba was built too fast to be a completely new design, so the multiple tertiary stages were probably copied over from an earlier device. IIRC it was George Herbert, myself, and the NWA’s Carey Sublette going through the list of possible candidates and saying “six of these would fit the yield and dimensions of the Tsar Bomba, nothing else looks close”, but I don’t recall the details. It’s certainly not a known fact, just an educated guess.
Ah. Makes sense. I’ll file t hat one away.
Still, the H-bombs increased an order of magnitude in less than 10 years, and the fact that they never got any bigger than that seems to have been a result of the lack of any use for such large bombs rather than the scaling problems. To all appearances the scaling problems were solvable.
I’m not sure this is really true. If the objective term we choose is “explain the universe in a novel and accurate way” then Joe is a chimp next to Einstein. Or a mouse. Or an Amoeba. Einstein could do everything Joe can do (and most of them better) AND he could do this other thing that Joe, and almost no other human being on the planet, could do. Who are you (or Eliezer or me) to tell us with any confidence how close Joe is to Einstein? I don’t mean this to be rude, but how can we confidently measure intelligence while still lacking the ability to replicate it? What does it mean to say that a chimp is more intelligent than a squirrel? I’ll grant that a chimp is smarter than a squirrel, but they have evolved in such different environments (and only one of them is thriving and the other struggling, and it isn’t the “smart one” thriving) that it seems odd to talk about the magnitude of difference between them.
But Einstein couldn’t drive a car, making him dumber in that realm than 90% of the adult population of the U.S. Maybe he had the potential – maybe not? – but he never developed the potential into working intelligence.
It’s only relevant if you think Einstein couldn’t learn to drive a car due to his intelligence, or couldn’t learn how to do basic plumbing or any number of things that can be taught to the general public. Even then the ability to invent something new is far rarer and valuable than the ability to learn a skill.
My understanding of the models that predict the rapid onset of super-intellegent AGI is that this critical mass or inflexion point is when an AI is “smart” enough to start re-engineering its own intelligence.
It’s a moving target, though – the smarter it is, the smarter it’ll need to be in order to understand itself. Could you re-code your brain to improve your mental acuity, even if you had the ability to perform flawless synapse-level surgery? Could any human?
I think the answer to this question is “depends”.
We actually understand quite a lot about the low-level computational structure of the brain. If you could really run, like, a database query over synaptic connections in your brain, you might see some obvious areas for improvement. You might not even need synapse-level surgery.
It might be a “simple” matter of semi-randomly increasing the population of synapses that reach long distances across the neocortex, and then just going about your day and seeing if your brain starts taking advantage of those new connections, or if it just prunes them. You’d lose nothing by trying and stand to gain much, potentially.
Your brain is optimized largely for energy-efficiency, not raw compute power, and it may perform very differently if you could artificially improve the process of cellular waste removal and glucose delivery. A person operating at peak mental clarity 100% of the time is qualitatively different from that same person if they can only reach that state after optimal sleep/nutrition/caffeine conditions are met.
Or maybe you have a knob that just increases the volume of your skull, and another knob that encourages your brain to grow to fill that volume, and you just turn that knob up a notch every few days. Remember, we’re still architecturally limited by the birth canal, so we don’t know that “stupid” solutions like naively increasing brain volume wouldn’t work really well.
The above paragraphs have analogs to modern AI concepts. Once you find an architecture that works on a problem, the first thing you do is play with the hyperparameters such as the depth and breadth of the net, which is analogous to increasing the brain size. And there are techniques that involve randomly tossing in artificial synaptic connections that “cross levels” and seeing if the algorithm makes use of them.
A major problem that current self driving cars have is the “garbage can problem”. It seems that they tend to run into large brightly colored objects when they are left on the side of the road. I asked about this the last time I saw the usual suspects and they of course said, “yes, yes, it is a known problem.” I think that this gives a sense of how near/far we are from done. Self driving works almost all the time, because almost all the time there is nothing to do. Driving is remarkably easy (at least in the US) so computers can wing it. The hard parts involve recognizing what objects are in the vicinity, and we are bad at that. Building models of what the object might do on the near future has not even been touched.
There is a tendency to constantly return to fiddling with sensors, as better sensors help everything, but self driving is not a sensor problem. It is almost entirely a software problem.
As for “fully-loaded prototypes performing far better than a human”, the people who are running the projects do not think so, but their marketing people might.
Oh, so I’m not the only crazy naysayer here. Yay!
To go slightly meta, some of the smartest humans on the planet have been working on AI for over half a century. Let’s say 80 years by the time they make a computer as smart as an AI researcher. What will make that computer any better at creating superhuman AI than the equally intelligent humans who took close to a century? Perhaps it’ll be able to throw hardware at the problem more readily, but that’s hardly overnight AI explosion territory.
Also, note the iron law of computer science: No algorithm that you actually give a shit about scales linearly.
Personally, I suspect (but can not prove) that to the extent that you can reasonably describe something that you’ve thrown hardware at as smarter, it will get linearly smarter with exponential increases in hardware. But it’s essentially guaranteed that at the very best, it will get linearly smarter with quadratic increases in hardware.
The same factors that make us more likely than the ancient Babylonians to create AGI, is the short answer.
We aren’t smarter than ancient Babylonians, so try again.
That was my premise, you Babylonian. Do you have a brilliant argument you forgot to mention? Do you have any reason to think an AI of human level – technically, the question was about human AI researcher level – couldn’t absorb more knowledge than humans could physically read?
I guess that for any value of “absorb more knowledge than humans can” that actually means anything interesting, you’re presupposing superhuman AI.
Like, sure, if an “AI” is “of human level” except that it can read, absorb, and draw correlating insight from terrabytes of data relatively quickly, and that drawing correlating insight from large amounts of data is how you advance intelligence technology, then sure, your superhuman AI may or may not be better at developing, er, superhuman AI better.
But that’s not really why we’re better than Babylonians at creating AI.
…OK, the idea of AI reaching human level in the areas where it hasn’t done so, but somehow losing the capabilities of modern AI, doesn’t make the slightest sense to me. We’re not required to imitate evolution’s mistakes.
And yes, our knowledge is exactly why we can outdo Babylon.
I think the idea is that once we’re able to create AGI, we’ll have understood how minds in general work, and so we can simply copy that blueprint and just scale it up with more resources.
If we get to AGI by a complicated mess of neural networks training neural networks training neural networks, then we might have built something we understand very little about, and then the resulting human level AGI won’t be any the wiser either. Depends on which of the scenarios seems more likely to you.
My suspicion is that it’s mathematically impossible to “understand how minds in general work”, except for minds far, far less intelligent than our own. And that this will be true at all intelligence levels. Humans can plausibly understand a worm brain, and some hypothetical post-AI-explosion supergenius computer can plausibly understand a human brain, but I doubt a human will ever understand a human brain, or that the AI will ever understand itself.
That said, it’s possible to improve things by various means even when you don’t understand the system – this seems to be a decent description of about half of pharmacology, for example, as well as the evolution that created us in the first place. But it’s much slower and chancier.
I tend to agree; we’re used to being intelligent so for us the question isn’t so much “how did we get it” as “it inevitably happens so all we have to work on is scaling it up”.
Thing is, if you look around, human-level intelligence is pretty much confined to humans, and the processes of evolution certainly have not had a big control committee sitting on them saying “okay, we let you get away with the hominids, but no more tinkering with brains from now on”. So why not human-level intelligence in non-human animals? (Unless your answer to that is that humans were the first AI risk and we exterminated all competing intelligences, as Unfriendly AI will do to us).
Cephalods are reputed to be intelligent, maybe even approaching human intelligence, and there are stories of octopi in tanks doing amazing things:
And yet – it’s we who are keeping octopi in tanks and studying them, not the other way round. (Part of that may be due to their extremely short lives – a couple of years only. That again poses a problem: if large, complex brains mean a trade-off in lifespan, why do we live for decades?)
We look at ourselves and think “yeah, we’re really smart, but this was all achieved via a dumb material process. If we just copy that process in principle, we can easily create an intelligence equal to ours, and because it won’t be limited by a biological substrate there’s no end to how smart it can become”.
But maybe we really are special, some kind of freaky fluke that is one of those “well yeah in a multiverse any possible one-in-a-gazilion-octillion chances can happen” cases, and lightning won’t strike twice. We might get mouse or even chimp-level, but human-level? And better than human genius level?
All those superhuman computers doing arithmetical operations better than any single human or team of humans – but do they understand mathematics? Have they a theoretical concept?
That would be a very interesting result, but it’s really hard to imagine the shape of the proof. There are a lot of truly profound theoretical results that nevertheless don’t translate well into the real world.
Yes, the Halting Problem is unsolvable, but that doesn’t keep us from writing all sorts of programs that we can prove are correct.
Yes, the Traveling Salesman problem is NP-complete, and therefore intractable in general. And yet salesmen travel, though perhaps not on optimal routes.
Yes, Godel showed that a sufficiently rich formal system can express carefully-constructed true statements that cannot be proven within the system. But mathematicians did not at that point stop trying to prove things — there are still lots and lots of true statements that can be proven.
Lots of people — I hope not you, of course — grossly over-apply this kind of result for the nice feeling of seeing deeply into reality, but it’s kind of like the folks who look at 2000-year-old Indian philosophy and conclude that they had really nailed the notion of quantum entanglement all along.
I’m not sure how one would construct such a formal proof either. But much like how Fermat’s Last Theorem felt right for 300 years before it was proved(using methods Fermat could never have dreamed of), this feels right to me.
Bits and pieces can be understood, but it’ll never be like a financial spreadsheet where I say “Okay, if I double the amount I save every month, I expect to see the total savings go up, annual interest earned go up, and the time before I can retire to go down”. That’s a system I understand fully – a computer is doing the heavy lifting, but I could replicate the whole thing with pen and paper if I wanted to.
I don’t think it is possible for a human to start changing the construction of a human(or human-level) brain and be able to know what the results of doing so will be in advance. Guess and check, sure. But planned engineering? There’s vastly simpler things out there that extremely smart people with copious resources and long periods to work on it can’t engineer successfully.
And yet “guess and check” is exactly how planned engineering got its start. What if there was widespread guessing and checking for 4000 years? Would we develop an engineering of brain improvement?
If so, we’re just haggling over the price. Given that an AI can do guess-and-check with full backup capability, maybe it could cut that to 400 years? Given that it can progress at semiconductor speeds rather than protein transfer, maybe 40?
(But I am really glad to see that you aren’t inclined to make some kind of hand-wavy reference to Godel’s proof! SSC community is the best.)
Rubbish!
Don’t be daft. It trivially won’t take an AI a century to make another AI, because it will have access to its own source code (and, more generally, the computer science that produced it.)
Well yes, ctrl-C, ctrl-V will be an option in a way it isn’t for humans(though we do have a lot of fun trying to copy ourselves). But that only creates an AI of equal intelligence, not one that’s smarter. If there’s easy tweaks to the source code that make the AI smarter that are visible to a being of AI-researcher intelligence, they’ll probably be incorporated into the original by the human researchers, which means that the computer won’t have any low-hanging fruit for self-improvement.
It doesn’t need low-hanging fruit. It just needs reachable fruit. It not being “low-hanging” just means the takeoff starts slower. Once your researcher-equivalent AI — or rather, AIs, because there’s no way any researcher is going to stop with creating just one. And they’ll have them communicate, because what experimenter wouldn’t want to see what happens if they did? — figures out how to make itself a bit smarter, the new smarter AI will find it easier (than the researcher) to make itself smarter still. Of course how fast this takes off depends on how hard it is to raise AI intelligence; if the difficulty of raising intelligence goes up with intelligence, this process may be slow or even self-limiting. My guess would be that it is not, and once you have a few AIs around with AI-researcher-level intelligence and sufficient resources to play with, you’ll quickly end up with highly superhuman AIs.
