[Epistemic status: very uncertain. Not to be taken as medical advice. Talk to your doctor before deciding whether or not to get any tests.]
I.
There are many antidepressants in common use. With a few exceptions, none are globally better than any others. The conventional wisdom says patients should keep trying antidepressants until they find one that works for them. If we knew beforehand which antidepressants would work for which patients, it would save everyone a lot of time, money, and misery. This is the allure of pharmacogenomics, the new field of genetically-guided medication prescription.
Everybody has various different types of cytochrome enzymes which metabolize medication. Some of them play major roles in metabolizing antidepressants; usually it’s really complicated and several different enzymes can affect the same antidepressant at different stages. But sometimes one or another dominates; for example, Prozac is mostly metabolized by one enzyme called CYP2D6, and Zoloft is mostly metabolized by a different enzyme called CYP2C19.
Suppose (say the pharmacogenomicists) that my individual genetics code for a normal CYP2D6, but a hyperactive CYP2C19 that works ten times faster than usual. Then maybe Prozac would work normally for me, but every drop of Zoloft would get shredded by my enzymes before it can even get to my brain. A genetic test could tell my psychiatrist this, and then she would know to give me Prozac and not Zoloft. Some tests like this are already commercially available. Preliminary results look encouraging. As always, the key words are “preliminary” and “look”, and did I mention that these results were mostly produced by pharma companies pushing their products?
But let me dream for a just a second. There’s been this uneasy tension in psychopharmacology. Clinical psychiatrists give their patients antidepressants and see them get better. Then research psychiatrists do studies and show that antidepressant effect sizes are so small as to be practically unnoticeable. The clinicians say “Something must be wrong with your studies, we see our patients on antidepressants get much better all the time”. The researchers counter with “The plural of anecdote isn’t ‘data’, your intuitions deceive you, antidepressant effects are almost imperceptibly weak.” At this point we prescribe antidepressants anyway, because – what else are you going to do when someone comes into your office in tears and begs for help? – but we feel kind of bad about it.
Pharmacogenomics offers a way out of this conundrum. Suppose half of the time patients get antidepressants, their enzymes shred the medicine before it can even get to the brain, and there’s no effect. In the other half, the patients have normal enzymes, the medications reach the brain, and the patient gets better. Researchers would average together all these patients and conclude “Antidepressants have an effect, but on average it’s very small”. Clinicians would keep the patients who get good effects, keep switching drugs for the patients who get bad effects until they find something that works, and say “Eventually, most of my patients seem to have good effects from antidepressants”.
There’s a little bit of support for this in studies. STAR*D found that only 33% of patients improved on their first antidepressant, but that if you kept changing antidepressants, about 66% of patients would eventually find one that helped them improve. Gueorguieva & Mallinckrodt (2011) find something similar by modelling “growth trajectories” of antidepressants in previous studies. If it were true, it would be a big relief for everybody.
It might also mean that pharmacogenomic testing would solve the whole problem forever and lets everyone be on an antidepressant that works well for them. Such is the dream.
But pharmacogenomics still very young. And due to a complicated series of legal loopholes, it isn’t regulated by the FDA. I’m mostly in favor of more things avoiding FDA regulation, but it means the rest of us have to be much more vigilant.
A few days ago I got to talk to a representative of the company that makes GeneSight, the biggest name in pharmacogenomic testing. They sell a $2000 test which analyzes seven genes, then produces a report on which psychotropic medications you might do best or worst on. It’s exactly the sort of thing that would be great if it worked – so let’s look at it in more depth.
II.
GeneSight tests seven genes. Five are cytochrome enzymes like the ones discussed above. The other two are HTR2A, a serotonin receptor, and SLC6A4, a serotonin transporter. These are obvious and reasonable targets if you’re worried about serotonergic drugs. But is there evidence that they predict medication response?
GeneSight looks at the rs6313 SNP in HTR2A, which they say determines “side effects”. I think they’re thinking of Murphy et al (2003), who found that patients with the (C,C) genotype had worse side effects on Paxil. The study followed 122 patients on Paxil, of whom 41 were (C,C) and 81 were something else. 46% of the (C,C) patients hated Paxil so much they stopped taking it, compared to only 16% of the others (p = 0.001). There was no similar effect on a nonserotonergic drug, Remeron. This study is interesting, but it’s small and it’s never been replicated. The closest thing to replication is this study which focused on nausea, the most common Paxil side effect; it found the gene had no effect. This study looked at Prozac and found that the gene didn’t affect Prozac response, but it didn’t look at side effects and didn’t explain how it handled dropouts from the study. I am really surprised they’re including a gene here based on a small study from fifteen years ago that was never replicated.
They also look at SLC6A4, specifically the difference between the “long” versus “short” allele. This has been studied ad nauseum – which isn’t to say anyone has come to any conclusions. According to Fabbri, Di Girolamo, & Serretti, there are 25 studies saying the long allele of the gene is better, 9 studies saying the short allele is better, and 20 studies showing no difference. Two meta-analyses (1 n = 1435, 2 n = 5479) come out in favor of the long allele; two others (1 n = 4309, 2, n = 1914) fail to find any effect. But even the people who find the effect admit it’s pretty small – the Italian group estimates 3.2%. This would both explain why so many people miss it, and relieve us of the burden of caring about it at all.
The Carlat Report has a conspiracy theory that GeneSight really only uses the liver enzyme genes, but they add in a few serotonin-related genes so they can look cool; presumably there’s more of a “wow” factor in directly understanding the target receptors in the brain than in mucking around with liver enzymes. I like this theory. Certainly the results on both these genes are small enough and weak enough that it would be weird to make a commercial test out of them. The liver enzymes seem to be where it’s at. Let’s move on to those.
The Italian group that did the pharmacogenomics review mentioned above are not sanguine about liver enzymes. They write (as of 2012, presumably based on Genetic Polymorphisms Of Cytochrome P450 Enzymes And Antidepressant Metabolism“>this previous review):
Available data do not support a correlation between antidepressant plasma levels and response for most antidepressants (with the exception of TCAs) and this is probably linked to the lack of association between response and CYP450 genetic polymorphisms found by the most part of previous studies. In all facts, the first CYP2D6 and CYP2C19 genotyping test (AmpliChip) approved by the Food and Drug Administration has not been recommended by guidelines because of lack of evidence linking this test to clinical outcomes and cost-effectiveness studies.
What does it even mean to say that there’s no relationship between SSRI plasma level and therapeutic effect? Doesn’t the drug only work when it’s in your body? And shouldn’t the amount in your body determine the effective dose? The only people I’ve found who even begin to answer this question are Papakostas & Fava, who say that there are complicated individual factors determining how much SSRI makes it from the plasma to the CNS, and how much of it binds to the serotonin transporter versus other stuff. This would be a lot more reassuring if amount of SSRI bound to the serotonin transporter correlated with clinical effects, which studies seem very uncertain about. I’m not really sure how to fit this together with SSRIs having a dose-dependent effect, and I worry that somebody must be very confused. But taking all of this at face value, it doesn’t really look good for using cytochrome enzymes predicting response.
I talked to the GeneSight rep about this, and he agreed; their internal tests don’t show strong effects for any of the candidate genes alone, because they all interact with each other in complicated ways. It’s only when you look at all of them together, using the proprietary algorithm based off of their proprietary panel, that everything starts to come together.
This is possible, but given the poor results of everyone else in the field I think we should take it with a grain of salt.
III.
We might also want to zoom out and take a broader picture: should we expect these genes to matter?
It’s much easier to find the total effect of genetics than it is to find the effect of any individual gene; this is the principle behind twin studies and GCTAs. Tansey et al do a GCTA on antidepressant response and find that all the genetic variants tested, combined, explain 42% of individual differences in antidepressant response. Their methodology allowed them to break it down chromosome-by-chromosome, and they found that genetic effects were pretty evenly distributed across chromosomes, with longer chromosomes counting more. This is consistent with massively polygenic structure where there are hundreds of thousands of genes, each of small effects – much like height or IQ. But typically even the strongest IQ or height genes only explain about 1% of the variance. So an antidepressant response test containing only seven genes isn’t likely to do very much even if those genes are correctly chosen and well-understood.
SLC6A4 is a great example of this. It’s on chromosome 17. According to Tansey, chromosome 17 explains less than 1% of variance in antidepressant effect. So unless Tansey is very wrong, SLC6A4 must also explain less than 1% of the variance, which means it’s clinically useless. The other six genes on the test aren’t looking great either.
Does this mean that the GeneSight panel must be useless? I’m not sure. For one thing, the genetic structure of which antidepressant you respond to might be different from the structure of antidepressant response generally (though the study found similar structures to any-antidepressant response and SSRI-only response). For another, for complicated reasons sometimes exploiting variance is easier than predicting variance; I don’t understand this enough to be sure that this isn’t one of these cases, though it doesn’t look that way to me.
I don’t think this is a knock-down argument against anything. But I think it means we should take any claims that a seven (or ten, or fifty) gene panel can predict very much with another grain of salt.
IV.
But assuming that there are relatively few genes, and we figure out what they are, then we’re basically good, right? Wrong.
Warfarin is a drug used to prevent blood clots. It’s notorious among doctors for being finicky, confusing, difficult to dose, and making people to bleed to death if you get it wrong. This made it a very promising candidate for pharmacogenomics: what if we could predict everyone’s individualized optimal warfarin dose and take out the guesswork?
Early efforts showed promise. Much of the variability was traced to two genes, VKORC1 and CYP2C9. Companies created pharmacogenomic panels that could predict warfarin levels pretty well based off of those genes. Doctors were urged to set warfarin doses based on the results. Some initial studies looked positive. Caraco et al and Primohamed et al both found in randomized controlled trials with decent sample sizes that warfarin patients did better on the genetically-guided algorithm, p < 0.001. A 2014 meta-analysis looked at nine studies of the algorithm, over 2812 patients, and found that it didn’t work. Whether you used the genetic test or not didn’t affect number of blood clots, percent chance of having your blood within normal clotting parameters, or likelihood of major bleeding. There wasn’t even a marginally significant trend. Another 2015 meta-analysis found the same thing. Confusingly, a Chinese group did a third meta-analysis that did find advantages in some areas, but Chinese studies tend to use shady research practices, and besides, it’s two to one.
UpToDate, the canonical medical evidence aggregation site for doctors, concludes:
We suggest not using pharmacogenomic testing (ie, genotyping for polymorphisms that affect metabolism of warfarin and vitamin K-dependent coagulation factors) to guide initial dosing of the vitamin K antagonists (VKAs). Two meta-analyses of randomized trials (both involving approximately 3000 patients) found that dosing incorporating hepatic cytochrome P-450 2C9 (CYP2C9) or vitamin K epoxide reductase complex (VKORC1) genotype did not reduce rates of bleeding or thromboembolism.
I mention this to add another grain of salt. Warfarin is the perfect candidate for pharmacogenomics. It’s got a lot of really complicated interpersonal variation that often leads to disaster. We know this is due to only a few genes, and we know exactly which genes they are. We understand pretty much every aspect of its chemistry perfectly. Preliminary studies showed amazing effects.
And yet pharmacogenomic testing for warfarin basically doesn’t work. There are a few special cases where it can be helpful, and I think the guidelines say something like “if you have your patient’s genotype already for some reason, you might as well use it”. But overall the promise has failed to pan out.
Antidepressants are in a worse place than warfarin. We have only a vague idea how they work, only a vague idea what genes are involved, and plasma levels don’t even consistently correlate with function. It would be very strange if antidepressant testing worked where warfarin testing failed. But, of course, it’s not impossible, so let’s keep our grains of salt and keep going.
V.
Why didn’t the warfarin pharmacogenomics work? They had the genes right, didn’t they?
I’m not too sure what’s going on, but maybe it just didn’t work better than doctors titrating the dose the old-fashioned way. Warfarin is a blood thinner. You can take blood and check how thin it is, usually measured with a number called INR. Most warfarin users are aiming for an INR between 2 and 3. So suppose (to oversimplify) you give your patient a dose of 3 mg, and find that the INR is 1.7. It seems like maybe the patient needs a little more warfarin, so you increase the dose to 4 mg. You take the INR later and it’s 2.3, so you declare victory and move on.
Maybe if you had a high-tech genetic test you could read the microscopic letters of the code of life itself, run the results through a supercomputer, and determine from the outset that 4 mg was the optimal dose. But all it would do is save you a little time.
There’s something similar going on with depression. Starting dose of Prozac is supposedly 20 mg, but I sometimes start it as low as 10 to make sure people won’t have side effects. And maximum dose is 80 mg. So there’s almost an order of magnitude between the highest and lowest Prozac doses. Most people stay on 20 to 40, and that dose seems to work pretty well.
Suppose I have a patient with a mutation that slows down their metabolism of Prozac; they effectively get three times the dose I would expect. I start them on 10 mg, which to them is 30 mg, and they seem to be doing well. I increase to 20, which to them is 60, and they get a lot of side effects, so I back down to 10 mg. Now they’re on their equivalent of the optimal dose. How is this worse than a genetic test which warns me against using Prozac because they have mutant Prozac metabolism?
Or suppose I have a patient with a mutation that dectuples Prozac levels; now there’s no safe dose. I start them on 10 mg, and they immediately report terrible side effects. I say “Yikes”, stop the Prozac, and put them on Zoloft, which works fine. How is this worse than a genetic test which says Prozac is bad for this patient but Zoloft is good?
Or suppose I have a patient with a mutation that makes them an ultrarapid metabolizer; no matter how much Prozac I give them, zero percent ever reaches their brain. I start them on Prozac 10 mg, nothing happens, go up to 20, then 40, then 60, then 80, nothing happens, finally I say “Screw this” and switch them to Zoloft. Once again, how is this worse than the genetic test?
(again, all of this is pretending that dose correlates with plasma levels correlates with efficacy in a way that’s hard to prove, but presumably necessary for any of this to be meaningful at all)
I expect the last two situations to be very rare; few people have orders-of-magnitude differences in metabolism compared to the general population. Mostly it’s going to be people who I would expect to need 20 of Prozac actually needing 40, or vice versa. But nobody has the slightest idea how to dose SSRIs anyway and we usually just try every possible dose and stick with the one that works. So I’m confused how genetic testing is supposed to make people do better or worse, as opposed to just needing a little more or less of a medication whose dosing is so mysterious that nobody ever knows how much anyone needs anyway.
As far as I can tell, this is why they need those pharmacodynamic genes like HTR2A and SLC6A4. Those represent real differences between antidepressants and not just changes in dose which we would get to anyway. I mean, you could still just switch antidepressants if your first one doesn’t work. But this would admittedly be hard and some people might not do it. Everyone titrates doses!
This is a fourth grain of salt and another reason why I’m wary about this idea.
VI.
Despite my skepticism, there are several studies showing impressive effects from pharmacogenomic antidepressant tests. Now that we’ve established some reasons to be doubtful, let’s look at them more closely.
GeneSight lists eight studies on its website here. Of note, all eight were conducted by GeneSight; as far as I know no external group has ever independently replicated any of their claims. The GeneSight rep I talked to said they’re trying to get other scientists to look at it but haven’t been able to so far. That’s fair, but it’s also fair for me to point out that studies by pharma companies are far more likely to find their products effective than studies by anyone else (OR = 4.05). I’m not going to start a whole other section for this, but let’s call it a fifth grain of salt.
First is the LaCrosse Clinical Study. 114 depressed patients being treated at a clinic in Wisconsin received the GeneSight test, and the results were given to their psychiatrists, who presumably changed medications in accordance with the tests. Another 113 depressed patients got normal treatment without any genetic testing. The results were:
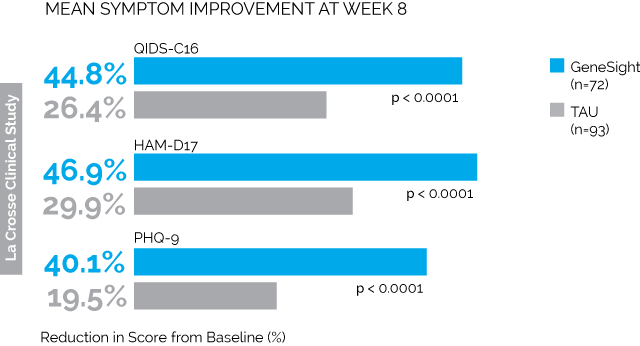
Taken from here, where you’ll find much more along the same lines.
All of the combinations of letters and numbers are different depression tests. The blue bars are the people who got genotyped. The grey bars are the people who didn’t. So we see that on every test, the people who got genotyped saw much greater improvement than the people who didn’t. The difference in remission was similarly impressive; by 8 weeks, 26% of the genotyped group were depression-free as per QIDS-C16 compared to only 13% of the control group (p = 0.03)
How can we nitpick these results? A couple of things come to mind.
Number one, the study wasn’t blinded. Everyone who was genotyped knew they were genotyped. Everyone who wasn’t genotyped knew they weren’t genotyped. I’m still not sure whether there’s a significant placebo effect in depression (Hróbjartsson and Gøtzsche say no!), but it’s at least worth worrying about.
Number two, the groups weren’t randomized. I have no idea why they didn’t randomize the groups, but they didn’t. The first hundred-odd people to come in got put in the control group. The second hundred-off people got put in the genotype group. In accordance with the prophecy, there are various confusing and inexplicable differences between the two groups. The control group had more previous medication trials (4.7 vs. 3.6, p = 0.02). The intervention group had higher QIDS scores at baseline (16 vs. 17.5, p = 0.003). They even had different CYP2D6 phenotypes (p = 0.03). On their own these differences don’t seem so bad, but they raise the question of why these groups were different at all and what other differences might be lurking.
Number three, the groups had very different numbers of dropouts. 42 people dropped out of the genotyped group, compared to 20 people from the control group. Dropouts made up about a quarter of the entire study population. The authors theorize that people were more likely to drop out of the genotype group than the control group because they’d promised to give the control group their genotypes at the end of the study, so they were sticking around to get their reward. But this means that people who were failing treatment were likely to drop out of the genotype group (making them look better) but stay in the control group (making them look worse). The authors do an analysis and say that this didn’t affect things, but it’s another crack in the study.
All of these are bad, but intuitively I don’t feel like any of them should have been able to produce as dramatic an effect as they actually found. But I do have one theory about how this might have happened. Remember, these are all people who are on antidepressants already but aren’t getting better. The intervention group’s doctors get genetic testing results saying what antidepressant is best for them; the control group’s doctors get nothing. So the intervention group’s doctors will probably switch their patients’ medication to the one the test says will be best, and the control group’s doctors might just leave them on the antidepressant that’s already not working. Indeed, we find that 77% of intervention group patients switched medications, compared to 44% of control group patients. So imagine if the genetic test didn’t work at all. 77% of intervention group patients at least switch off their antidepressant that definitely doesn’t work and onto one that might work; meanwhile, the control group mostly stays on the same old failed drugs.
Someone (maybe Carlat again?) mentioned how they should have controlled this study: give everyone a genetic test. Give the intervention group their own test results, and give the control group someone else’s test results. If people do better on their own results than on random results, then we’re getting somewhere.
Second is the Hamm Study, which is so similar to the above I’m not going to treat it separately.
Third is the Pine Rest Study. This one is, at least, randomized and single-blind. Single-blind means that the patients don’t know which group they’re in, but their doctors do; this is considered worse than double-blind (where neither patients nor doctors know) because the doctors’ subtle expectations could unconsciously influence the patients. But at least it’s something.
Unfortunately, the sample size was only 51 people, and the p-value for the main outcome was 0.28. They tried to salvage this with some subgroup analyses, but f**k that.
Fourth and fifth are two different meta-analyses of the above three studies, which is the lowest study-to-meta-analysis ratio I’ve ever seen. They find big effects, but “garbage in, garbage out”.
Sixth, there’s the Medco Study by Winner et al; I assume his name is a Big Pharma plot to make us associate positive feelings with him. This study is an attempt to prove cost-effectiveness. The GeneSight test costs $2000, but it might be worth it to insurers/governments if it makes people so much healthier that they spend less money on health care later. And indeed, it finds that GeneSight users spend $1036 less per year on medication than matched controls.
The details: they search health insurance databases for patients who were taking an psychiatric medication and then got GeneSight tests. Then they search the same databases for control patients for each; the control patients take the same psych med, have the same gender, are similar in age, and have the same primary psychiatric diagnosis. They end up with 2000 GeneSight patients and 10000 matched controls, whom they prove are definitely similar (even as a group) on the traits mentioned above. Then they follow all these people for a year and see how their medication spending changes.