Fair. My guess is that it is, so I’m an AI explosion skeptic. (I think it’s worth researching, but the probability isn’t that high.)
There’s a fairly good chance that “its own source code” in practice will mean petabytes of black box neural network data. That’s a better starting point than the human brain, since at least it is copyable and debuggable, but it’s still pretty hard to work with.
It probably takes hour or days to make a complete copy of it, and understanding exactly how it works well enough to improve on the processes that created it might take a lifetime or more of intellectual effort.
That’s just speculation, of course, but I think it’s a likely outcome extrapolating current research forward. A lot of AI risk discussion assumes that it will be trivial for AIs to self-modify, but I don’t think that assumption is warranted.
Longer version of this argument.
Google claims to have reduced the price of its lidar below $10k.
In the other direction, Tesla claims that the currently shipping cars have enough sensors do full autonomy and that all it needs is better software, this year. (That link gives two dates, “full autonomy” on local roads this year, but maybe occasionally asking for human input? tailing off to no human input in a couple of years.) [Maybe Telsa charges $6k to get the full sensors?]
It’s probably very hard to say with a straight face that “the hardware is good enough, we’re only missing the software”. I mean, theoretically it’s obviously true: a human can probably drive very well just from a camera feed (evidence: video games and simulators), and the difference between us and the computer is the software running inside our brains. But saying that, in practice, better software will give us self-driving cars for sure in a practical time frame appears to be overoptimistic… You’ll only know after you’ve implemented the thing.
A lot of the variation in human chess-intelligence is due to levels of training rather than genetics. Thus, it seems very likely that the variation in human chess playing ability is considerably broader than the variation in human innate intelligence since the amount of time spent studying chess varies so tremendously.
That’s a good point. To get a true sense of the variation in human chess-playing ability, you’d have to have everybody spending grandmaster-level time learning and practising chess, with incentives available for each person which keep them as intensely interested in chess as grandmasters are. I suspect that if you did that, you’d discover that the range of human chess-playing potential is quite a bit narrower than actually-existing chess-playing ability.
Part of the variation might not be in intelligence, but in things like competitiveness, endurance, lack of distraction, amount of (and triggers for) anxiety, etc.
If you’ll permit some math-nerdery in explanation, I’ve got a suspicion that you’re looking at something like a variable transformation problem here. Essentially, I suspect that while one can be “way superhuman” or “way subhuman” at the tasks described re: driving, one is going to see diminishing returns (positive and negative) as one gets more extreme in either direction.
Think of it like using Gauss-Legendre quadrature on a variable that lives on the whole real line. You need to transform that variable to something that lives on (-1,1) in order to use the method. Perceptual tasks may have a tremendous range in which one can be sub- or super-human—but it doesn’t matter HOW good/bad you are, it just matters if you’re good enough to miss the deer that jumped out into the middle of the road.
It actually seems perfectly reasonable to me that a computer that’s mixing sub- and super-human levels of ability is going to average out somewhere in the human range, because the human range probably spans from “functionally incompetent at all tasks, even if it’s not as bad as the computer” to “functionally expert at all tasks, even if it’s not as good as the computer”.
‘Intelligence’ looks pretty related to long + short term memory I/O speed, error rate, size, and longevity. Low IQ people have absolutely atrocious error rate and I/O speed in particular. Intuitively there would be very sharp diminishing returns on improvements in error rate and I/O speed as you become almost completely accurate and can process information about as quickly as you can acquire it.
I seem to recall some article which looked at IQ and GDP, and found that if you cut off a fair bit below 100 the correlation looks stronger. That would line up with this idea – before you hit diminishing returns every improvement matters tremendously. https://plot.ly/~randal_olson/14/iq-vs-income-per-capita-worldwide/ Looks like it’s the case at a glance. Keep in mind there’s some noise from natural resource economies.
Obviously the mind isn’t just a recording device, and there are people with photographic memories who aren’t geniuses. But if you’ve got the learning speed and recall down, man, that lets you do a lot. And if you don’t, it’s difficult to see how the analogue to ‘clock speed’ could make up for that.
The idea that there are diminishing returns to IQ is dismissed by people who know that you keep getting returns, say in terms of papers published or whatever else. But that’s competition, intellectual competition. Where you’d see diminishing returns if they exist is in some assorted real world tasks with some intellectual component. Which ‘the economy’ should be a fairly good cross section of.
Indeed if anything would throw this off it would be that in very high IQ places – such as major global cities – ‘the economy’ becomes really unbalanced, focused on intellectual fuck-fuck games. Like, imagine a horse race economy where odds calculations were the skill to have and everything rested on coming out ahead on average by very slim margins. This economy values intelligence very highly because it is in effect intellectual competition, but that doesn’t mean anything of value is produced by that competition – there’s just value sucked up by the winners. Wealth would accrue to those who are suckering rubes, but it wouldn’t be being produced by the main focus of the economy. Smarts would be economically productive without improving quality of life any. Large cities look a lot like that to me, sucking up lots of objectively valuable work hours and resources without outputting anything that clearly justifies the expense. Some mixture of a con and an expression of the power that flows from centrality and large social networks.
I’m guessing you haven’t been around animals much?
The thing you need to measure if you want to understand the difference is the surface area of the brain because all the actual cognition happens in the outer few millimetres of brain while the rest is just instinct, autonomous functions and reflexes. Most animals don’t even have such an outer layer at all so that their effective intelligence is zero.
I suppose it depends on the animal. Crows and chimps are wicked smart, and there are lots of examples of them passing multi-stage physical puzzles. What I really want to know and can find precious information on is what tasks and puzzles confound animals we think of as smart. Knowing exactly what they can’t do is as important as knowing what they can do. Where does the smartest species of crow top out at? What can’t it do?
There have been several proposed examples (e.g. tool use, language), but further research showed that in fact some were capable of every example proposed.
“Coming up with ways to define sapience so it only includes humans” fell out of favour, even though intuitively there must be examples.
When it comes to categories as broad as tool use and language then animals can do all of them. The answer to the question of whether they can use tools and language as well as humans is already well known (spoilers: they can’t), but of course, animals are handicapped in that a lot of smart animals don’t have the digits needed to use tools as humans do.
What I’m interested in is setting up a puzzle that a human would succeed at but an animal would fail at. We’ve already seen crows (Caledonian?) do puzzles where they need to drop pebbles in water to raise the water level so that they can grab something, or use a stick so they can pull another longer stick out of a cage so that they can use it to snare something, but nobody seems to have defined the limit of what they can do. Could a crow solve a rubix cube if a big one was set up that it could turn? Could a crow play chess if properly motivated and trained? What can’t they do?
Here’s a story which makes me wonder whether human-invented intelligence tests capture enough.
Some friends had a male cat. Every time they added a dog to the household, the cat would socialize a feral kitten and bring it home.
That is such a good story. I love it so much that the urge to share it widely even without any documentation is really, really, intense. Such a good story.
So, honestly: actual friends you knew? Have you seen this effect in this household over time, or is it just an anecdote related to you? I’m not trying to denigrate your story, I’m truly curious, and I really hope you personally are a witness to it even though that wouldn’t really justify my promulgating it. But it’s Just. So. Good.
Actual friends I know. However, I was told this as a story. I haven’t seen the cat bringing kittens home.
The friends don’t have a history of practical jokes/telling false stories so far as I know.
Whenever this kind of signalling derails my train of thought, I feel like I’m losing some kind of game.
Genuine question: What signalling is that? I detect none, honestly.
her instead of he maybe?
Her parents spent $5000 on a gaming computer but couldn’t or wouldn’t spring a lousy couple of bucks for a basic calculator? 🙂
A question for you: do you speak a language with grammatical gender?
I find that this sometimes surprises me when the person is being referred to as someone whose sex (or real life gender, as opposed to grammatical gender) is different from the grammatical gender of the word. I’ll always refer to sex when talking about a person’s gender and gender when talking about grammatical gender.
For example, in Portuguese, “kid” might be translated as a female word (“criança”). Yes, there are other equally good translations that are male, but those explicitly assume that the kid is male (“miúdo”) or female (“miúda”). When speaking in general, a “criança” is a She in Portuguese. So it might slightly surprise me if later in the text the generic word kid is referred to as a He. Individual kids are still male or female, of course, when referred by name.
English doesn’t have grammatical gender in many places in the language, so people can use any of the pronouns. For example, this riddle doesn’t work in Portuguese at all (I’ve read it originally in Portuguese and had to remind myself it as probbaly a bad translation from something in English):
The obvious explanation is that the surgeon is a woman. The less obvious ones are that the surgeon is one of two gay fathers, but that stretches the definition a bit. We might as well say that one of the fathers is the biological one while the other one is a stepfather, whatever. In a riddle context we have to assume that “son” means someone who shares genetic material with the parents, not “whoever you wish to call son”. Nevermind.
The point is that in Portuguese, you’d have to say “o médico” or “o cirurgião” (the male doctor or surgeon) or “a médica” or “a cirugiã” (the female doctor or surgeon). You can not tell the story from a gender-neutral perspective. So, guess my surprise when I say the riddle written with “o médico” (male) and then was berated for showing gender bias by assuming the doctor is, in fact, male. Which is kind of dumb because in my country there are way more female doctors than male doctors (anecdotal evidence, but will be true in the future for sure because way above 50% of new graduates are female).
So maybe this might be a source of distraction for you if your language has a more pervasive form of grammatical gender.
It does cause me a little mental hiccup, both because “he” was taught as the gender-neutral pronoun in school (at least in the regressive dark ages of the late 1990s) and because a kid with a $5000 gaming computer is quite likely to be male. It’s like if someone stopped in the middle of a conversation to do a “Haha, got your nose!” trick and then kept going like nothing happened.
Of course, I’ve always just taken this to be one more natural impulse one has to suppress to get along in modern society. Not much to be gained from culture warring on every trivial annoyance.
I’m not sure how I feel about the general question, but I think that there’s a very important distinction to be made in your examples depending on the “relevance of human rules and input” to the AI.
In particular, it is my understanding that chess AIs up to and including Deep Blue (and possibly beyond that) worked very roughly as follows: the computer enumerates lots of potential positions and decides what to do based on heuristic scoring mechanisms designed by really good human chess players. In other words, though there are all sorts of subtleties here, Deep Blue was still quite reliant on humans’ ability to (design simple rules to) decide how “good” various different chess positions are.
It’s therefore not too surprising that our chess programs looked roughly like human chess players. Essentially, how good such an AI is depends on how much it can enumerate and how good our “scoring” system of chess positions is. (FWIW, I believe that in practice a lot of this scoring is actually used to lower the number of positions that it is necessary to enumerate by ignoring certain branches of the tree.) And, our scoring system of chess positions will necessarily be an imperfect approximation to something that a group of great human chess players could come up with. Such an AI can get better than humans (and they have) if its ability to enumerate positions outweighs this, but (ignoring the currently absurd possibility of enumerating nearly all possible moves), the AI will still be constrained by human skill to some degree. So, it seems much more likely that such an AI would tend to fall somewhere roughly between slightly worse than the worst humans or slightly better than the best humans.
AlphaGo, on the other hand, used a qualitatively different approach. As I understand it, AlphaGo was based primarily on a machine learning algorithm, with very few (if any?) human-designed heuristics and rules. The machine learning algorithm learned a lot from data of human games, so it was constrained a bit by human knowledge in that sense. But, this seems qualitatively different than using human-designed heuristics. Plus, AlphaGo also “trained” a lot against itself. To me, it seems much more plausible that such an AI would end up either way better than all humans or way way worse than all humans, and there seems to be little reason to expect it to perform somewhere within the range of human ability.