The year of the study, the GeneSight patients spent on average $689 more on medications than they did the year before – unfortunate, but not entirely unexpected since apparently they’re pretty sick. The control patients spent on average $1725 more. So their medication costs increased much more than the GeneSight patients. That presumably suggests GeneSight was doing a good job treating their depression, thus keeping costs down.
The problem is, this study wasn’t randomized and so I see no reason to expect these groups to be comparable in any way. The groups were matched for sex, age, diagnosis, and one drug, but not on any other basis. And we have reason to think that they’re not the same – after all, one group consists of people who ordered a little-known $2000 genetic test. To me, that means they’re probably 1) rich, and 2) have psychiatrists who are really cutting-edge and into this kind of stuff. To be fair, I would expect both of those to drive up their costs, whereas in fact their costs were lower. But consider the possibility that rich people with good psychiatrists probably have less severe disease and are more likely to recover.
Here’s some more evidence for this: of the ~$1000 cost savings, $300 was in psychiatric drugs and $700 was in non-psychiatric drugs. The article mentions that there’s a mind-body connection and so maybe treating depression effectively will make people’s non-psychiatric diseases get better too. This is true, but I think seeing that the effect of a psychiatric intervention is stronger on non-psychiatric than psychiatric conditions should at least raise our suspicion that we’re actually seeing some confounder.
I cannot find anywhere in the study a comparison of how much money each group spent the year before the study started. This is a very strange omission. If these numbers were very different, that would clinch this argument.
Seventh is the Union Health Service study. They genotype people at a health insurance company who have already been taking a psychotropic medication. The genetic test either says that their existing medication is good for them (“green bin”), okay for them (“yellow bin”) or bad for them (“red bin”). Then they compare how the green vs. yellow vs. red patients have been doing over the past year on their medications. They find green and yellow patients mostly doing the same, but red patients doing very badly; for example, green patients have about five sick days from work a year, but red patients have about twenty.
I don’t really see any obvious flaws in this study, but there are only nine red patients, which means their entire results depend on an n = 9 experimental group.
Eighth is a study that just seems to be a simulation of how QALYs might change if you enter some parameters; it doesn’t contain any new empirical data.
Overall these studies show very impressive effects. While it’s possible to nitpick all of them, we have to remind ourselves that we can nitpick anything, even the best of studies, and do we really want to be that much of a jerk when these people have tested their revolutionary new product in five different ways, and every time it’s passed with flying colors aside from a few minor quibbles?
And the answer is: yes, I want to be exactly that much of a jerk. The history of modern medicine is one of pharmaceutical companies having amazing studies supporting their product, and maybe if you squint you can just barely find one or two little flaws but it hardly seems worth worrying about, and then a few years later it comes out that the product had no benefits whatsoever and caused everyone who took it to bleed to death. The reason for all those grains of salt above was to suppress our natural instincts toward mercy and cultivate the proper instincts to use when faced with pharmaceutical company studies, ie Cartesian doubt mixed with smoldering hatred.
VII.
I am totally not above introducing arguments from authority, and I’ve seen two people with much more credibility than myself look into this. The first is Daniel Carlat, Tufts professor and editor of The Carlat Report, a well-respected newsletter/magazine for psychiatrists. He writes a skeptical review of their studies, and finishes:
If we were to hold the GeneSight test to the usual standards we require for making medication decisions, we’d conclude that there’s very little reliable evidence that it works.
The second is John Ioannidis, professor of health research at Stanford and universally recognized expert on clinical evidence. He doesn’t look at GeneSight in particular, but he writes of the whole pharmacogenomic project:
For at least 3 years now, the expectation has been that newer platforms using exome or full-genome sequencing may improve the genome coverage and identify far more variants that regulate phenotypes of interest, including pharmacogenomic ones. Despite an intensive research investment, these promises have not yet materialized as of early 2013. A PubMed search on May 12, 2013, with (pharmacogenomics* OR pharmacogenetc*) AND sequencing yielded an impressive number of 604 items. I scrutinized the 80 most recently indexed ones. The majority were either reviews/commentary articles with highly promising (if not zealot) titles or irrelevant articles. There was not a single paper that had shown robust statistical association between a newly discovered gene and some pharmacogenomics outcome, detected by sequencing. If anything, the few articles with real data, rather than promises, show that the task of detecting and validating statistically rigorous associations for rare variants is likely to be formidable. One comprehensive study sequencing 202 genes encoding drug targets in 14,002 individuals found an abundance of rare variants, with 1 rare variant appearing every 17 bases, and there was also geographic localization and heterogeneity. Although this is an embarrassment of riches, eventually finding which of these thousands of rare variants are most relevant to treatment response and treatment-related harm will be a tough puzzle to solve even with large sample sizes.
Despite these disappointing results, the prospect of applying pharmacogenomics in clinical care has not abided. If anything, it is pursued with continued enthusiasm among believers. But how much of that information is valid and is making any impact? […]
Before investing into expensive clinical trials for testing the new crop of mostly weak pharmacogenomic markers, a more radical decision is whether we should find some means to improve the yield of pharmacogenomics or just call it a day and largely abandon the field. The latter option sounds like a painfully radical solution, but on the other hand, we have already spent many thousands of papers and enormous funding, and the yield is so minimal. The utility yield seems to be even diminishing, if anything, as we develop more sophisticated genetic measurement techniques. Perhaps we should acknowledge that pharmacogenomics was a brilliant idea, we have learned some interesting facts to date, and we also found a handful of potentially useful markers, but industrial-level application of research funds may need to shift elsewhere.
I think the warning from respected authorities like these should add a sixth grain of salt to our rapidly-growing pile and make us feel a little bit better about rejecting the evidence above and deciding to wait.
VIII.
There’s a thing I always used to hate about the skeptic community. Some otherwise-responsible scientist would decide to study homeopathy for some reason, and to everyone’s surprise they would get positive results. And we would be uneasy, and turn to the skeptic community for advice. And they would say “Yeah, but homeopathy is stupid, so forget about this.” And they would be right, but – what’s the point of having evidence if you ignore it when it goes the wrong way? And what’s the point in having experts if all they can do is say “this evidence went the wrong way, so let’s ignore it”? Shouldn’t we demand experts so confident in their understanding that they can explain to us why the new “evidence” is wrong? And as a corollary, shouldn’t we demand experts who – if the world really was topsy-turvy and some crazy alternative medicine scheme did work – would be able to recognize that and tell us when to suspend our usual skepticism?
But at this point I’m starting to feel a deep kinship with skeptic bloggers. Sometimes we can figure out possible cracks in studies, and I think Part VI above did okay with that. But there will be cracks in even the best studies, and there will especially be cracks in studies done by small pharmaceutical companies who don’t have the resources to do a major multicenter trial, and it’s never clear when to use them as an excuse to reject the whole edifice versus when to let them pass as an unavoidable part of life. And because of how tough pharmacogenomics has proven so far, this is a case where I – after reading the warnings from Carlat and Ioannidis and the Italian team and everyone else – tentatively reject the edifice.
I hope later I kick myself over this. This might be the start of a revolutionary exciting new era in psychiatry. But I don’t think I can believe it until independent groups have evaluated the tests, until other independent groups have replicated the work of the first independent groups, until everyone involved has publicly released their data (GeneSight didn’t release any of the raw data for any of these studies!), and until our priors have been raised by equivalent success in other areas of pharmacogenomics.
Until then, I think it is a neat toy. I am glad some people are studying it. But I would not recommend spending your money on it if you don’t have $2000 to burn (though I understand most people find ways to make their insurance or the government pay).
But if you just want to have fun with this, you can get a cheap approximation from 23andMe. Use the procedure outlined here to get your raw data, then look up rs6313 for the HTR2A polymorphism; (G,G) supposedly means more Paxil side effects (and maybe SSRI side effects in general). 23andMe completely dropped the ball on SLC6A4 and I would not recommend trying to look that one up. The cytochromes are much more complicated, but you might be able to piece some of it together from this page’s links links to lists of alleles and related SNPs for each individual enzyme; also Promethease will do some of it for you automatically. Right now I think this process would produce pretty much 100% noise and be completely useless. But I’m not sure it would be more useless than the $2000 test. And if any of this pharmacogenomic stuff turns out to work, I hope some hobbyist automates the 23andMe-checking process and sells it as shareware for $5.
A related question, how do you as a doctor find out information about drugs, treatments, etc? E.g. you want to find out the dosage and effects of a novel drug.
I assume/hope there is a more complicated system than just googling it. But I’ve found it hard to find useful information online.
UpToDate is like an expensive-but-carefully-verified Wikipedia for doctors.
(I’ve been known to google things in the rare cases when I don’t have UpToDate available)
Wikipedia mentions UpToDate is freely accessible in Norway by the website Helsebiblioteket.no (Norwegian Electronic Health Library) by any IP address in the country without any need for login. I tried using norwegian proxies but couldn’t access it.
Does anybody else have any info regarding this?
Also: https://www.ncbi.nlm.nih.gov/pmc/articles/PMC4260083/
Just speculating, but I wouldn’t be surprised if they block known proxies.
The problem arose only when I tried to access UpToDate. Other journals made available by the website were accessible via proxy.
Obviously, sales representatives tell you about them. 😀
And fed you lunch, to boot!
“GeneSight lists eight studies on its website are far more likely to find their products effective than studies by anyone else (OR = 4.05). I’m not going to start a whole other section for this, but let’s call it a fifth grain of salt.”
The link in this paragraph is broken, and it seems to have swallowed up some of your text.
Thanks, fixed.
But how many grains of salt would you say ought to constitute a heap?
you can totally have a heap of zero grains…
Yes, I myself favor universalism as the solution to a lot of metaphysical problems. Or, more rarely, nihilism. But this doesn’t look like one of the cases in which to employ nihilism; I prefer everything being a heap, probably as you suggest even including the empty heap (since having a zero case often makes things simpler), to there being no such things as heaps. And certainly any sort of moderate proposal of some things being heaps and some things being non-heaps is just the sort of thing that never ends up working in metaphysics.
Just add a clustered index and don’t worry about it.
This sounds really, really similar to “the passage of time is helpful to at least 66% of patients”.
…because with the genetic test, they don’t have to experience the “terrible side effects”? That sounds like an improvement to me.
The skeptic community is all over this particular question in the particular case of homeopathy. They will tell you at length that this evidence is necessarily wrong because all homeopathic treatments are chemically identical, and in fact are all water.