Of course, as I mentioned, AlphaGo is tied to human ability in that it is largely trained on human data. If we designed AIs that used machine learning on non-human data (say, data obtained by physical experiments, or self-generated data), then I think we would have no reason at all to expect them to perform like a human in any way. So, in this case, I think we should expect the jump from mouse to man to be much much harder than the jump from Joe to Einstein. However, I don’t know of any examples of this where we’ve achieved much more than mouse-level intelligence so far :).
I think that this misunderstands how AlphaGo works. It’s primarily based on Monte Carlo tree search, and uses machine learning to improve its sampling algorithm. I don’t think that this is all that different from a program based on alpha-beta pruning using sample games to improve its static evaluator. And while I’m not certain whether or not chess programs used training to improve their evaluators, I know that even early checkers programs did this.
This is not an accurate history of computer chess.
There were two broad schools of thought. One school believed that the path to high-level computer chess involved consulting chess experts and writing really good heuristics. The other school of thought wanted to examine as many moves as possible, and was willing to use simplistic heuristics to speed itself up. Empirically, the latter approach beats the pants off the former.
Deep Blue evaluated 20M positions per second. To reach that point, it used purpose-built hardware that extracted various features of the board position in a mere handful of cycles. Any human heuristics that couldn’t be calculated nigh-instantly were ruthlessly pruned.
Human chess skill was not a meaningful constraint on Deep Blue’s performance.
Personally, whenever I discover empirical data that contradicts my common sense, I take it as a signal that my common sense could be wrong. Just saying.
Also, given how much of the definition of “common sense” in this group is “Eliezer wrote this thing a decade ago, and we’ve all assimilated it into our brains on an instinctive level since then”, one needs to be careful about assuming it’s “true common sense”, for lack of a better way to phrase it. This could well be the equivalent of a Christian group disputing evolution because it conflicts with the common-sense notion that God must exist.
This is unfair. My reading is that Scott basically winds up concluding, “Maybe Eliezer is wrong and human ability range is actually quite wide.”
The initial setup to the post, on the contrast between speculation from Eliezer Yudkowsky and data-driven thoughts from Katja Grace highlights why I find the the AI-obsessed “rationalist” world so comical. I’m sure Eliezer Yudkowsky is a nice person, but why should I care about the AI thoughts of someone who isn’t a computer scientist, skilled programmer, or someone with hands-on accomplishments in science / technology? I really don’t understand this.
My own opinion on AI is that we understand its potential course so little that (i) actual data are important to look at, and (ii) any pontification is futile, and says more about our own fears, hopes, or biases than anything about the future.
Why whould we care about the AI thoughts of an anonymous Internet blog commenter using the name Elephant?
At least Eliezer uses some kind of logic that we can evaluate. Shouldn’t we consider the argument rather than the person making it?
I was going to add: “of course, you shouldn’t care about my opinion, either,” but I thought it too obvious.
I’ll try to address the second point later. As far as I can tell, there’s no logical argument, but rather just strongly worded beliefs, which beg the question of why to favor them over other beliefs.
https://en.wikipedia.org/wiki/Eliezer_Yudkowsky#Academic_publications
I’m not sure what the point of the “Academic publications” list is. These seem in general to be just published essays / opinion pieces.
Have you not heard of Nick Bostrom or Cambridge University Press? They certainly think his work on AI is valuable.
Something worth noting about those chess/go graphs is that while they’re linear for computers they’re generally logarithmic for people. For example, there’s a saying in Go that the gap between a complete amateur and 1dan is the same as the gap between 1d and 2d, in terms of the work it takes to improve. Similarly if you look at the number of people at each skill level, you get an exponential decay. Meanwhile, AI got from 1d to 2d as easily as it did from 10k to 9k.
So the conclusion from these graphs is that AI probably advances exponentially, but not superexponentially. This might break down once you make an AI that knows how to design AI better than humans (a fundamental difference from game playing AI).
Since there are a lot of humans, you’d expect the range from the worst to the best humans to be about twelve stdevs. So if that’s the same as the human/chimp gap, the average chimp has an iq of around negative sixty. Which doesn’t sound that crazy.
On the other hand, Moore’s Law is also exponential. The power of the AI could just be scaling linearly (or maybe polynomially) with processing power.
I think someone above said the improvements are about equal parts Moore’s law and algorithmic improvement, which supports this.
Maybe the reason humans are so much smarter than animals is because we didn’t evolve.
It’s possible. But then, it’s also possible that the reason why whales are so much bigger than other animals is that they didn’t evolve. God really just likes huge things, and evolution wasn’t doing the job, so he made whales. (Brains? Who cares about brains? Those things are tiny!)
You can come to all sorts of fun conclusions if you start using arguments like that.
And also using evolutionary arguments, as exemplified pretty well on this thread.
In most domains, there is probably a bigger difference between humans and animals than among humans but when it comes to math ability, there is a bigger difference between Terrence Tao and a slightly mentally handicapped person than between the latter and an animal.
There’s actually some math-based questions where the average human is worse than the average animal of some species. Usually ones involving probability and decision-making – https://www.livescience.com/6150-pigeons-beat-humans-solving-monty-hall-problem.html for example.
I don’t think anyone is surprised that pigeons are smarter than undergraduate students.
Joking aside I think the primary inhibitor for humans with these problems is that the odds are static. Socially we have evolved to try to figure out what the other person is up to to put it crudely. If you select A and then the game show host appears to try to convince you to choose B there is some chance they are trying to trick you for their own benefit. Route answers in human interactions are rare beyond banal pleasantries.
Whenever I’ve seen this in the past, it’s been missing the emphasized portion. I’ve always assumed that it must be there, but only because it’s the only way I can see it making sense to switch, and it’s not something I’d consider obvious having not seen the show. If he always picked the door the prize was behind when possible, then obviously you should never switch.
…he opened one of the two doors you didn’t pick. You saw what was behind it.
Yea, so if you saw the prize, either you’d instantly lose or (essentially instantly) win depending on the exact rules.
So if you watched the show, and that never happened, you could reasonably infer that the host knew which door the prize was behind and never picked it. Without having watched the show, however, this was not obvious at least to me.
I mean… you could also reasonably infer that if you kind of basically understood how game shows or games in general work.
Having the host be unaware of the location of the prize with the contestant instantly losing if the unveil showed the prize seems totally reasonable to me, particularly if the contestants are chosen from the audience with a variable number per show (thus eliminating the need to try and keep timing constant). Admittedly, however, I haven’t watched many game shows.
Even if you don’t like the idea of a potential instant win/loss after the reveal, the host could still put his finger on the scale if he knows where the prize is and can skip the reveal round at his discretion. Particularly useful as it would be at least a bit less obvious than the other way around.
Truly solving the Monty Hall problem absolutely requires a Theory of the Mind, because the problem as stated is incompletely specified and the ambiguity is left to an agent with a mind (and a name, so you can in principle look him up and ask him, which people have done).
I believe the correct answer is approximately “switch you are a bubbly telegenic young woman and/or the show has had a recent string of losers, otherwise not, and definitely not if you are going to come across to Monty as a tricksy game-theorist type”.
Are you saying that “[he] would always open one of the remaining doors that he knew did not contain the prize” is incorrect (which would explain why I’ve never seen it associated with the problem before)? If you weren’t someone he wanted to win, what would he do?
I don’t believe Monty Hall ever opened the door with a prize in a 3-door situation. However, I don’t think he always offered the option to switch either. And there was one variant where two players would choose a door, and Monty Hall would open a losing door picked by one of the players, and offer the other player the option to switch: in that variant , it’s 2/3rds to NOT switch.
That is the case(*). Monty was allowed but not required to open one of the remaining doors and offer the option to switch. He made this decision with full knowledge of what the prize was and which door it was behind, and with competing objectives including making the show entertaining, keeping its prize budget low, and enjoying himself.
Monty thinks the show would be plenty fun if you’re the loser and doesn’t want to explain to the executives why they have to buy another new car or whatever? Easy. If (2/3 chance) you picked one of the doors without the prize, he would simply open the door you picked and reveal you as a loser. If you got lucky and picked the door with the prize, then he opens one of the other doors and asks if you want to switch. If you don’t, he asks if you’re sure about that. Asks you to think how foolish you’ll feel if you lose due to your stubbornness even though he gave you a second chance.
If you do stubbornly insist on sticking with your original pick, you have 1/3 chance of winning the prize, because they don’t actually cheat. If you switch when offered the chance, Monty gets to decide whether you get the prize.
* At least in the real-life game show. The logic puzzle merely leaves the agent’s ruleset, algorithm, and long-term behavior undefined while name-checking the real person.
Regardless of whether he knew or not, that doesn’t change what course of action gives you a greater probability of winning given that he in fact opened a door that in fact did not contain the prize. You are not meaningfully faced with this decision to switch or not if he skips the reveal round, or if he reveals the one with the prize, even if those are things that sometimes happen to other people.
What?
If he opens a door in an attempt to trick you into switching away from the prize, then your odds when switching are 0 and your odds when not switching are 1. If he opens a door in an attempt to help you because you chose wrongly, then your odds when switching are 1 and your odds when not switching are 0.
As such, all that matters is the relative odds of those two scenarios.
That’s not true. If the host always opens one of the other doors at random (and if it’s the prize you lose), then if the host opens a door which did not contain the prize, switching gives you a 50% probability of winning (as does not switching).
If the host only opens a no-prize door and offers you a chance to switch when the door you picked has the prize, switching gives you a 0% chance of winning.
If the host only opens a no-prize door and offers you a chance to switch when the door you picked does not have the prize, switching gives you a 100% chance of winning.
If the host always opens a no-prize door and offers you a chance to switch, then switching gives you a 2/3rds chance of winning.
These scenarios are all indistinguishable to a contestant who doesn’t know the history of the show and isn’t given the rules explicitly.
@MugaSofer
Uh, that’s what I said? My point was that scenarios where he doesn’t open the door aren’t relevant because he did open the door, and scenarios where the door he opened contained the prize aren’t relevant because it doesn’t, and it doesn’t actually matter why those things didn’t happen.
@The Nybbler
Wrong.
What I am disputing is that there is a difference between these two scenarios (I did not address the other scenarios, I was not replying to the post in which they were brought up). In both cases, switching gives a 2/3 chance of winning. Whether branches exist where he would have opened the door with the prize were possible is irrelevant because you know you’re not in one.
@random832
Work it out completely. Prize is assigned to doors randomly. Without loss of generality, assume you pick Door A.
P(Door A is a winner) = P(Door B is a winner) = P(Door C is a winner) = 1/3
You win by switching if
1) You are given a chance to switch and
2) Door A is not the winner
These are not necessarily independent events. So
P(win by switching) = P(A is loser) * P(chance to switch | A is loser)
Obviously P(A is loser) = 2/3.
In the “random door case”, assuming A is a loser, your chance of being given the chance to switch is 1/2 — Monty will open one of the other doors at random, and if A is a loser, B and C are equally likely to be winners, and you get to switch only if Monty picks a loser. So you’re partly right, P(win given switch) is not 1/2 in this situation: It’s actually 1/3, and therefore you should not switch.
In the “always empty door” case, assuming A is a loser, your chance of being given the chance to switch is 1. In that case, it’s 2/3rds to switch.
Nybbler-
I think what they’re saying is this:
Yes, initially you are picking one door of three, with zero information. So your best guess is that you have a 1/3 chance of being right.
If Monte is an automaton who always reveals a loser door and lets you switch, then switching gives you a 2/3 chance.