The other question, “what’s the point of having evidence if we’re just going to reject it if it turns out favorable to homeopathy”, then tells us that there is no point in collecting evidence on homeopathy. There’s no logical issue there. An experiment that wanted to provide meaningful evidence about homeopathy would have to first show e.g. that there was a chemical difference between one homeopathic remedy and a different homeopathic remedy, which would go towards contradicting the point “it’s all just water”.
But homeopathy’s point is that it works on a method other than the chemistry. I know and you know that chemistry matters, but you aren’t addressing their point if you only talk about chemistry.
Anyway, isn’t the easier explanation that it’s just green jelly beans? https://xkcd.com/882/
If you only talk about chemistry, you are addressing their claim that their methods are medicinally beneficial. Why do you need to address some other point of theirs? What point would that be?
Homeopaths claim that there are properties of water aside from chemistry (and temperature, pressure, and other things acknowledged by mainstream science) which are relevant to medicine. If you simply say “But chemistry/temperature/pressure don’t show any difference!” they’ll nod and agree with you.
Sure, the burden of proof is on their side if you want to get picky, but they don’t care.
My untested hypothesis is that some homeopathic remedies contain real drugs put in by the manufacturers so that people get better and keep buying the homeopathic remedy.
Depending somewhat on the drug in question, this would be an outrageously risky maneuver on the part of the manufacturers – as in, like, criminal liability – and would obviate one of the major benefits of being in the homeopathy business: trivial manufacturing costs. I suspect that the pure placebo effect is more than capable of keeping people on the sugar pill train.
(If you were being facetious then disregard this comment!)
Now I wonder if there is any standards body that would complain if the homeopathic method wasn’t made by 20C and instead just pumping out straight sugar pills.
It’s already been proven to happen, at least once. (See http://www.wired.co.uk/article/homeopathy-contains-medicine)
In Europe the term “homeopathic” is regulated, but in America it doesn’t mean anything and the term is slapped onto many substances whose label claims to contain measurable doses. Go into a drug store and look for zinc lozenges. The last time I did, they were all marked “homeopathic,” but I think that the label disagreed. I think that it is common for them to have a specific dose listed. Alternately, they may be labeled with the homeopathic notation “1X” which should mean that they were diluted 10:1, or hardly at all. In Germany that would be illegal, but still the rule is that they must be diluted 4X (aka 4D), 10000:1, which certainly leaves a measurable amount and may well a practical amount, depending on the substance.
This seems like a flawed approach. It’s attempting to rely on reasoning rather than empirical evidence.
This is not scientific
Bob: “XYZ works because of a non-chemical difference.”
Alice: “BAH! There is no chemical difference! Disproven!”
It would be like if the Randi Prize people had simply gone around declaring that since someones claims violated the current best understanding of physics they were going to refuse to test them.
Your position seems closer to classical philosophical Rationality than LW style rationality or empiricism.
If, hypothetically, someone did a well run and supervised double blind trial of a homeopathic remedy and the intervention arm did vastly better than the controls on placebo that would be spectacularly scientifically interesting if it could be replicated. Even more interesting if they followed up by showing that there was indeed no chemical difference between the remedy and the placebo.
But the point is not to collect evidence about homeopathy; it’s to collect evidence about science. We already know homeopathy is false, so if science provides evidence it works, that’s a problem. It means that science probably also provides evidence that other non-working things work, in cases where we don’t already know the answer (so we have to trust science). It suggests that we need a more robust, less optimistic process for proving things work, one weak enough that it can’t “prove” homeopathy works.
The only basis on which we know it is false is that science does not provide evidence that it works.
Because of the FDA loophole you mentioned, there are no real standards for these tests, and people are taking advantage of it. Here is a related story:
https://www.statnews.com/2017/02/28/proove-biosciences-genetic-tests/
A biotech company (Proove) claims it can use genetic tests and questionnaires to determine who will develop opioid dependence and which pain killers a person will respond best to. They get doctors to sign on to do ‘research’ on the effectiveness of the tests. The research measures the doctors impressions of whether the test helps. Some doctors fill out the forms themselves, but Proove will also send out employees to fill out the forms for them to help increase volume. The tests are billed to insurance companies, and the doctors get a payment for each patient the enroll in the ‘study’. Unsurprisingly, the responses show great benefit from the test.
Going back to this post, a detail that stood out to me was using a 15 year old candidate gene study to support the GeneSight test. These studies are now known to have abysmally low replication rates (I think John Ioannidis gives a figure of 98% non-replication in many of the talks he gives, I would have to look up the source). That’s kind of a red flag that they are thinking wishfully.
Finally, this part:
“I talked to the GeneSight rep about this, and he agreed; their internal tests don’t show strong effects for any of the candidate genes alone, because they all interact with each other in complicated ways. It’s only when you look at all of them together, using the proprietary algorithm based off of their proprietary panel, that everything starts to come together.”
Reminded me of this:
https://www.statnews.com/2016/11/29/brca-cancer-myriad-genetic-tests/
Another gene testing company (this time with a product that actually works. The BRCA test for detecting breast cancer risk) says that it has complicated proprietary methods that are better than its competitors, but produces no peer-reviewed or even public data to back up the claim.
Ya, I’m gonna agree with this point. There’s a lot of genes with only 1 or 2 papers about their function and the effects of many variants tend to get overstated.
One of the findings from ExAC was a note of how many subjects appeared to be walking around with 40 to 50 variants which according to the literature are linked to serious issues but appear fine.
recently the BMA published a list of something like 30 variants which they believed were the only ones which had sufficient evidence behind their link to serious health problems to ethically justify notifying patients if they’re found incidentally during other investigations.
Sometimes students will be looking into conditions trying to find associated variants and it’s a bit depressing how flimsy the evidence base is for many links. 10 year old guesses at gene function are pretty normal.
Throw in the “fuck it we can make money this week” attitude of the typical startup founder and you’re not going to get meaningful data or careful analysis. It’s worth taking a lot of things with a pinch of salt until they’ve been well replicated.
I doubt that Myriad’s proprietary algorithms are accurate, but even if they were, they are clinically irrelevant. They are probably like 23andMe’s claim that a particular SNP raised the chance of breast cancer from 10% to 12%, which is irrelevant to any decision (mammograms, prophylactic mastectomy). The only thing that matters are nonsense mutations.
But Myriad does offer something that other companies don’t: it will sequence your BRCA and look for a unique nonsense mutation, while everyone else will just look for the specific nonsense mutations that are common in the Ashkenazi and Dutch. You could get another company to sequence your whole exome for a fraction of the cost, but if you do have a unique mutation, Myriad will then build a boutique test to give to all your relatives. It is still probably cheaper to get all your relatives’ exomes sequenced, but it is close and insurance will cover Myriad. Anyhow, this is a really niche product, for the 1 in 10k people who already know that they have nonsense BRCA in their family but aren’t Ashkenazi or Dutch (or have already passed those tests).
“They are probably like 23andMe’s claim that a particular SNP raised the chance of breast cancer from 10% to 12%”
This is the one case I know of where that really isn’t the situation. Here are the stats from the article:
“if either gene is mutated, a woman’s risk of developing breast and ovarian cancer soars to as high as 85 percent and 40 percent, respectively. That compares with a risk of 12.7 percent and 1.4 percent in the general population.”
These results have held up over time, and women with the bad versions of the genes often opt to have a mastectomy rather than face such a high risk of cancer.
Which is why I mentioned that in my very next sentence. Taken literally, the sentence you quoted is false. It is only nonsense* mutations that have a dramatic effect. Both 23andMe and Myriad claim that other BRCA mutations have effects and they probably do, but they don’t matter. Whereas nonsense mutations are easy to recognize (although not as easy to detect as a SNP) and not a matter of proprietary algorithms.
* Added: I include frameshift mutations as nonsense mutations and not just stop codon mutations. I am not sure if this is standard.
Oh, sorry, I misunderstood your point.
As I understand it, Myriad and its competitors both sequence the same genes and have access to the same genetic information (since Myriad lost its patent protections in the Supreme Court in 2013). The difference is that Myriad currently has a larger database linking sequences to outcomes. Simply knowing whether the mutation is a nonsense mutation is not enough to determine its significance. Myriad claims that its larger database leads to more accurate results, and fewer rare mutations where no call can be made about the clinical significance due to lack of data. That seems to be true only in a very small number of cases.
No, it really is. “Nonsense mutation” is a technical term. (I include frameshift mutations as nonsense mutations. I don’t know if this is standard. In any event, both frameshift and stop-codon mutations have dramatic effects, hardly dependent on the location on the gene. Maybe the importance of a stop mutation depends on the location, but it would be a monotone function of location and pretty easy to figure out.)
I know that nonsense mutation is a technical term, but it’s not enough to determine the clinical significance of a mutation in BRCA1 or BRCA2 gene. There are rare variants where no one knows whether they increase the risk of cancer or not.
1. You sure don’t act like you know it. You also don’t act like to know the difference between a sign error and a magnitude error. (nor your link)
2. Yes, there are lots of rare missense mutations on BRCA. I don’t know the sign of their effect, but I do know the magnitude: clinically irrelevant. Myriad has a database of missense mutations. They probably know more than other people about these mutations. Probably they are 75% correct about guessing the sign of the effect. But even if everything they claim is correct, they only claim that the effects are tiny and worthless, just like the public information about the SNP that 23andMe used. Except that they don’t use the word “worthless” but leave it to genetic counselors to give bad advice.
3. The people who should be using Myriad are the people who already know that they have aggressive breast cancer in their families. Like Angelina Jolie. Except that she got it from her Dutch grandmother, so it’s probably the common variant and she didn’t need sequencing. If in such a person you see a nonsense mutation, you can be pretty sure that it is the problem, even if nonsense mutations are ever safe. Ideally you get a sample from someone who did get aggressive breast cancer, not Angelina Jolie, but her mother, aunt, or grandmother. Then you know that there really is a problem.
It’s not true that you just have to classify a mutation as nonsense or not to determine whether it is of clinical significance. Here is a source:
https://www.ncbi.nlm.nih.gov/pmc/articles/PMC2928257/
“Most deleterious mutations introduce premature termination codons through small frameshift deletions or insertions, nonsense or splice junction alterations, or large deletions or duplications. ”
So nonsense mutations are a common cause of major problems, but just because a mutation is not nonsense doesn’t mean it can’t have a major negative effect.
Sorry, I made a big reading error. I read “where” in place of “whether.” So my last couple of comments are non sequiturs.