But Monte is not an automaton, and doesn’t always offer that choice. Why is he offering it to you? Does he think he can get you to switch away from the right door? Does he think you will think he’s trying to get you to do that? Or is he trying to trick you into sticking by your choice, by showing you a goat door, so you’ll feel better knowing at least you didn’t pick the goat?
I mean, he knows which door is right. If you can get inside his head, you can shift the odds in your favor. (And conversely.)
I have to admit it’s a take on this puzzle I’ve never run across elsewhere.
It seems you haven’t looked at areas where computers crush humans. Multiplication, weather prediction, much data processing… There may be an availability bias in that as soon as a computer crushes humans, noone cares/tracks/mentions it.
Let’s look at theory 3 again: many human activities involve a mix of skills. Computers start challenging humans when they master some of the skills. They win when they master enough skills, or are good enough at what they do master to compensate for the lack. And then people soon forget about tracking computers in that activity.
So the main problem with computers is the lack of general intelligence. They can’t develop a skillset they are missing, and need to wait for humans to put those in by hand.
This kind of language annoys me, because in practice what computers have is a single area of expertise: doing floating point calculations very fast. The only qualitative difference between multiplication homework, weather prediction, Tetris and Go is in the models that humans have specified and let run on the computer. From the point of view CPU/GPU/TPU it’s all processing that is fundamentally very similar. (Just that for the Go model to be useful, one needs a TPU or several GPUs instead of one CPU to compute those results.)
Domains where computers beat humans are domains where humans have found models that are amenable to the numerical computations. The question of AGI is can we build a similar model for all the important human cognitive capabilities, and how? (And singularity hypothesis is concerned about what happens if we can build an efficient model for model-building, in particular)
>doing floating point calculations very fast.
I’d say it’s more running through algorithms perfectly (and fast), which is a much more genralisable skill, given the right algorithm.
Along the lines of what meltedcheesefondue said, the Church-Turing thesis roughly implies that if computes can do this, they can in principle do anything. It’s called a thesis because it certainly hasn’t been proven, but it’s also many decades old and nobody has managed to prove it false either. So saying “computers can only do this” isn’t really limiting them at all. Though of course what’s so far possible in practice lags a little bit behind what is possible in principle in this area, as you note.
Giving it some thought, of course everything in the brain reduces to chemical interactions, so maybe that’s comparable to the logical operations on microchip in some sense.
However, currently still everything computers do is running human-designed models. Most often when computers “can do speech recognition”, more true description of what happens is that no, computer carries out some arithmetical operations and it’s the Hidden Markov Model that is actually running the show. HMM is an abstract concept / model created by humans. Even the radar antenna designed by an evolutionary algorithm is a product of algorithm we wrote. (And the evolutionary algo software certainly ran on top of OS that was engineered by humans.)
Especially the “weather prediction” thing got me peeved, because I happen to know meteorology people (okay, passing acquaintance but still) and given the amount of human thought goes into building all those weather models, it certainly is not a matter of computers beating humans. My understanding it’s more of a question which team has the better differential equations than other. It’s a question of very carefully crafted human thoughts beating other such thoughts.
This is why I think the question of AGI is fascinating: currently computers are not actors in the same sense as e.g. mice, and when we say “computers do X brilliantly” I think it’s far too easy to miss that point. However, the RL agent development by DeepMind et al is our first step into path that might lead to a future where things might be otherwise and we have software (“a model”) that is genuinely capable of independent, autonomous model-building. I think we should remember this in our everyday language.
It’s more correct to say that human+computer teams beat human and computers.
Androids FTW.
This is such an annoyingly common misconception. Solving the differential equations is obviously *sufficient* to catch the ball, but its not necessary at all. An alternative would be a closed-loop control theory sort of paradigm. Ie, rather than actually predicting the balls’ trajectory and calculating where your hand must be at time X, simply move your hand to where its directly between you and the ball over time, and the ball and hand will inevitably collide.
You’re right. How about archery or long-distance marksmanship? Military sniping is basically down to a science, it’s true, but in order to hit moving targets without spending much time aiming, you’ve got to do some pretty complicated equations.
Or, as is more likely, you use the brain’s differing architecture to sacrifice the pinpoint precision of calculus in order to instead complete a bunch of very simple equations at the same time for a chance to mess up but much higher speed. Not precisely the same as having a big chalkboard in the brain, but it’s something pretty gosh-darn impressive.
(Worth noting: it’s not that simple to just move your hand between you and the ball. It’s taken a lot of effort and some very expensive parts to get highly specialized robots good at it, and humans have to practice for a while before they can do it right. Again, sure, not the big chalkboard, but it’s nothing that can be waved away just because we can express the concept in simple words. After all, the way you walk a tightrope is just by putting one foot in front of the other.)
Not to mention that at the low hardware level, I find pretty unlikely that the brain manipulates symbolic equations. It’s just how we like to talk about them. But the concept of equations and performing computations is a very high level abstraction that took millenia for the high level cognitive processes to develop; not probably very much alike what the mess of wet biology and neurons that is the brain does under the hood.
Nassim Taleb tackles this specific canard as an example of the “Overintellectualization of Life” by pointing out the actual heuristic we use is known (citing two others):
https://medium.com/incerto/surgeons-should-notlook-like-surgeons-23b0e2cf6d52
…
Overintellectualization of Life
Gigerenzer and Brighton contrast the approaches of the “rationalistic” school (in brackets as there is little that is rational in these rationalists) and that of the heuristic one, in the following example on how a baseball player catches the ball by Richard Dawkins:
Richard Dawkins (…) argues that “He behaves as if he had solved a set of differential equations in predicting the trajectory of the ball. At some subconscious level, something functionally equivalent to the mathematical calculations is going on”.
(…) Instead, experiments have shown that players rely on several heuristics. The gaze heuristic is the simplest one and works if the ball is already high up in the air: Fix your gaze on the ball, start running, and adjust your running speed so that the angle of gaze remains constant .
This error by the science entertainer Richard Dawkins error generalizes to, simply, overintellectualizing humans in their responses to all manner of natural phenomena, rather than accepting the role of a collection of mental heuristics used for specific purposes. The baseball player has no clue about the exact heuristic, but he goes with it –otherwise he would lose the game to another nonintellectualizing competitor.
I think that perhaps the best conclusion to draw from all this is that we don’t really know what on Earth is going on with intelligence. Intelligence is among the trickiest philosophical issues to solve, although we’ve made progress by describing different kinds of intelligence (e.g. social rather than mathematical), and yet our common-language way of describing it is still as some kind of scalar? That one mind can simply be more “intelligent” than another, like you can just assign someone an intelligence-number and be done with it? IQ is great and all, but it’s measured across a non-complete set of differing intellectual competences and certainly isn’t the end-all and be-all to what makes minds sparkle and shine. To get from that to trying to plot all animals on some kind of linear intelligence graph is fallacious at best, and sophistical at worst. It’s part of why I don’t take much of the AI-threat concerns seriously: we’re just barely good enough to make artificial “intelligence” that can overwhelmingly specialize in one task and we think we’re knocking on the door to the secrets of the mind itself. Let’s just be clear: we’re proud that we can make specialized machines which can solve specialized problems which are highly vulnerable to mathematical approaches to some degree better than a general AI can – while this same general AI is able to do that and a host of other things, not least be totally fluent in one or more languages, which is so difficult that only one creature on Earth can actually do it! Not only that, but despite the best efforts of these language-expert creatures to get machines to do the same, the best we have are the most primitive solution imaginable and are terrible even at that! Perhaps once we can get a single computer to really talk, it’ll be time to take general AI seriously, but as things stand we aren’t even close.
Fair point: human brains do appear to be wired specifically to be good at language. The ability of even humans with significant congenital brain defects to speak and understand languages strongly indicates that, as well as our understanding of Broca and Wernicke’s areas. This makes criticism of AI for not being able to speak just the teensiest bit unfair, as we’re probably geared precisely to be outstanding at it. At the same time, I have a sneaking suspicion that what “real” intelligence might require is precisely the ability to use language, given how language functions with regards to the mind. But that’s an essay by itself, so I’ll leave off there.
>Or maybe the first graph representing the naive perspective is right, Eliezer’s graph representing a more reasonable perspective is wrong, and the range of human variability is immense.
How is Eliezer’s perspective more reasonable? There doesn’t seem to be any evidence in favor of it.
Dogs typically understand 100-200 words, up to 1000 for exceptional dogs (or with exceptional training) http://www.animalplanet.com/pets/how-many-words-do-dogs-know/
Cats understand fewer words (25-35), but that may be because they are less eager to learn? http://blog.mysanantonio.com/animals/2012/07/the-intelligence-of-dogs-and-cats/
The jury is still out on whether cats are smarter than dogs, or vice versa:
http://www.huffingtonpost.com/joan-liebmannsmith-phd/the-cat-vs-dog-iq-debate-revisited_b_1572610.html
A chimpanzee understands 450 words, but is able to do more complex things with them and express himself (herself).
http://www.dailymail.co.uk/sciencetech/article-1317838/Kanzi-amazing-ape-speak-humans-understands-450-words.html
I guess a typical human can recognize on the order of 100 000 words, so by that (rather unfair, since we depend on language) scale, we are a thousand times “smarter” than these animals. I found some articles about the vocabulary of people with Downs, which might give an indication of human variability, but they were paywalled.
Please link the article on the vocabulary of people with Downs. It sounds interesting. Some of us work in academia and have access to those journals…
I’m not sure what constitutes recognizing a word, but a very smart (2sd +) American adult at peak vocabulary knows the meaning of about 40,000 words. More typical is 30,000.
I’m sure if you included words that people recognize as actual words in the dictionary but which they cannot actually define you’d get a much higher figure, but I’m not sure whether that’s a useful measure of anything.
http://testyourvocab.com/
I guess I have a vocabulary of tens of thousands of words in at least two of the languages I know, polyglots can learn tens of languages. But in any case, the direct comparison to animals isn’t all that interesting, since humans are language-oriented in a way animals aren’t. Any way you flip it, we have many orders of magnitude better resolution at understanding words, and this probably overstates our general intelligence advantage by some, but not all of those magnitudes.
I suppose most readers are aware of this, but IQ is a seriously misleading way to measure absolute mental capability. By definition, average human IQ is 100 and the standard deviation is 15. This means that a negative IQ is not only possible, but that a similar number of people have a negative IQ as have an IQ over 200. (Six sigmas correspond to about three per million, according to manufacturing process literature). In any case, IQ doesn’t let you claim that Einstein (hypothetically with an IQ of 200) is “twice as smart” as the average, such a term would be just meaningless.
Which I think is the whole confusion behind the posting, we don’t have any good ways of comparing mental capability on an absolute scale.
Anyway, I did a visualization for my machine learning class (on the blackboard only, sorry, not preserved for posterity), showing a rough picture of the development of various technologies (natural language processing, self-driving cars, chess, algebra and calculus, etc) and the y-axis having categories of “works at all”, “worse than human”, “better than some humans”, and “better than any human” – with the (admittedly not very well founded) conclusion that going from better than some to better than all often goes quite quickly. YMMV.
Another way to compare is by the number of neurons in artificial and biological systems. With the caveat that an artificial neuron is not particularly comparable to a biological neuron, it still reflects the capability of an organism or an AI system to solve complex tasks. See the figure in http://www.deeplearningbook.org/contents/intro.html, figure 1.11, page 27, where a linear extrapolation indicates that we will be building artificial networks with a comparable complexity to the human brain around 2056.
Very cool figure, thanks for the link. 🙂
No; 4.5 sigmas correspond to about three per million; you’re supposed to leave a 1.5 sigma radius safety buffer for the process mean to shift around in.
6 sigmas, with no shift, is about 1 per billion.
Right, thanks for the clarification. Still, this leaves us with perhaps a couple of persons with an IQ above 200, and the same number with a negative IQ, statistically speaking. (Which is a statement that probably shouldn’t be taken literally, IQ tests are only valid for a certain range, and it’s probably not meaningful to talk about IQ too far from the mean.)
I’ve also seen statements that indicate that IQ is only beneficial up to a point, and that after that, differences in IQ no longer reflects work performance or other correlates. Is this a defect of the IQ tests, perhaps due to their limited ranges?
Yudkowsky’s argument might not be in-congruent with the data. If the intelligence gap between humans and animals is large, but the gap between the least and most intelligent humans is small in comparison and we expect the intelligence of AI to follow an exponential curve through these samples, then it follows that, when the AI is developing over the range of humans, its progress looks linear. This is for the same reason as when you zoom in on any smooth curve it eventually looks straight.
The first plot you showed looks like linear regression applied to a data set of computer capabilities in which an exponential could have fit quite well, considering the clustering of low success computers in the 1950’s. Has a Chi-squared test been done to see if a linear model actually fits the data better?
The second plot also looks quite bereft of data, enough that I could draw a straight line through it and you mention that alphago’s recent success would be an uptick on that plot.
Is it possible that the problem where dealing with is that animals just don’t have intelligence, at least as we define it for people, and people do. In other words that humans have certain fundamental ways of thinking that animals just don’t and that therefore the range of humans can be very large and take sometime to surpass because some humans really do have almost no intelligence (which is still way better than none) and some have a lot. Obviously this couldn’t be true for all aspects of intelligence but might be for some.
It’s actually pretty easy to set up a compelling case for “intelligence” for which this exact model applies, although it does mean that we have to toss out a lot of complex cognitive tasks as being fundamentally “unintelligent.”
Let’s say that “intelligence” is precisely the innate skill required in order to successfully relate “real” phenomena, in all their varying forms and indistinct nature, to abstract structures like language. That is, all beings with eyes can look at trees, but only intelligent ones will declare that they are trees as a separate category from grasses, herbs, and shrubs. Carrying this further, more intelligent individuals will identify separate species of trees, and perhaps the most will identify groups of similar species, like oaks and pines. This definition decently matches our innate intuitions about intelligence, in that we regard people who are able to describe things which are already out there in the world in comprehensible terms as being very intelligent. (To echo Neil Gaiman’s comments on the late Douglas Adams, a genius is someone who sees the world in a different way than regular people do and can make them see it in the same way – to communicate it effectively.)
So, does this link up well to your criteria? It neatly separates out all other animals, barring possibly some extremely high-intelligence ones like dolphins and chimps and Alex the African Gray Parrot, as being unintelligent, and further establishes certain humans as exhibiting little intelligence. To this extent, it fits those criteria perfectly. Further, it takes all tasks which computers have surpassed humans at and categorizes them as inherently unintelligent: rather, it was those humans who programmed the computers who demonstrated actual intelligence by binding down real-world phenomena into some form of code. An interesting side-effect of this is that very few human activities actually count as demonstrating intelligence at all, which does prove problematic.
The limitation of using this definition is simply that it doesn’t deal at all with the various other mental skills which we regard humans highly for: good memory, fast calculation, hand-eye coordination, etc. Although they might be different in kind from what we called intelligence, a good number of them seem to go hand-in-hand with it. It also gives us trouble in separating things like octopi, which seem pretty clever but don’t have a lot of word skills, from things as dumb as pillbugs. These aren’t knockdown critiques, though, but places the theory could be expanded.
Either way, I think that answers your question. Yes, it is possible, but we might not have a totally clear answer without a lot more work.
I’m not sure about that. Current neural network systems are able to recognize quite abstract classes, such as chairs and mammals, and I don’t think it is correct to ascribe that level of “intelligence” to the intelligence of the programmers – they are undoubtedly very clever, but the actual implementation is about linear algebra and mathematics, and the training simply consists of showing the network a huge number of examples – not in itself a task requiring a lot of cognition.
An interesting side-effect of this is that very few human activities actually count as demonstrating intelligence at all, which does prove problematic.
I disagree. Just because we are intelligent, doesn’t mean intelligence is needed for everything we do.
Neural networks are lovely, aren’t they? In fact, it’s the very idea of how to use them and the intellectual work needed to get them to the state where they can be tuned is significant. The “intelligent” work here is how to get from linear algebra to a working and trainable AI. After the first breakthrough, subsequent work is less difficult, but there’s some real creative labor in figuring out what examples to give. For example, check out Google’s pictures of what their visual AI – probably the best on Earth – does to various images when it’s tuned to things that aren’t in them. It’s a great little lesson on how some excellent computer scientists think about the training process, and it’s more than just force-feeding the thing examples!
It’s fine to disagree on that point, and in fact I’m very sympathetic to the opinion, but it is a problem that needs a thorough solution. The traditional position is that intelligence is needed for many tasks outside of just assigning abstract classes, and throwing out the traditional position takes extra work. Otherwise, the argument remains vulnerable to the “but everyone knows” counterpoint, and that’s no good. (The “but everyone knows” counterpoint isn’t worth getting rid of, either, in part because it lets you defend typical use of words against people who insist on using them in strange ways.)
I didn’t mean to belittle the work people do on neural networks. But I think it is clear that e.g. AlphaGO’s skill does not stem from its programmers’ skill at playing GO. The systems are in fact capable of constructing its own, new abstractions to fit the problem.
And the efficacy of a sewing machine has nothing to do with its designer’s ability to do needlework. I’m afraid I must have put my points in a confusing manner for you to have interpreted “the ‘intelligent’ work here is how to get from linear algebra to a working and trainable AI” as having anything to do with skill at Go rather than skill at transforming the complexities of games into (relatively) complete machine solutions.
The machines, I’m afraid, do not construct abstractions. They are given abstractions and return abstractions according to an internal calculus, in the same way that mathematics does not construct abstractions but simply takes in abstractions and returns them. Humans understand the abstractions on both ends.
I don’t think Eliezer’s argument is common sense. It’s actually pretty contrarian. Common sense says that Einstein is substantially more intellectually capable than a mouse, a chimp, or a village idiot. I think I might agree.
“Amount of evolutionary work it took to generate the difference, number of neurons, measurable difference in brain structure” — these are all inputs, not outputs. So they might not be a good measure of intelligence — akin to trying to value a corporation based on its annual expenses.
As for the last one, performance on various tasks, I’d argue that Einstein is able to handily outperform a mouse, a chimp, and a village idiot on a broad range of tasks.
If this is true, how is Einstein able to do so much more with similar inputs? I’m not sure.
Maybe Einstein isn’t a genius due to hardware, he’s a genius due to software. If you strand Einstein on an island and he grows up as a feral child, he isn’t going to be able to invent relativity. The village idiot can’t run the latest software (he can’t do calculus for example) and thus the range of intellectual tasks he’s capable of performing is underwhelming.
Yes, much of the variance in intellectual output between humans seems to be explained by genes rather than environment. But that doesn’t mean software is unimportant. Consider a world where all the world’s software is open source, but some computers aren’t very good at running it. Most variance between computers will be accounted for by hardware, but it would be a mistake to conclude that we can just ignore software.
Instead of just thinking in terms of amount of evolutionary work, try thinking in terms of amount of computational work period. You could think of biological evolution as doing very slow computation to optimize the genome of an individual species. You could think of a human society as performing much faster computation, distributed among human brains, which develops intellectual tools like calculus. Maybe there is some crazy ratio like 100 yrs of human society computation=1 million years of evolutionary computation or something like that.
But why does this matter? We don’t care how Einstein got smart, we’re only comparing his intelligence to other things.
It could matter. This partly depends on what scientific information is available – the AI is allowed to do what you would do in its place.
«“Amount of evolutionary work it took to generate the difference, number of neurons, measurable difference in brain structure” — these are all inputs, not outputs.»
Sure, obviously that’s true. But it is also the case that metrics of outputs have their own problems, and some cannot be applied transversely, so measuring inputs is also important. This is why most companies demand employees to pass a card when they arrive and leave. Measuring inputs also helps you evaluating your subject. If an animal has only a couple of neurons, you can rule out any high intelligence. Likewise, if an employee never shows up to work, you can infer his outputs are nil as well.
I tentatively think you’re just wrong about how driving works. It takes training to be able to apply our ‘natural’ brainpower to the task. I suspect the worst adult human drivers can’t apply it consistently, for one reason or another.
(It also seems odd to say that the societal equivalent of Scaramucci’s tenure has been doing anything “for a while.” But it would still surprise me if it got stuck within the range of people who can actually apply their intelligence to the task.)
I would add my 0.25 cents. It is wrong to compare mice brain and Einstein’s brain, as most what makes Einstein = Einstein is not its hardware, but its training. In case of mice, there is no much training. I mean that if Einstein’s brain was born 10 000 ago he would not be able to create the relativity theory. Einstein is the product of the long evolution of billion’s of brains, which created language, physiscs and even invented Lorentz equation. So Einstein’s brain is equal to billion human brains and the gap between chimp and him is much wider, when it is typically presented by the EY’s graph.
Off-topic: Your comment about “my 0.25 cents” piqued my interest. Is this a phrase borrowed from another language/culture, or a joke about inflation/having an eighth as much to offer/etc.?
On-topic: Yes, training makes a big deal. I suspect animals are a lot closer to humans than we realize in terms of raw intelligence, they just can’t be trained nearly as well.
In fact, I copied my comment from the Facebook, where I replied to another comment, which started with “My 5 cents..” – so I was joking about commenting on a comment.
Current success with neural nets shows the importance of the large datasets, and humans have enormous dataset from their childhood. May be we just don’t have correct dataset to train animals. ))
So a general distinction about AI that’s being missed here: there are two ways we might think ai will improve suddenly at some point. First, maybe once we figure out how to do it we can just throw a bunch of extra hardware at the problem to cross from regular smart to super smart.
In practice, this would probably just give the regular (exponential) curve we see here for things like chess – we’re assuming at the point we get the algorithmic improvement we still have a bunch of boring tricks we can add. In practice, I suspect by the time we’re anywhere close to AGI we’ve already long since done all the easy tricks like adding hardware, so improvement will remain gradual.
But, there’s another method which doesn’t generalize, which is that agi might be able to improve itself. This has no analogy in the chess scenario (a computer good at playing chess isn’t good at programming a computer to play chess). It doesn’t have an equivalent anywhere short of agi. And that’s the version that could plausibly lead to a foom scenario.
Interestingly, it only leads to the food scenario once the ai is comparable in ability to top ai experts. So we’d have a gradual curve all the way up to “ai can match top human players”, and then a sudden jump to being a hundred times smarter over a day or two.
Thank you. Though there’s another issue: as I said elsewhere, people are talking about human-level AGI as if it hasn’t already passed – by assumption – the only visible barriers to absorbing a superhuman amount of knowledge.
One important thing that seems to be missing from this, is the huge difference in training between biological intelligence and artificial intelligence. At the moment AI can do really well, often better than humans, if tons of labeled samples exist. Once this training is done, we can just copy the coefficients to generate a clone of the AI. We can’t do that with humans. Children are not born knowing everything that there parents knew at conception. But on other hand, humans are much better at learning from a tiny set of examples. Show a human a single picture of a pangolin and he will be able to recognize pangolins in completely different pictures including drawings.
And on other issue is energy efficiency. The brain is extremely energy efficient, it uses around 20 watt and even that was only possible because it also enabled more efficient use of food via cooking. Current AI often uses large amounts of power, especially for training and CMOS semiconductor technology is close to its limits.
I think once you get to full mouse level intelligence it is like easy to scale that up to beyond human intelligence, however, we are far apart from that. Just because AI has started to become really useful on some tasks, that doesn’t mean that we have made much progress toward general AI as these specialized tasks still put constrains on the tasks that are very helpful for AI, but not all required for human intelligence.