I claim that only big errors matter. I claim that Myriad’s proprietary algorithm is about small errors. That paper acknowledges that some variants are higher risk than others, but fails to really exhibit belief in it.
I agree that there are more kinds of big errors than nonsense errors. The important claim is that big errors are easy to recognize. In particular, all the errors in that quote are easy to recognize. Maybe skipping an exon is a medium size error that is easy to recognize but hard to evaluate. Maybe that is a place where there is real uncertainty, useful knowledge that could be acquired, and perhaps is held by Myriad.
“Fourth and fifth are two different meta-analyses of the above three studies, which is the highest study-to-meta-analysis ratio I’ve ever seen.”
Probably you mean “[…] lowest study-to-meta-analysis ratio […]”
Highest: Most under the influence of marijuana.
The study I want to see done: Recruit participants in a study. Get informed consent from all of them for reports from their doctors &etc, tell them that some will be in the treatment and some in the control group, and all of the above.
Then tell the control group that the experiment was at capacity. Have the experimental group get the test, but no change in intervention.
If that produces a 20% reduction in symptoms in the experimental group, then I would suggest offering genetic testing as treatment is effective.
I feel like “don’t waste time on things which are obviously bullshit” and “actually decide based on the evidence” are both useful skills, and I’ve yet to work out what to do when they seem to conflict. I have some intuition for which to follow, but not sufficient certainty to say confidently which to follow when.
In practice I find this conflict mostly reduces to the skill of “accurately judge the trustworthiness of interpreters and synthesizers of the evidence.” But I’m mostly a consumer for broad-but-shallow knowledge rather than a specialist in anything. Specialists should probably apply their own judgement much more, in their areas.
The advanced-level skills for knowledge consumers, which I haven’t gotten the hang of yet, are “efficiently seek and find the most trustworthy evidence-interpreters for a given issue”, and “deduce which issues are likely to reward a search for evidence-interpreters, as a function of insight payoff versus search costs.”
I basically still rely on other people to do those things for me, and am therefore grateful every time Scott does (and reports back so thoroughly, besides).
Let’s call a spade a shovel. We all know what that data looks like – because it was omitted. If it had come out the other way, it would be in the article.
Related:
“Let’s call a spade a shovel. We all know what that data looks like – because it was omitted. If it had come out the other way, it would be in the article.”
I don’t like making these kinds of accusations, because every time I write an article and try to include everything I think is important, someone in the comments accuses me of deliberately leaving out the strongest argument for the opposing side for sinister reasons. When they tell it to me, it usually turns out to be something I figured was so far down the list of possibilities that it wasn’t worth anyone’s time to talk about.
I quite agree you shouldn’t make such accusations overtly; they would make you look petty, personalise the subject and detract from your larger point. And how would you prove it? You have a reputation to maintain.
But yahoos like me in the comments section are able to make explicit the not very subtle subtext here. You can write “This is a very strange omission,” and I can say what I really think.
You don’t need that many genes to make it hard to figure out what is going on.
Besides the potential for each gene to have multiple alleles, the effect a particular gene has can depend on what the rest of the genome contains.
For what it’s worth, the current estimate of (protein-coding) genes in the human genome is around 20,000. I propose that as a conservative upper bound on the number of genes which might be involved in any trait.
I think usually people find that genetic effects are basically additive.
You mean the genetic effects people have detected are additive.
When getting the result involves doing an ANOVAR or similar, maybe that’s not surprising. All of the ‘non-additive’ effects effectively become noise in the variation within groups and give you the small detectable effects you observed in the literature in the first place.
Non-additive effects would be something like a mutation in a regulator which leads to reduced expression of the enzyme you care about. There may be dozens of relatively rare but individually important interactions you don’t detect when all you look at is the enzyme sequence.
In case it’s not clear I should point out that the upper bound of 20000 I mention above is tongue in cheek. I think it’s reasonable to say that for most measurable traits there will be a few genes with a relatively large effect, some which monkey around with those and a rapid tailing-off of importance.
“There’s a thing I always used to hate about the skeptic community.”
I’ve been saying for years – if you applied to standards of evidence that many in the skeptic community call for in essentially all situations, you simply couldn’t function as a human being. In many areas we’d be rendered completely inert because there simply doesn’t exist evidence that they consider robust. I mean if we were only allowed to teach using methods that we know from randomized controlled studies to be superior to alternative methods, we’d have almost nothing to do in the classroom because almost nothing survives research of that level of rigor.
It’s come up on the site before — the opinion that made the most sense to me went something like “no, the skeptics aren’t any better than anyone else at knowing what’s true. What they’re good at is exposing fraud that they know exists based on their community values”. (And this is still a valuable thing! There’s so much fraud out there.)
Think of them as an engine for generating disbelief in a fairly well-defined set of core values, not as an engine for generating knowledge that didn’t exist before.
To follow up on what was probably intended as just one random example of many:
I worked briefly on a team doing research synthesis of education RCTs. There are lots of problems, but one of the biggest is the control groups. There’s no standard control curriculum, but the control kids of course have to be getting some kind of education. The typical control is “practice as usual,” which could mean radically different things depending on region, school district, and individual teacher.
Worse, published reports are terrible about describing what the control group actually did. Authors aren’t that interested – they want to focus on describing their own intervention instead of what the control does – and may not even know in much detail. Trying to estimate effects over control, in a way that is comparable across studies, is a huge challenge when the control conditions are both highly variable and poorly described.
Exactly right.
Could it be that their test likes to assign certain exotic medications to the “red bin”. Being exotic, doctors only prescribe them to patients where everything else has failed and who desperately need something.
Interesting thought. Someone (maybe Carlat again) pointed out that there’s a lot of room for mischief if they do just put the same (presumably bad) medications in the red group for everybody. I was thinking this didn’t matter because there aren’t any globally bad antidepressants, but your way would definitely work too.
I feel like if this were the case, we’d just have to look for bimodal distributions when evaluating antidepressant effectiveness.
I think that’s sort of what the growth-model-trajectory paper is doing. I’m not sure why I don’t see people just using the words “bimodal distribution”, though.
At a guess, people don’t want to assert that it’s bimodal specifically?
There’s a similar claim saying that antidepressants only help people with very serious depression, and all the moderate cases only work by spontaneous recovery. If both of those narratives are true, we’d have one “good” bucket, two “not great” buckets, and one “hopeless” bucket.
Presumably there are lots of other similar assertions you could make, like talking about who responds to SSRIs versus NMRIs. So broad-spectrum studies find nothing, clinicians find something, but the number of buckets could be 2 or 32 or even more.
I want to examine the implications of this.
Given that systems can be gamed, we should expect that the above described pharmaceutical companies to attempt to game the system. Given no system, we should expect the above described companies to attempt to manipulate the market. We should also expect them to be successful if there is no coordinated attempt to stop them.
So, what does that mean for any coordinated attempt to stop them? It means that the system is not going to be charitable or even very efficient.
The reason the FDA “sucks” is because the market they are regulating “sucks” more.
I can certainly see the argument for this. Medical markets are famously irrational: people will pay arbitrary amounts of money and can’t assess results well, so spending doesn’t reflect preferences and information is badly asymmetric. Therefore, you need Draconian regulations just to cut down on deadly fraud a little bit.
But I think the counterpoint is that the FDA isn’t oppressively fighting fraud, it’s just sort of blundering around ruining things. Grandfathering drugs doesn’t make sense, setting equivalent evidence standards for redundant symptom managers and novel life-savers doesn’t make sense, barring international reciprocity doesn’t make sense, accepting studies with known flaws and no replications doesn’t make sense, and so on.
So we have a user-hostile, dishonest market which deserves our “smoldering hatred”. And then we have a risk-averse, regulatory-captured regulator adding random chaos and harm that can’t really be justified by the hatred.
It sounds to me like a whole lot more needs to be known about how the drugs behave in people’s bodies. Once that’s established, we’ll know more about which genes (and epigenetic changes, I bet) to look at.
cultivate the proper instincts to use when faced with pharmaceutical company studies, ie Cartesian doubt mixed with smoldering hatred
A dream career for me, matching my strengths and capabilities, if only I were mathematically able! 🙂
More broadly, the genomics approach – where, if any of you remember, Great Things were going to happen as soon as we mapped the human genome (like “personalised medical treatment targeted at an individual based on their genes”) – is partly why I’m sceptical on God-Emperor AI: reality is complicated, there are a lot of things that go with other things to cause third things which down the line cause sixteenth things, and every time we think we’ve cracked it and got one simple model that will let us do all this great stuff with a tweak here and a nudge there – it’s not that easy.
To be fair to the pharamcogenomics crowd, their idea is not crazy and it’s an avenue worth exploring, but I think we’re (for now) only going to get very broad/weak effects where “population with this gene will do slightly better on this drug and population with this gene will do slightly better on that”, which is better than nothing (at least if it saves the “take this drug – it’s not working – keep taking it – still not working – try a higher dose – nope, no good – okay, try this one instead” approach and we go straight to “try that one instead” first).
Just in my short lifetime, we’ve been promised medicine-redefining breakthroughs from stem cells, human genome sequencing, personalized genome sequencing, cloned organs, and nanoparticle drug delivery. CRISPR looks to be next. But the biggest actual breakthroughs have mostly been simple mechanical improvements to known processes (e.g. small-incision, robotic surgeries).
Certainly, I think we should assume that any new development which will be “important and ready soon” is going to hit horrible unforeseen issues, like primate cells proving way harder to clone than other mammal cells.
I’m not sure how you mean the AI connection, though? If you mean “it won’t happen as soon as people think”, I can see the shared pattern where things like the Dartmouth AI conference wrongly assumed that all the hard breakthroughs were done. If you mean the viability or eventual outcome of strong AI, I don’t really follow – unlike medical breakthroughs, we have solid proof that human-intelligence agents can be made (i.e. humans) and are just baffled about how to get there.
I’m not sure how you mean the AI connection, though?
Broadly in the sense that “holy cow, we figured out how to do this thing, huge immense changes to the world as we know it will shortly follow!”
And maybe they do (penicillin*) and maybe they don’t, but certainly not in any of the ways we expect they will. So I remain more convinced that the problem of AI will not be “rogue AI decides to turn all humans into paperclips” but “humans use AI to achieve an aim – generally doing down some opponent, rival or competitor – and unintended consequences follow”.
*e.g. in Samuel Delany’s “Nova” the taboo against sharing food directly from one mouth to another no longer exists, because disease no longer exists, because antibiotics have done away with all sickness. Yeah. Plainly superbugs and antibiotic resistance were not visualised as being a problem in the 60s.
“Suppose (say the pharmacogenomicists) that my individual genetics code for a normal CYP2D6, but a hyperactive CYP2C19 that works ten times faster than usual.”
I believe you meant for a “Not” to be inserted here: “my individual genetics code NOT for a normal CYP2D6, but a hyperactive CYP2C19… “ Unless I’m really mis-reading you.
Very interesting post. The problem of antidepressant pharmacogenomics seems like a sub-problem within the larger issue of the irreproducibility of candidate gene studies. In the 90’s/early 2000’s, there were hundreds of studies looking for associations between specific genes and individual phenotypes. People tested candidate genes that they thought might be associated with a trait (say, hormone receptors and sexuality), and published positive results linking single genes with homosexuality, intelligence, alcoholism, etc. However, about 15 years ago, it became much easier to do genome-wide association studies in 1000’s of people – and approximately none of these candidate genes previously found were reproduced in well-powered genome-wide cohorts. What the genome-wide studies found were dozens of genes each explaining 0.1%-1% of the variance in a trait, as Scott notes above. This led to what’s sometimes called the “dark matter” problem of human genetics – we know that intelligence is ~50% genetic, but using all of the markers we’ve found so far, we can only explain ~5% of the variance in intelligence by looking at a genome. We don’t know where the rest of the genetic variation comes from.
So, not having read the studies linked above but knowing a little about the progress of human genetics research, I agree with Scott’s skepticism that an 8 gene panel could accurately predict a very, very complex phenotype like anti-depressant response.
Promethease is based on SNPedia, and we’re very much in agreement with your analysis. We keep waiting for robust pharmacogenomics findings, and we will be more than happy to include them in SNPedia – and therefore in our $5 Promethease reports – when they come about.
So far, people learn more from their caffeine metabolism and lactose intolerance predictions than from “straight” PGx, although the increased myopathy risk for carriers of certain SLCO1B1 variants when taking statins seems pretty robust too.
And for readers of these comments (and article): feel free to point out your favorite replicated/robust PGx finding, especially if it’s not adequately represented in your view in SNPedia at the moment. We are always adding new information, and there’s bound to be good PGx info sooner or later … no?
Hey, thanks for everything you’re doing, you guys are great.
Do you think we’re at the point where someone can figure out their CP450 alleles from a 23andMe report? I tried to do it myself with Promethease last night and it looked like maybe if I’d spent a really long time learning exactly how to do it I could get a pretty good idea. But I was surprised that it wasn’t automatic on Promethease and I’m wondering whether it’s not possible with any decent level of accuracy.
Also, I see you list a lot of SNPs like “7x higher chance of responding to antidepressants”. I know you guys mostly just quote studies and don’t set yourselves up as gatekeepers, but do you think those kinds of things are plausible, or do you think it’s probably so polygenic that no individual SNP can matter much?
As you realize (and as discussed in SNPedia here), there are over 60 cytochrome genes classified into 14 different subfamilies.
We can figure out some of those 60, and that conclusion usually manifests itself as a ‘genoset‘, since it takes the data from multiple variants to determine one (let alone two) alleles. And an additional problem is that current DNA chip data is unphased, so we can’t know for sure when variants seen in the same gene are actually on the same allele.
As for being gatekeepers, we are a wiki, but we state our guidelines for the types of studies we strive to include in SNPedia in part as follows:
… Our emphasis is on SNPs and mutations that have significant medical or genealogical consequences and are reproducible (for example, the reported consequence has been independently replicated by at least one group besides the first group reporting the finding). These are typically found in meta-analyses, studies of at least 500 patients, replication studies including those looking at other populations, genome-wide significance thresholds of under 5 x 10e-8 for GWAS findings, and/or mutations with historic or proven medical significance. …
We’d love to have a single statistic indicating “primo data that is 99.9% likely to withstand the test of time” but we have to do the best we can with science publications as they exist today.
With respect to the discussion of titration, I think that the best-case scenario for gene testing is on the margin of what you are discussing. If you titrate the doses, you may end up in an equilibrium where the drugs are effective, but there are noticeable side effects so that it’s only just beating a cost-benefits analysis for the patient. It could be that at 40mg Prozac a patient has uncomfortable side effects but the Prozac is working so you stop titrating and stick with that. But maybe if you tried Zoloft you would find that they would have the same effectiveness with zero side effects which would be a huge win. Changing could be too risky with the titrating approach. If gene testing were actually effective then you wouldn’t have to worry about getting stuck in local maxima as much.
Separately, it seems weird that genes would account for such a small percentage of variation in drug metabolism. If not genes, there has to be something. Perhaps the next stage is doing association studies on gut biome variables with drug metabolism. Does anyone have anything to say on the state of that research effort?
I think describing the flaws you found in the LaCrosse study as “cracks” is significantly under-selling them. There were .77/.44=1.75x as many medication-changes in the intervention as in the control group, and an average QIDS-C16 improvement 44.8/26.4=1.7x as large. When I find a flaw that severe, I discount the study’s evidence-strength all the way to zero, and then discount it a little bit more, treating it as evidence against its conclusion.
Yeah, I don’t take that one too seriously. The Union Health one really bothers me, though. Sure, n=9 gives me an excuse to dismiss it, but I really want to know how they did it.
It’s really absurd they didn’t randomize their study. In my single grad course in Causal Inference the entire class revolved around the idea that you really really should always randomize, and if it’s impossible here are the econometric techniques that can hopefully get you closer to randomization.
It’s obviously great you went into it in more detail, but the fact that they didn’t randomize to me is sufficient to dismiss their evidence (conditional on the priors we know that anti-depressant signals are incredibly weak).
Where did you take this class (and in what department, econometrics?) Or did I ask you already?
—
I broadly agree with “always randomize if you can” thought, but you will still have non-compliance and dropout and all sorts of other things that will necessitate causal inference kung fu to deal with.
I took this one (http://www.lse.ac.uk/resources/calendar/courseGuides/MY/2016_MY457.htm).
I’d have loved to take more advanced stuff, but I’m stuck self-teaching, since I’m in the private sector now.
I’m just so surprised they didn’t randomize, and instead took the first 100 in Group A and second 100 in group B. It seems almost an unforgivably naive mistake, but perhaps there was some complicating factor I’m unaware of.
If you are still in London and have money:
http://www.lshtm.ac.uk/study/cpd/causal_inference.html
I thought the same thing, although I don’t know enough about the field to offer any suggestions. I do wonder if that is what the company is already doing. If they have a rich dataset and are using a forest method, I’d be willing to give them a little bit more benefit of the doubt than if they just have a regression with a bunch of interaction terms.
Still, I know nothing about the field.
From the National Education Alliance for Borderline Personality Disorder (NEA-BPD):
*-*-*-*-*-*-*-*-*
Borderline personality disorder (BPD) is a serious mental illness that centers on the inability to manage emotions effectively … Other disorders, such as depression, anxiety disorders, eating disorders, substance abuse and other personality disorders can often exist along with BPD … BPD affects 5.9% of adults (about 14 million Americans) at some time in their life …
Borderline personality disorder often occurs with other illnesses … Most co-morbidities are listed below, followed by the estimated percent of people with BPD who have them:
* Major Depressive Disorder – 60%
* Dysthymia (a chronic type of depression) – 70%
* Substance abuse – 35%
* Eating disorders (such as anorexia, bulimia, binge eating) – 25%
* Bipolar disorder – 15%
* Antisocial Personality Disorder – 25%
* Narcissistic Personality Disorder – 25%
* Self-Injury – 55%-85%
Research has shown that outcomes can be quite good for people with BPD, particularly if they are engaged in treatment … Talk therapy is usually the first choice of treatment (unlike some other illnesses where medication is often first). …
Dialectical behavior therapy (DBT) is the most studied treatment for BPD and the one shown to be most effective. … DBT teaches skills to control intense emotions, reduce self-destructive behavior, manage distress, and improve relationships. It seeks a balance between accepting and changing behaviors …
Medications cannot cure BPD but can help treat other conditions that often accompany BPD such as depression, impulsivity, and anxiety. …. Often patients are treated with several medications, but there is little evidence that this approach is necessary or effective.
*-*-*-*-*-*-*-*-*
The moral of this story seems pretty simple: use antidepressants to remedy synapse disorders; use therapy to remedy connectome disorders; be mindful in clinical practice that confusing these disorders and/or reversing these treatments is harmful.
Here’s a reasonable question: Given the high prevalence of BPD, can any pharmaceutical study be assessed as reliable that does not explicitly control for the (very many) patients for whom BPD is a credible primary diagnosis?
In other words, isn’t everyday life administering a long-term regimen of “dialectical behavior therapy” to pretty much every living person? Despite the (admitted) inconvenience and added complexity, isn’t BPD a clinically crucial variable, that requires careful experimental control, in pretty much any depression-related outcome study?
Most antidepressant studies exclude people with alternate diagnoses, including personality disorders. Although many people with BPD have depression, most people with depression don’t have BPD.
I think the distinction between “synapse disorders” and “connectome disorders” is way too speculative at this point to do anything with. For example, schizophrenia seems like both; schizophrenics have altered brain connectivity, but they also do a lot better on dopamine antagonists.
*-*-*-*-*-*-*-*-*
(from the OP) “Clinicians keep the patients who get good effects [by] switching drugs for the patients who get bad effects until they find something that works.”
(from above) “I think the distinction between “synapse disorders” and “connectome disorders” is way too speculative at this point to do anything with.”
*-*-*-*-*-*-*-*-*
Yet doesn’t the former (common) clinical practice help blind physicians to the everyday reality of the latter distinction?
The portion of patients for whom “drug-switching” fails is substantial (to say the least). It isn’t just physicians who (in the words of the OP) “feel kind of bad about this.”
Eva Candle,
I don’t see how you invoke connectomics as an “everyday reality.” At very best it’s an emerging domain.
Don’t get me wrong, connectomics is very exciting. Speaking personally, there is the potential for great strides within my profession (epilepsy). But vis-a-vis clinical practice, it remains a hypothetical.
Rahien.din, definitely I agree with you that in three great spheres of discourse—scientific, medical, and popular—the distinction between “synapse disorders” and “connectome disorders” is least widely embraced in the medical sphere.