On a technical note, EQ actually has a serious flaw – it uses brain size as a proxy for number of neurons, and those in turn as a proxy for the connectivity. There was a recent paper on this (http://www.pnas.org/content/113/26/7255.abstract) showing that even though smart birds like corvids have physically small brains, those brains are jam-packed with neurons, reaching primate-like total numbers.
As for the “smart at one thing, dumb at others”, this is basically the description of most “smart” non-mammals, especially if you also exclude birds. Crocodilians and monitor lizards are amazingly/terrifyingly intelligent in specific contexts, yet dunces in others. Using bait to catch food, understanding locking mechanisms (even if they lack the dexterity to manipulate them), anticipating prey behavior, coordinated pack hunting, etc., yet their communication abilities range from modest to minimal, and they’re largely uninterested in applying their minds to anything that doesn’t end with a meal or injuring a keeper. The same is likely true of cephalopods, though I don’t have direct experience with them like I do with crocodilians and other reptiles.
I’m also skeptical of the “Humans are so brilliant” angle, thought not in the stereotypical “but animals are smart too”, but rather the opposite. Even dealing with less intelligent animals than crocs and monitors, it’s amazing what can be accomplished with little more than a good memory, diverse stimuli, communication, and classical and operant conditioning. For all our vaunted intelligence, how much of what most humans do (or what we do on a day-to-day basis) really requires explanations beyond those factors, possibly with imitation thrown in? Are we *really* that smart, or are we just chimps with great memories and who are good enough at generalizing their operant conditioning to find rules which apply universally (i.e. rationality), then apply them all over the place until we get a banana / a program to compile / a Nature paper? I once heard it said that genius isn’t a matter of information stored or processing speed, but the ability to draw connections; can we think of Einstein as just a chimp who generalized the right rules in the right way at the right time to the right problem, a chimp could form more associations between rules and experiences than the others? We’ve created a system which amplifies our abilities through recorded knowledge, detailed communication, a special language for these universal rules (math), and which has positive feedback (new tools -> new info -> new discoveries -> new tools…), but in the absence of that community, amplification, & feedback, how smart are we? Are we only 5% smarter than an Austalopithecus, but that crucial 5% was the tipping point allowing us to create a feedback system? After all, we spent the vast majority of our species lifespan in a state only barely more advanced that modern chimp troops.
Perhaps it’s like flight. TONS of animals (and plant seeds) glide in some manner or another, and some can add power (just not enough). But once you cross the threshold into being capable of powered, steady, level flight (which has only happened 4 times out of the numerous gliders), the benefits are immense and a huge adaptive radiation follows. Archaeopteryx wasn’t that much worse, muscularly or aerodynamically, that some of the poorly-flying modern birds, but that last extra gram of muscle, that last 2% increase in lift:drag ratio makes all the difference. Perhaps we’re simply on the “winning”* side of that last inch?
*results pending.
You beat me to it, Cerastes :).
Brain size has only a loose correlation to neuron count, and neuron count correlates directly to information processing and data manipulation.
As far as AI goes, I’ve always believed the hockey stick jump in AI development as the inevitable conclusion:
You can give a machine the equivalent of a trillion neurons. It’ll take a while (and lots of programming) to get those “neurons” to function the way organic neurons do.. but when that is achieved, the AI will become super-intelligent (approximately 10x human) in relatively short order.. much faster than a human takes to develop. Electrical signals travel through our brain at about 200 miles per hour, or have about a 100 millisecond delay from signal activation to completion. In our hypothetical trillion “neuron” computer, using the same pathway lengths that exist in the human brain, that same signal would travel at about 175,000 mph or have a delay of 3 nanoseconds from activation to completion.
In a nutshell, it may take a human about 20 years to fully develop cognition, but computers operate about 3 millions times faster than humans.. so once the programming is complete, it would take a computer about 4 minutes to reach that same level of development, but with 10x the amount of neurons a human has.. or becomes “super intelligent.” The question I have is what happens in the next 4 minutes.. 😉
Wait, what?
The “short” period of time we are taking about here is longer than humans have existed as a species. And that’s not taking into account the engineering work to develop the preconditions, namely DNA, sexual selection, mammals, and the entire ecosystem that created the perfect game theoretic conditions where evolving intelligence makes sense.
I think there’s an assumption in there somewhere that evolution is massively inefficient and that more goal-directed human engineering can outperform it by orders of magnitude.
That assumption has proven true in certain domains. In other domains, not so much. It’s certainly one hell of an assumption to apply to intelligence.
On the other hand, if you decrease the time between generations by orders of magnitude you can accomplish that speedup without changing anything else at all.
What do you mean by “without changing anything else at all?” Presumably a human engineering effort to develop AI is not going to literally simulate the entire evolutionary history of the human species, and if one tried, it’d run orders of magnitude slower than the real thing, not faster.
Right. My point was, if you can iterate faster than 15 years or so, then the system evolves faster than humans do. Iterate once an hour and you’re evolving 130000 times as fast as humans, for example, plus whatever gains you realize from targeting mutations / faster mutation rate / stronger selection pressure / etc.
Yeah, but that assumes that your iteration process is as good at producing intelligence as human evolution was.
One way of looking at human evolution is “wow, so inefficient, new generation every 15 years, easy to outperform”.
Another way is “wow, the Earth is basically a giant super-computer simulating a complex evolutionary algorithm (DNA), competing / cooperating in a completely open-ended game (survival in a world with a fully-realized physics engine), and even with the computational resources of an entire planet, it still took millions of years to evolve human-level intelligence”.
In other words, in order to run an evolutionary simulation on human-created hardware that runs faster than the Earth-as-supercomputer, your simulation is going to need to be a lot simpler than the actual process that led to humans was, by an order of magnitude that has a lot of zeros attached to it. Maybe that’s possible, maybe that’s not. And even if it is possible, to actually implement it, you have to understand the evolution of human intelligence well enough to know which parts of the simulation you can safely discard.
Whenever I see debris in the street (empty plastic bag? raccoon carcass? fallen chunk of rock?), I wonder how a self-driving car would handle that situation.
@ Kaj Sotala
Rigidly following all speed limits seems a perilous strategy.
It is a great strategy if everyone else is also doing it. Its terrible if everyone else is doing 1.5x the speed limit.
Before I read on, wasn’t eliezer obviously talking about general intelligence?
I do need to say, the title of this post is fantastic 🙂
Part of the answer may be that the tasks you’re talking about (playing chess, driving cars) are not general goals achievable by a myriad of aims, but are specific constrained tasks that have been optimized for the human level.
Chess is a game that’s pretty well aimed at the human level. Mice can’t play it at all. You might be able to train chimps to not make illegal moves, but even that seems hard enough. Bad human players are close to as bad as you can be while still playing legal moves. And at the highest levels a lot of games are draws, so I think it’s quite plausible that the best human players aren’t *too far* away from ‘perfect’ play, which in chess means playing moves that (in the worst case) force a draw.
Cars and roads are likewise optimized for humans. Superhuman reflexes are helpful on the track, but they don’t buy you much driving around town; certainly not enough to make up for the occasional lapse in vision perception or whatever.
Basically the model I’m suggesting is that, in activities that are optimized for humans, there are often rapidly diminishing returns to performance above human level on specific subtasks, while subhuman performance can be very deleterious. That’s because the tasks are designed under the assumption that people will perform the component parts at around the human level, and so there’s no point in rewarding ability above this level, and you can safely assume human-level ability. Thus it’s not too surprising that narrow AIs progress slowly through human ability space.
Of course there are exceptions on other human tasks, particularly ones that reward particular subskills far above human level. I would imagine that a counter strike AI would progress rather rapidly from “running around in circles” to “instantly headshotting any human player” fairly quickly.
I don’t think this actually happens very often. It’s just that it is very noticeable when it does. I think there are far more things that fall out of that range. I think we often don’t even think about human and machine being substitutable for tasks where there is a clear winner, so we focus on the few cases where we can even think about substitution:
* information storage (humans have been outperformed by technology on this since the invention of writing)
* information retrieval (humans have been outperformed on this clearly since google and in some forms since indexing)
* arithmetic
* applying skills and information from one ad-hoc unstructured domain to another (humans still greatly outperform machines here)
* theory of mind (humans still outperform greatly)
* running a switchboard (very fast transition from human to machine winner)
* delivering a short message between cities (clearly machines have won this for a long time)
I could go on, but I think the vast majority of tasks have a clear winner between man and machine, with a few tasks that combine skills from both lists or which the technology is still developing falling in the middle. It’s really quite a short list of specific things where we can even have the man versus machine fight with a straight face.
We also redefine those tasks as the established use changes. For example running a switchboard also involved voice recognition, i.e. taking the request of who you want to be connected to. This was substituted with dialing systems. Which in a way are dumber but more machine-friendly. Only now we have voice recognition could enough that we could go back to the old operator-style calling.
Cars also can’t reproduce, horses can. We just didn’t value that part that much once industrialization allowed us to externalize the production. Hypothetically, if cars had been invented in medival times they would have been extravagant royal carriages crafted by hundreds of blacksmiths, clockmakers etc.
The commoners would still have used horses because they’re a lot easier to make. All you need is two horses.
Some of the tasks here strike me as a bit weird, because it’s hard to draw the line between a somehow distinct machine and a tool being operated by a human. It’s sort of like saying: “Machines have always been better at knocking fruit off of high branches than humans have been, because look, here’s a stick you can use to whack at it,” and then trying to use that to demonstrate machine intelligence and sophistication at solving some problems. Writing seems to fall under the same category, as does the human-driven car and arithmetic. The obvious distinction one can try to draw is between automatic and non-automatic tasks, where the switchboard and Google handle things automatically, but all of our “automatic” systems still have to have a human at the helm telling them when and how to get started. If we accept this task-comparison as a standard of thought, then it’s very hard to cordon off the computer and say “this, now this is machine intelligence, while everything else was just tools of varying efficiency!” Instead, the computer just looks like an extraordinarily sophisticated tool, so much so that it inspires thoughts of intelligence, but which doesn’t have any of the real meat of it. The computer can beat people at information storage, retrieval, arithmetic, and even chess, but all that just means that it’s a really, really good tool. After all, the stick can beat the human at some tasks.
The next problem is to try and figure out what intelligence is if it has nothing to do with tool-oriented tasks. Potentially there could be no such thing as intelligence, and instead we could find there are only tool-oriented tasks, but I’m personally suspicious of that claim.
Those gaps are quite different. The evolutionary work for example contains requirements for birth canal size and available daily energy intake. A too-powerful brain on a vegetable diet would simply starve animals to death.
AI as designed by humans on the other hand may scale quite different because human engineering often allows for dump stats. The system is optimized towards a few goals while others are just externalized. Neutral networks don’t need to reproduce. They don’t need to self-repair. They don’t need to supply themselves with constant energy, they can just be turned off and booted up again. Humans will supply everything they need to exist.
So if we measure difference in intelligence based on evolutionary steps it takes vs. how much hardware and advances in algorithms it takes then you’re getting different results. Do we have to factor in the billions spent on researching the latest semiconductor technology? And how do we account that most ANNs don’t even run on specialized ANN-hardware because the field is moving so fast that it rarely makes sense to build ASICs, especially for training instead of inference?
There are so many confounding factors in both chess progress and autonomous car progress. Autonomous cars are constrained by economics, we want them to run on cheap, embedded hardware and not on a supercomputer to beat the world’s best human driver. Chess computers on the other hand contain a lot of hand-crafted algorithms, something that is bottlenecked by humans writing task-specific code. That’s not generalizable intelligence. And even if you pair such hand-crafted rules with a hypothetical scalable ANN then the system may show more limited scaling behavior than whatever specific subtask the ANN is assigned to.