In contrast, the confluence of scientific and popular discourse in regard to “synapse disorders” versus “connectome disorders” is noted in Robert B. Laughlin’s flawed-but-stimulating essay “Physics, Emergence, and the Connectome” (Neuron 2014, available on-line), specifically in regard to the crucial role in the scientific understanding of the connectome of the popular notion of “play activities.”
Here Laughlin’s notion of “play” is sufficiently broad that even psychiatric regimens like psychotherapy are to be understood as “play activities” that are deliberately structured so as to exert a therapeutic effect upon the connectome.
There is any event, a much more natural identification of “psychotherapy” with “play” than with “pill-taking”. 🙂
Much more can be said in this regard, and future SSC posts no doubt will afford opportunities to say it. Most importantly, there is plenty of scope for varied perspectives and reasoned disagreement as to what it all means.
Thank you for your to-the-point observations, Rahien.din.
I might be missing something basic about anatomy / biology but why do we have to go all the way to genetics to discover a person’s metabolic response to a given substance, as opposed to sampling their blood at periodic intervals after ingestion and measuring it directly ?
This is the plasma level point. Those sound like they should work, but don’t, maybe because there’s large individual variation in how much gets from the plasma to the brain. As the Italian team pointed out, if plasma levels themselves don’t help, it’s unclear why metabolism should.
Right that’s basically what I don’t understand on the premise level – if there are no metabolic effects not expressed by blood contents that we already know how to measure, what is the claimed value proposition of pharmagenomics here that prompted all this research to begin with ?
Because for a lot of drugs, they’re not active in the plasma. They’re active in brain cells (so have to get past the blood – brain barrier), they’re active in cells, which means their activity is modulated by receptors, and there may be modifications that need to happen once they’ve been brought into the cells, etc.
I don’t know this literature all too well, but I think you can start to resolve this by focusing on the fact that plasma drug levels are an extremely crude but easy-to-measure proxy for the actual parameter of interest: the free drug concentration in the CNS compartment. The pharmacokinetics for SSRIs in plasma tend to be wildly different than in the CNS, where they’re often achingly slow to accumulate and much faster to clear. I suspect that the major issue here is that plasma levels, low hanging fruit though they may be, are just poor approximators of CNS levels; note that Papakostas & Fava cite a study that did show a correlation between efficacy and cerebrospinal fluid levels (and it’s worth noting that even something as unpleasant as a spinal tap only gives you another, somewhat better proxy measure, since the CNS and CSF are separate compartments with a barrier between them.)
Of course, that argument is blown out of the water if in vivo measures of SSRI-SERT binding don’t show any relation to efficacy. The papers I’ve seen claim that there is a correlation (here and here, for example), but I’d be interested in seeing any conflicting studies.
Alternative hypothesis: as long as the side effects of SSRIs are dose-dependent, then higher doses make for more active placebos; thus, the placebo effect of SSRIs might be dose-dependent even if their on-target effects are not.
Great post by the way.
If plasma levels are proportional to CNS levels, and CNS levels are proportional to efficacy, then plasma levels should definitely be proportional to efficacy. So if you are correct, the objection is strengthened, and expanded.
That’s true in the simple case of a strong correlation, but those are not the correlations that generally obtain here. Plasma:CNS ratios often vary non-linearly, with weird ceiling effects; change substantially over time, with slow CNS accumulation followed by precipitous drops when you miss doses; and are heavily modulated by idiosyncratic individual factors (genetics of drug transporters, age, other meds, other conditions that affect BBB permeability).
The correlations are strong in lab mice sharing a cage and a diet, but in wild human populations people with the same plasma exposure can have CNS levels all over the map. There’s some relationship there, obviously, but I suspect just not enough signal to rise above the noise when you’re trying to correlate with another intrinsically noisy metric like antidepressant efficacy.
Mediocrates,
Agreed!
Maybe I misunderstood you, because, I think we’re ultimately saying the same thing – the defining feature of this plasma:CNS correlation is its crudeness.
Agreed to agree!
I was at a talk not long ago on pharmacogenomics which was pretty bearish on CYP testing for medicine in general, simply because the concordance between genotype and phenotype was bad enough to lack predictive value. I don’t know if that’s mostly a result of polypharmacy inducing and inhibiting enzymes or what, but it’s certainly discouraging.
Slightly meta: I think the question we should ask ourselves here is: “Are we looking at Depression from the wrong level of abstraction?”
My hypothesis is that yes, the “neurochemical view” is a terrible way to look at depression, and instead we should be looking at the information-theoretic view.
http://opentheory.net/2016/12/principia-qualia-executive-summary/
Scott, how long does it take you (median, hi-low) to find the right medication and titrate the right dose?
A friend of mine spent ca 6 months indoors until they found something that stopped his mania. I’m pretty sure they were inefficient and superstitious (trying for “interactions”/combos before trying all different classes and wanting to keep him on the extraneous meds that hadn’t helped when he finally stabilised with valproate).
Because that’s the cost I would think we’re paying for not having good matching of patient to treatment, but probably 6 months is a high estimate?
That said, I think your argument from GCTA was pretty much sufficient to dismiss this candidate gene crap. I’m not sure what you mean by exploiting variance vs. predicting variance, but if a SNP is reasonably common (if not, why test for it) it should be picked up in GCTA or GWAS. If it isn’t common, testing for it won’t help many.
What I’d like to know is some quantitative estimate of response heterogeneity. Without trying to identify moderators, just how much variation is there around the main effect. I’d assume to reliably estimate this for single persons, you’d need to test people a couple of times before and after. My feeling is also that there is high heterogeneity, but I’d like a number on this and then I’d like to know how much is left to be explained after accounting for age, severity, comorbidity, “resources” etc.
My takeaway from all this is that it’d be more worthwhile to get out all the study clinical reports from the file drawer and synthesise them to properly rank antidepressants. Did you read this http://crystalprisonzone.blogspot.de/2017/02/publication-bias-hampers-meta-regression.html? A compelling argument against the dodo bird verdict (both in psychotherapy and psychiatry) IMO.
Probably half of people do well on their first drug/dose. Antidepressants usually take a month to work, so it takes about a month to see if someone’s doing well on the first try. Then maybe another half will do okay after three or four months of playing around. This is my personal impression and not placebo-controlled; the placebo-controlled version of this is STAR*D.
Although I agree it would be good to cut out the experimentation, this shouldn’t have such exceptional effects on the studies where people have already been tried on many different drugs and doses.
The NIH’s web page “Questions and Answers about the NIMH Sequenced Treatment Alternatives to Relieve Depression (STAR*D) Study — All Medication Levels” states:
*-*-*-*-*-*-*-*-*
“So that results could be generalized to a broad group of real-world patients, most adults with MDD [major depressive disorder] were eligible.”
*-*-*-*-*-*-*-*-*
This is affirmed by (for example) Laje et. al. “Pharmacogenetics Studies in STAR*D: Strengths, Limitations, and Results” (2009), who flatly state “STAR*D did not assess personality traits.” These same authors also assert “STAR*D had no placebo arm; thus all participants received active treatment”.
So is it correct to say (in particular), that STAR*D made no systematic effort to exclude and/or differentiate patients with (for example) BPD, or any other classification of personality disorder?
Is STAR*D in this respect a notable exception to your statement (above) that “Most antidepressant studies exclude people with alternate diagnoses, including personality disorders”?
Also, was STAR*D placebo-controlled, or not?
Are there published (or presently underway), to your knowledge, any long-term studies that are comparable in scope and quality to STAR*D, whose protocols do distinguish among personality traits and disorders, as a relevant clinical variable in the treatment of depression?
And MOST importantly, thank you VERY MUCH for your efforts to clarify these very difficult, very important issues for a broad audience.
My impression was that research clinicians tend to think of TAU as maybe slightly better than harmful (i.e. matching and titration both aren’t usually done well). You have a higher opinion of your colleagues apparently.
I’m not convinced that antidepressants have any effectiveness beyond their placebo effects. Consider the “rule of thirds”:
“The priest said that about a third of the people he ministers to are healed, another third are ‘noticeably improved’, and the other third are unchanged.”
—From an article about faith-healing: “Finding power in the Holy Spirit”, by Willmar Thorkelson (religion editor), The Minneapolis Star, page 1B. May 21, 1975.
“The data from our sample of 6,931 patients, who underwent five noneffective treatments, clusters around one-third excellent results, one-third good results, and one-third poor results…”
—From a research article about the characteristics of the placebo effect: Roberts AH, Kewman DG, Mercier L, and Hovell M. (1993). “The power of nonspecific effects in healing: Implications for psychosocial and biological treatments”. Clinical Psychology Review, 13, pages 375-391.
“Put 100 patients on any antidepressant, and about a third will respond beautifully. Another third will have a partial response and the last third will not respond at all.”
—Dr. Pierre Blier, as quoted in The Gainesville Sun: “A speedier remedy for depression” by Diane Chun. January 12, 2002.
All good trials are placebo-controlled. See https://slatestarcodex.com/2014/07/07/ssris-much-more-than-you-wanted-to-know/ for a more complete discussion of this.
I’ve read all your stuff on SSRIs, and despite having training in experimental methods, ever since I went on SSRIs when I was younger (and subsequently came off), It’s been obvious to me they can work in some situations.
I know people talk about anecdotes, and how our brains are unreliable, and we can’t trust ourselves. Yet I distinctly remember when I started celexa this insane, gushing, overwhelming, euphoria, bubbling in the pit of my stomach and exploding through my brain. As it dissipated over the first couple months, my awful anxiety was completely gone. So much so that it was an uncomfortable personality change, and I found myself making choices and saying things that didn’t match my previous self.
I cannot reconcile this massive biochemical reaction I experienced with a state of the world where antidepressants are strictly placebo.
Typo:
23andMe uses the opposite strand convention as everyone else, so everyone else’s (C,C) equals their (G,G). I think. Unless they’ve changed since the last time I looked into this.
Ah, gotcha. Hope that doesn’t cause any predictable interpretative tragedies (“Good lord – positive for Huntington’s!”) ever.
Given the weak evidence base, the GeneSight test so far seems more cost than benefit. I’ve had a few clients come to me (I’m a talk therapist) who were patients in large practices of psychiatric NPs and these practices were wholesale using the GeneSight test on all of their patients. Imagine the money! Almost like giving an MRI to every patient who comes in for an annual checkup with their GP.