And this issue generalizes, most current ANN uses rely on manually designed scaffolding. And ANNs don’t even scale arbitrarily (yet). As hardware gets faster new research gets cranked out that shows what is necessary to structure those deeper networks for efficient training. Which means the structuring is still hand-crafted by humans for a specific scale level and for specific tasks.
So “progress” is measured by the combining many different inputs where most inputs will yield diminishing returns. And all practical implementations are cheating a lot by making some of those inputs irrelevant.
This is another confounding factor. Each human needs to be trained from a fairly low level (some batteries included). On the other hand ANNs can be copied. Once a single copy has been improved in a development setting it can be rolled out to millions of cars and the whole fleet will improve. That’s like everyone getting Einstein brain transplants.
It seems like a lot of your intelligence touchstones are binary. You can either do them or you can’t.
You can come up with dummy metrics that sum the number of tasks something is able to do, but that doesn’t tell you how “close” two groups are in intelligence.
There are some tasks that operate on a sliding scale. At what elo can you play chess, etc. But what we really want to know is whether leaps in intelligence translate into being able to do new tasks no one can really do yet. For example it is easy to imagine that we develop chess AI to 9001 elo but it the same AI recipe still sucks at driving.
Human chess-playing ability runs from “has never seen a chessboard before” to “grandmaster”. By definition, any progress up until you surpass human grandmasters falls somewhere within this range – even the decades where computers couldn’t play chess at all technically fell within the human range of chess-playing ability.
I’m not sure about the villiage idiot, but grandmaster level is about where people start playing professionally.
Chess computers went from barely being able to compete in state tournaments (Chess 4.5, ’76) to master level (Cray Blitz in ’81, Belle in ’82) in five or six years, then to grandmaster level (HiTech and Deep Thought, ’88) in six or seven years. By 96-97, Deep Blue was about on par with the world champion, so that’s another decade. At some point over the decade after that, it became clear that the best chess computers were strictly superhuman; it was now impressive for a world champion to merely draw with one, and even that became more and more fleeting over time. And now, two decades after Deep Blue, the idea that a human could beat the best chess computers is laughable.
That’s about 8-15 years from “chess computers are as good as human professionals” to “chess computers are better than every human on earth”. Does that really sound like a wide band of human exceptionalism?
Depends how impressive you think being a professional in a niche field is. If a hypothetical baseball-playing robot went from AA to World Series MVP in 20 years, I wouldn’t find that nearly as impressive as being able to make one as good as a AA ballplayer in the first place.
If we’re trying to compare something to humans generally, using the average human is probably wisest. The average human can be okay at chess, but they’ll never be a grandmaster, even with intense training – chess AIs were “superhuman” in the 70s, if you’re talking about normal humans and not those with freakish chess skillz.
That’s rather the point, is it not?
Alternate theory: Human individual variation in intelligence is large enough that it overlaps the average value of other species. A troop of chimps can survive in a jungle, a troop of village idiots left to their own devices will probably starve in a month.
Conversely, this theory suggests that there are some exceptionally intelligent chimps who approach average human intelligence. Maybe not quite, because it’s harder to improve something rather than break it, but they might perhaps get within a few standard deviations from it. This would explain the occasional chimp who invents new tools or manages to learn sign language.
I wouldn’t bet on a troop of Einsteins, or equivalent, to do much better than our village idiots. In fact, i wouldn’t bet against them doing worse. Intelligence is all well and good, but knowledge is probably equally, if not more so, important.
I can’t help but think of this line: “The crucial cognitive structures of the mind are working memory, a bottleneck that is fixed, limited and easily overloaded, and long-term memory, a storehouse that is almost unlimited.”
Pragmatic Education
If our reasoning powers are dominated by one or two bottlenecks, then widening those bottlenecks could have a huge effect on our reasoning powers, without requiring much change to the rest of the brain. So people with brains that were in most respects highly similar could vary greatly in their reasoning powers, just because of a better working memory.
Of course, an AI could be built with however large a working memory you liked. It would be relatively easy to improve any specific bottleneck with the next version.
“But that doesn’t address the fundamental disparity: isn’t the difference between a mouse and Joe Average still immeasurably greater than the difference between Joe Average and Einstein?”
Not necessarily. I’d say it’s just immeasurable. The fact that you put these things on a number line does not make it sensible to put them on a number line.
How well did Einstein drive?
… TIL.
If an AI was coming to get me, I would just spritz it with some orange juice and be done with it.
Seriously, though, I don’t see how it’s reasonable to think of any AI as “mouse-like”, or almost chimp-like (in some decades), or almost almost human-like (in some more decades). What AI has ever done anything with intent? What AI has ever been self-sufficient?
The fact that they can perform a couple tasks better than some humans is a trivial feat. Rocks are super good at sitting still. Flies are super good at smelling things. Should I be afraid of rocks and flies?
If someone told me, “Over the last 50 years flies have become exponentially better at smelling XYZ,” I would reply, “Huh…curious.” If someone then told me, “Humans engineered them to do it!” I would reply, “Oh…less curious.”
And yet, the fly is more threatening than the AI. The fly is a hearty thing; it can replicate and evolve according to the pressures of its environment. Spritz it with orange juice and it gets super psyched!
I might be inclined to find the AI threatening if it could act with intention, self-sustain, and replicate. The AI would be decidedly scarier than the rock, if it could do these things.
Anyway, I don’t mean for this comment to be disrespectful — killer blog, first time participating here! — but I wanted to inject into the discussion a little appreciation for what it takes to make it in this universe.
I’ll be sure to take your advice if the US government ever sends an autonomous weapon after me. 😛
I think you’re confusing hardware with software here.
The average supercomputer has hardware that’s highly specialized and nonthreatening (unless the hardware has some reason to be made threatening.) Far less dangerous than even a fly’s body… well, unless a battery explodes.
But the supercomputer’s software is already much more dangerous and useful than a fly’s mind, which can seek food and halfheartedly avoid flyswatters and that’s about it. It can be turned to a far wider variety of tasks than any fly – it can predict the weather, analyse language, play the stock market, trawl through databases to identify persons of interest or target demographics for an ad campaign … still not that dangerous except in the wrong circumstances, though.
The hardware probably isn’t going to get much more dangerous, at least not for cutting-edge AI. They’re always going to be in massive server farms. But the software is going to get more dangerous over time; maybe even comparable to, or more dangerous than, the human mind.
And once it’s acting with well-reasoned intentions, becoming self-sustaining and self-replicating (humans already know how to make computers) isn’t far behind.
There’s a potential equivocation here between classifying AIs as dangerous agents and classifying them as dangerous tools. There’s a fantastic case to be made for our current, non-general AIs being very dangerous tools in the wrong hands. It’s a much weaker case to try and paint them as dangerous agents.
I would just be wary of that with your argument.
This is very misleading. You make it sound like these were random children, but in fact they were dan-level players (https://en.wikipedia.org/wiki/Computer_Go#History) who had been studying Go seriously for years. Similarly, in Go (and most things?), the gap between “random professional” and “world champion” is much narrower than the gap between “random professional” and “random human” (or even “amateur human”); perhaps a few hundred elo points compared to a few thousand elo points (https://en.wikipedia.org/wiki/Go_ranks_and_ratings).
In the first paragraph, you use “easy” to refer to millions of years of optimization by evolution. In the second, you use “a lot of work” to refer to decades of work by small teams of humans. Maybe the variation/work-required-to-reproduce-that-variation between humans is small relative to the scale that evolution operates on, but large relative to the scale that humans operate on.
The obvious rejoinder is that mice and chimps seem to be closer to bad humans than bad humans are to world champions on many tasks…but I’m not sure that’s actually true. Has a chimp ever played a game of Chess or Go? Or learned a language? Or competed seriously with a below-average human on any intelligence task?
Maybe Eliezer’s picture is more-or-less accurate, but the scale is just much vaster than we think.
In the past, nature (or what have you) has sometimes selected for better intelligence. I’m curious how the author believes this selection may take place in the future, if ever.
EDIT: Moved this comment here because this is really where it belongs, not on the Open Thread.
There’s an interesting little anecdote over at this blog (run by one of our commenters) and if it’s accurate as to the state of play in machine recognition, then my level of scepticism about AI threat has been racheted up another couple of notches.
Because this is not intelligence in any form, this is about as intelligent as a photocopier. It’s not recognising the image of a panda because, unlike a human who has general models for recognising “panda” and “gibbon” and so could identify the second image as a panda even if they had never seen the first image, the machine plainly isn’t doing this.
It’s not abstracting out a platonic form, if you will, of “panda” from all the images of pandas shown to it; it’s doing the same thing as a photocopier reproducing what is put on the platen to be copied: scanning the image and keeping it in memory, with a lot of other scanned images, to compare with a new image shown to it. So of course it’s being thrown off by the coloured pixels: the first image has no coloured pixels, so it’s a different image. Why it comes out with “gibbon” – well, maybe the images of gibbons it has been trained on had similar colours to the pixelation, so it’s making the ‘best guess’.
It’s Chinese Room activity at its finest, and if Searle’s thought-experiment was meant to show you don’t need consciousness, I think it shows that machine “intelligence” is anything but intelligence as we define it.
And that’s why I’m sceptical of AI threat qua “independent actor with its own goals”; I think it highly unlikely we’ll get that. What we will get are Big Dumb Machines that work by being so much faster in processing and having so much more memory and having so much more speed of recall and ability to brute-force pattern match than humans. And we’ll fool ourselves that Alexa and Cortana are really intelligent (because we so badly want them to be intelligent), and we’ll use them to make recommendations and base policy and governing and economic decisions on those recommendations, and then we’ll go running off a cliff and splat at the bottom because these are not conscious or intelligent entities that we have entrusted with so much power, they’re Huge Stupid Fast Photocopiers.
Maybe this isn’t the latest word in machine learning or neural networks or what have you, but if it’s anyway recent I think the worry about “soon mouse-level, then human-level, and the four minutes after that superhuman and beyond!” is very much misplaced; human-level or anything like it looks a long, long way away (“human-level” as in “can recognise, by identifying the notes and checking them against pieces held in memory, that the notes of a piece of music being played are dah-dah-dah-dum that’s A major etc etc etc okay that’s the Mendelssohn Fourth” and not “pattern-match snippet of music to other snippets in memory and if it isn’t identical on an oscilloscope, or however the heck the thing works, to one particular recording that it’s sampled then it’s completely lost as to what it is”).
This does not follow. Neural-net based recognition does build a general model. It has to, it cannot work this way:
The models simply aren’t big enough to work this way. But just because you’ve trained a model to recognize things by features doesn’t mean you’ve picked the correct features; if all the pictures of pandas in the training set are strictly black and white, maybe the model over-weights the lack of color in the picture.
I would submit that if you are training your model to recognise features of “black” and “white” for pandas, and not “has round ears on the top of its head, a snout, and claws” then you are not alone training it on the wrong features, you are not training it at all. If the “model” the neural net is creating is “the salient features of an image of a panda are that the image be in black and white, not colour”, then it is so far from anything that can reasonably be called human intelligence, it’s not intelligent in any meaningful form at all. It is not building a general model of what a panda is, it is building a model of “images of pandas are always in black and white, so an image in black and white is a panda, an image with colour is not a panda”, without any idea in the world what a “panda” is.
But if it works to sort out ten (black and white) images of a panda from fifty images of various animals, then we tell ourselves “Aha, we’ve taught it to recognise a panda!” when we have done nothing of the kind, and even more erroneously “Aha, now it knows what a panda is!”. Throwing in a few colour images of pandas isn’t any kind of correction for the underlying problem; now all the machine has ‘learned’ is that some images are in colour as well. It still has no model for “panda”, so give it a line art image and it’ll be stumped. And it certainly has not learned “a panda is an animal belonging to the bear family and can be identified by these anatomical features”, which even small human children can learn.
Deiseach, if I’m correctly interpreting the conclusions you’ve drawn, I can say with confidence that you’re really misunderstanding. Machine learning systems can absolutely recognize pandas in a fairly general way, not just panda photos it’s already seen, and without any gimmes like the panda images being exactly the black and white ones. I think I see where you went wrong — the paper that’s linked from plover.com is publication-worthy precisely because it’s a very surprising result. They did a systematic search for changes that would cause the system to fail, and then systematically sought out the minimal version to cause failure — that’s what they mean when they say it’s an adversarial example. It’s not at all typical for such a small change to cause failure that way. This is the equivalent of an optical illusion — it’s just that what works as an optical illusion for that system isn’t at all the same as what would work for a human, and so to us it seems ridiculous that the example in question would cause failure.
This is made more confusing by the fact that the other example cited by plover.com, Shazam, really is a very dumb, photocopier-like program, with no machine learning involved at all as far as I know — it looks for a very specific fingerprint associated with a very specific recording, and can’t generalize at all (although it does some smart things with compensating for noise, different sound systems, and volume variation).
Machine learning has actually gotten as good as humans at recognizing the subject of a photo, possibly even better.
Does that clear things up? It’s possible I’m misunderstanding what conclusions you’ve drawn, in which case never mind ;).
It is rude to call Shazam a “very dumb, photocopier-like program”. Containing machine learned is not the only way that a program can be not dumb. The ideas behind Shazam are actually quite subtle, and are far beyond what current deep learning would come up with just given raw data. There is a recent tendency to disregard anything that is not “machine learning”, and I think your post is a good example of this.
I think Deiseach is mostly right in her doubts about how Pandas are being recognized. If the program can be tricked in certain ways, it strongly suggests that it is failing to have the same kind of understanding that we have. If machine learning sees patterns of black and yellow bars as a school bus, then it is missing something. It is not acceptable to claim that the program is right, and that we are mistaken. Current recognition rates are impressive, but the evidence is that they are not looking at the same kinds of things that we are looking at. This mismatch will hurt them later.
I’m certainly not intending to be rude to Shazam. I’m a software developer with some experience in both music and signal processing, and I’d be extremely proud if I’d conceived and implemented Shazam. I only mean that it’s dumb in the precise, “photocopier-like” way that Deiseach mentions: it’s looking for the fingerprint of a specific recording, not generalizing in any way over the musical qualities of a composition. And in this way, it’s extremely different from how eg image recognition works, which does generalize over characteristics of the image, rather than looking for the fingerprint of a particular file.
Deep learning works on the premiss that it is extracting some features, and matching against those features. Shazam works in the same way, the only difference is that they are explicit about how they extract features, rather than having a learning algorithm extract them. Choosing to move everything to frequency space, and to select just the loudest signals is one way of selecting features. Counting the number of features that overlap is another way of comparing features. A machine learned system might, in a similar way, extract a set of archetypes, and determine a set of weights for each archetype. Of course, machine learned models are not usually described in terms of archetypes, but they can be thought of in that way, especially when they can be used generatively.
A machine learning algorithm is generalizing when it is used in recognition, it is looking for features that it has previously chosen. I suppose in future, we might have networks that carry out learning the instance to be classified, and then use those second level weights as input to a previously learned classifier. I have not seen this done, probably because it would be equivalent to something simpler, or because it makes no sense.
They’re certainly different. It may just be a matter of semantics; to me they seem importantly different, and to you they seem unimportantly different. I’m happy to leave it there; it wasn’t the main point I was trying to make anyhow.
The chess and go data seem awfully noisy, and I suspect that they conflate two different AI modalities: pre-neural machine learning and post-neural machine learning. It’s entirely possible that they were valid for most of the expert system/rule-based/deep-cutoff era of AI, but deep learning techniques are likely to improve super-linearly–especially in well-constrained domains of knowledge and performance. And the deep learning stuff is only about a decade old. If you were to plot the deep learning systems, they go from “plays go worse than a planarium” to “beats Lee Sedol” in an alarmingly short amount of time.
Computers playing chess and playing Go were not solved by creating a general intelligence.
I can beat the transistors off Deep Blue and AlphaGo- at Go and Chess, respectively.
Making a computer that can play Go and Chess is possible- and I can beat it at Tic-Tac-Toe, or Monopoly, or Iterated Prisoner’s Dilemma, or some other game that it hasn’t been designed for.
When you can design a computer that learns the rules and victory conditions of arbitrary games and it can occasionally get in the money at poker tournaments, then I will believe that computers might be approaching human-level at the skill of ‘game playing’, and are a force imminently to be watched in the field of software design.
You might be interested to know that neural networks are now able to generalize at least to learning the rules & victory conditions of arbitrary Atari games, even the same instance of the neural network learning multiple games in a row. Also that a deep reinforcement learning system beats professionals at no limit heads-up poker.
And neither of those networks is general purpose.
I would bet a thousand dollars to a hundred that neither of those learning networks could regularly beat me at Monopoly, and even money that neither would be any good at gin rummy, spades, or Go.
/General purpose/ is a very hard thing to do.
You might be interested in General Game Playing, where programs first are given the rules of the game, and then play. I don’t know how good the current crop is, but they used to be able to beat me at certain games.
Are they able to beat competent humans at Chess, Go, or even checkers?
The problem with all of this speculation, is that we don’t have any idea how scaleable intelligence is outside of its observed range. Perhaps its huge but it also might be quite incremental. Most growth curves follow a sigmoid pattern and we could easily be anywhere on that curve (we could even be at the top of it). If we’re near the bottom or middle, then AI may overtake us in milliseconds if we’re near the top, it may be a lot of work just to bring AI level with us. That’s assuming we use a human-like intelligence architecture. If we use a different one to ours, it’s growth curve could look very different and top out either way above or below ours on general intelligence tasks.
For instance someone mentioned working memory as a bottleneck. This is certainly true in explaining within human difference, but we don’t have a good sense of how large gains it would produce outside of the observed range. For instance, 64 bit processors have significant advances over 32 bit processors, but most people seem to think that a 128 bit processor would not confer many practical advantages. Similarly, we presumably are somewhat constrained by the physical brain sizes we can fit through the birth canal, but we don’t actually know how much extra advantage you would get with a doubling. Obviously having integrated non-neuronal technology integrated into artificial brains would confer an advantage, but again it’s hard to know in advance how much of an advantage that will be compared with humans who have access to those tools (either as currently using computer interfaces) or a more direct brain interface.
Eh, the answer is sociality, and to some extent play. You’ve got to have complex things that are trying to figure out (and sometimes fool) other complex things, to have intelligence. A new person is like a whole game unto themselves; “learning” a new person is like learning a whole new game, like Chess, on the fly. So you multiply that by all the pairwise interactions in an ancestral environment tribe (so learning a bunch of people is like learning Chess1, Chess2, etc.) in terms of roughly the numbers of such a tribe (what’s the memory number, like 20 people or something?), then you might have something like actual intelligence popping up.
That’s really what you’ve got to get an AI to do, in order to be anything other than an idiot savant with a bloated specialization in some particular form of pattern recognition. Intelligence is more than pattern recognition or learning, it’s also negotiation, which involves play and modelling, not just of the “opponent” but also of the world you’re both embedded in.
Re. the Mouse to Joe/Einstein thing, why not look at it the other way round? Maybe the reason why the diff. between Joe and Einstein seems great is because it really is great, and the measurement that’s telling us that it isn’t is using the wrong parameters.
Here’s a different challenge: what would it take for a computer program to invent a game that people want to play?
I realize this is vague. How many people? How long would the game need to remain popular to count? Maybe it would be enough if people organize tournaments for the new game.
I’m actually pretty inclined to believe your Theory 2: Purpose Built Hardware provides most or all of the answer. It’s exactly as you say, humans have custom “hardware” (wetware?) to handle complex things like spatial perception, proprioception, navigation, etc. Self driving cars have some overlap with our skills, in regards to object detection and physics prediction, and to the degree that it’s optimized for just those things, it performs as well or better than we do. There are still algorithms we have in our repertoire that give us edges in other ways, such as the ability to ascribe agency to other drivers and project likely behaviors onto them, or first-hand knowledge of how varying conditions affect friction, visibility, etc. These aren’t free, these are acquired from a lifetime of learning first to control our bodies as infants, and then a succession of experiences that shape our heuristic into a general world model. By the time we come of age and go through driver training, that model includes tons of “extras” like “some people are jerks” and “ice is slippery” that, while not learned for the purpose of driving, can nonetheless apply in that domain.
So I guess it would be wrong to say that it’s custom hardware so much as custom training. The AI that steer your self-driving cars have many benefits in that regard, such as being able to share the heuristic knowledge between multiple AIs and cut out a lot of the re-learning process for each successive generation. But it’s still lacking in some basic training that humans get by virtue of growing up around other humans, and interacting with them in lots of other ways long before we ever start driving. I suspect the “secret sauce” that is missing from allowing self-driving cars to truly surpass even the most skilled human driver lies in some of those common experiences of being human that we take for granted to a degree that we fail to recognize it is even a learned skill.
What I think will prove interesting over time is the ways in which autonomous vehicles behavior over time will adapt as society reaches a critical mass of self-driving-vehicle adoption. I.e. at some point “understanding how other human motivations work and how it affects their cars on the road” will not be a relevant heuristic, and instead the vehicles will be learning from the behaviors of other AI (and/or from the networked internal communication between cars). I suspect what “traffic” looks like at that point will be something distinctly different from today, and more organic in structure.
In any case, self-driving-car Utopia can’t get here soon enough, as far as I’m concerned. I’m tired of dealing with all the worst parts of humanity vis-a-vis traffic on a daily basis during my commute. I, for one, welcome our new robotic car overlords.
Imo this discussion is missing the most important aspect, energy efficiency.
Human brains are ridiculously efficient compared to our hardware. It’s why I’m not worried about AI.
The size of smallest transistors (2D structures or 2.5D at best) is about 150nm, all things considered, especially spacing between transistors (this not the same thing as process size, which refers the smallest ‘mark’ you can make).
To contrast, the diameter of a ribosome – a full-fledged molecular factory – is 20nm. I think it’s a good illustration of how much silicon hardware sucks compared to biology – you can only do so much with software.
The more hypothetical part – which I find plausible – is how animal thinking works. Cell insides are small enough that the Second Law of Thermodynamics – a statistical law – no longer applies directly, but only on average. Operating on that scale allows reversible computing – which would sort-of explain how sudden insight works – you are not aware of how did you figure a solution to something because information about computation process is gone – reversed, leaving only the answer + some unavoidable heat waste.
If the part about reversible computation is true, there’s ZERO AI risk with both current and foreseeable hardware – trying to emulate reversible computation with a non-reversible one leads to an exponential blowup in energy usage. It could well be that a non-reversible human brain would use as much energy as a large city.
That doesn’t mean I’m skeptical about AI in general – it could well be that ~99% of human jobs are reproducible and simple enough they can be done even with an inferior silicon hardware.
As noted, it appears that human intelligence seems to filter basically everything abstract through language processing, spatial modeling, and heuristics. That we can manage systems of any complexity through this method is quite remarkable. It will be interesting to see how a “general intelligence” AI will develop, whether it will work in layers of increasing abstraction like our brains do, or if it will have a separate capacity to design and access custom-built direct-access algorithmic functions like Alpha Go does. An intelligence like that would be quite remarkable, and quite alien.