For some of my clients, their GeneSight results validated what they’d already observed in themselves — “I had a horrible reaction to Prozac and it turned up in the ‘red’ column for me.” GeneSight produces a report for patients that is a list of antidepressants sorted into red, yellow, green categories based on how “good” or “bad” those drugs are for them… an analysis that reaches beyond the capacity of their test it seems to me. I would think this kind of report has the potential to override relevant experience the patient or their doctor might have — is a psychiatrist going to feel they’re doing something risky by prescribing a patient a drug from the “red” column if that patient has failed to respond to a bunch of drugs from the “green” and “yellow” columns? Could this genetic test essentially foreclose options that might otherwise be helpful?
I wonder if patients wouldn’t do better just to look at a drug metabolism table like this — http://medicine.iupui.edu/clinpharm/ddis/main-table — and circle drugs they’ve taken and provide information about what doses were effective and how big their side effects were. I know for me chemicals metabolized mainly by the 1A2 and 2D6 liver enzymes tend to affect me at really low doses, for much longer than the reported half-life, and that the side effects are bigger than most people seem to report.
I’ve not seen research about whether the magnitude of withdrawal/discontinuation symptoms is also correlated with being a poor metabolizer of those drugs. It sure seems to be in my experience. I wish in general that doctors talked more about possible discontinuation symptoms — I see so many people who no longer want to be on the drugs they’re on but are having a very hard time getting off of them, and wish they’d been warned of that possibility at the time they decided to start on the drugs.
It seems likely to me too that other factors in one’s life may have a stronger effect on antidepressant metabolism than genes — coffee or alcohol consumption, status as a smoker, age, overall health, what other drugs they’re taking, particular diet and supplements, and toxic exposure. A weekend of spraying Roundup in the yard can significantly occupy liver enzymes for awhile.
And then I wonder too how much liver enzyme levels can change in response to environment. I gather we up-regulate liver enzymes in response to habitual consumption in some cases — so that some of the “tolerance” we get to things like nicotine or caffeine or cannabis is due to the body increasing enzyme levels to metabolize them. I would imagine our individual capacity to up and down-regulate liver enzymes is itself determined partly by genetics and partly by environment — some people maybe have very adaptable liver enzymes and others not so much. So that variable adds a dynamic dimension to pharmacokinetics that seems would be equally important to understand, but that we have no way to test for right now other than through the kind of trial-and-error experimentation that happens one-on-one with a smart psychiatrist over time.
Another variable I wonder about is how much range there seems to be in how responsive people are to the placebo effect. My unscientific observation is that people with tightly wound nervous systems (leaning anxious or PTSD, say) seem to experience both larger psychosomatic symptoms and larger placebo effects. This makes intuitive sense to me, so it’s possible I’m just doing confirmation bias. At any rate, people who tend to experience a lot of side effects and/or a lot of seemingly psychosomatic symptoms may complicate the process of using something like GeneSight tests to guide prescribing. I have clients who got on the first antidepressant they were ever prescribed and just stayed on it happily for years and others who have tried a dozen or more and respond intensely and well in the short-term to every drug they’ve tried before they poop out after two months. My guess is some of this has more to do with temperament than gene expression.
The handful of people I’ve met at either end of the bell curve — very poor metabolizers and super metabolizers — say it took them years of bad experiences with doctors before they found one who was willing to listen to their experience in enough detail to be willing to go very low or very high on dosage. The poor metabolizers had psychiatrists dismiss them for being on “subclinical” doses of a drug and essentially refuse to work with them because they wouldn’t take a “normal” dose even though the very low dose was providing relief from depression. It would help if psychiatrists overtly acknowledged the wide variety in metabolic-influencing variables and weren’t so quick to say “well, we start everyone at this dose…” It seems to me it’s worth some effort to find the lowest effective dose.
Given the heap of salt and these other confounding variables, I’m thinking psychiatrists are not yet at risk of being replaced by robots. Hard to say about us talk therapists, though. 🙂
What company is the best (cheapest) if I want to check a specific gene for mutations?
I cannot find this kind of service on 23andme.
This seems like a confused question. Do you want to check for specific variants? Or do you want to check a specific gene for lots of variants? Why?
You should be able to do this on the 23andMe website. You probably just haven’t found the right part of it. It might be easier to run it through Promethease for $5. If you want to know about a SNP that 23andMe doesn’t cover, try SNPedia to see if someone else (eg, FamilyTreeDNA) covers it. But probably no one covers it and your best bet is probably an exome sequence for ~$1000. But you’ll still have to analyze it.
I have an inheritable disease linked to the gene that significantly reduces life quality. My fiancée and I would like to check if she has a possible pathological mutation on there as well, to see if it would be safe to have children in a natural way. (It’s autosomal recessive).
Is this a kind of thing I could use 23andMe for? Or are there any other services online?
Really, you should just take up SNPedia’s offer in the other comment and email them.
If you really have a simple Mendelian disease, then people probably know what the mutation is. You need to figure out the name of the variant, not just the name of the gene. SNPedia will have a page about it and that page will link to 23andMe’s page about it. Try searching snpedia for the name of the disease.
If you know what the gene is, you could look it up on snpedia and look at all the variants of that gene, to figure out which one is relevant. If you know the gene, but think that multiple variants might break it, why do you think that? Do you have a paper saying so? Does it lists the names of the variants? If not, you are probably out of luck.
This is all assuming that it is a SNP. But a serious disease is unlikely to be a SNP and more likely to be something else, such as a deletion. 23andMe won’t measure that directly, nor probably anyone else. But there might be a SNP in linkage disequilibrium with the important variant. Learning what that SNP is might be harder.
Feel free to contact us directly. We can look up the disease and check how many mutations in the associated gene(s) are tested by the various direct-to-consumer (DTC) genomics companies (and get a sense for whether deletions that aren’t tested form a significant fraction of the disease burden).
Keep in mind though that you shouldn’t make medical decisions from tests that aren’t clinically validated. Most DTC genomic tests are not, even if the ones from reputable companies usually do replicate well in a technical sense, and, even if they point you in the right direction in terms of seeking appropriate health-care providers.
I have a molecular testing result for my brother who has the same disease. It states the gene and confirms two different pathological variants (presumed to lay on two different chromosomes, as it would be necessary to cause the disease).
Unfortunately I cannot get the same test. It needs to be authorized by my doctor who refuses do to so on the basis that “it’s enough indirect genetic proof” in combination with obvious symptoms.
Even less could I get my fiancée tested through the regular health care system.
But thanks a lot for your input, I will contact SNPedia 🙂
We maintain a Testing webpage that gives you general information on the overall coverage of various direct-to-consumer genomic company products, based on their coverage of SNPedia and of ClinVar.
That doesn’t quite get you information about a specific gene, though. For that, the best thing is to know which variants in a gene you care about, and then to look them up in SNPedia via either their gene page or one-by-one. You’ll see a row towards the bottom of most SNP pages called ‘Categories’, where we try to catch which product by which company normally returns data for that SNP. For example, if you see ‘On chip Ancestry v2’ listed in the Categories section for rs6313, that means that the v2 DNA chip from Ancestry (which is what they’ve been using from May 2016 to the present for their $99 product) normally will return data for this SNP.
Keeping track of this is hard. Most companies don’t share the information readily, and they can change what they’re doing behind the scenes (i.e. which SNPs they are testing) without any public announcement, so although we catch most of the information, it will always be a moving target.
Feel free to contact us directly/privately if you’d like help figuring any of this out for a given variant or gene.
On a related topic why does anyone believe that effective (SSRIs etc.. may be better than nothing but I wouldn’t call them effective) antidepressants that aren’t habit forming or enjoyable for the non-depressed should exist at all?
I mean being happy feels good. When there is a specific injury or disease causing agent we can develop treatments that just fix what’s broken but don’t affect people without the injury/disease. However, when we see continuous effects (like being extremely short, building musculator very easily, having low bone density) especially when they seem to be an extreme instance of natural variation we almost never find treatments that only affect those doing worse than average. Indeed, it would be astonishing if we found a drug that only made people below 5’5″ taller but not those above 6′ so why do we expect this for depression?
Now maybe we don’t but people just aren’t willing to contemplate widespread use of mildly enjoyable drugs even if engineered to avoid the problems of euphorics generally.
Tylenol can treat pain in people who have it, but doesn’t increase pleasure in people who have no pain to begin with. Why can’t antidepressants be like Tylenol?
(I don’t personally believe this, but some people think that SSRI emotional blunting is part of the activate effect – it removes ability to care much about emotions, which helps treat depression but is bad if your emotions are sometimes good)
It’s certainly true that the subjective experience of being on an antidepressant that is working does not at all feel like being on a pill that makes you happy. At best, it feels like it is removing a block that keeps you in the ‘depressed all the time’ end of the spectrum and gives access to a fuller range of emotions that includes happiness. It won’t make you happy in its own, except for in the trivial sense where there’s often a time when the fact that something finally seems to be working is a cause for celebration (this effect doesn’t seem to track with dosage or differ noticeably from other instances in which someone has finally found a thing that seems to be helping with a problem they have been struggling with).
Scott’s tylenol analogy works for me actually, not on any biochemical level I’m sure, but in terms of how the symptom ideally responds to the drug. Like, tylenol can reduce a fever but that’s not the same as it being a temperature-reducing drug that will put your body temperature below normal if you take it when you don’t have a fever. Similarly, antidepressants, when they work, are depression-reducing drugs not happiness-increasing drugs, and will not increase happiness in the absence of depression.
(Well. Unless they’re inducing mania. But that is quite clearly a different sort of thing to just an increased level of happiness, and happens at a wildly different timescale and intensity than the drug’s intended mood-lifting effect.)
(And yes another one for team antidepressants do sometimes work, because for fear of sounding like a clinical-experience-using fogie shying away from evidence-based-medicine, I have just felt and seen them work far too often. Something like genetics accounting for the thing where a given drug works for a given person seemingly at random seems plausible.)
I don’t think genetics is all there is to it. My wife did very well on nothing but Prozac for many years, but after the kids were born it just stopped working.
They do do that. @Scott do pharmacogenomics guys think genetics accounts for the thing where drugs sometimes just stop working for some people but not others? Anecdotally I feel like there seem to be people with a pattern of ‘everything eventually stops working’ and people who don’t experience pooping out at all more than there are people who get it like, one time with one drug.
I’m not remotely sold on the benefits of genetic testing, but:
Assuming (for the sake of argument) that the test actually works and produces relatively clear-cut results, and is something that a lab can process in a week or so (which are both rather wild assumptions, but such are the sacrifices we must make for a good argument in these philosophical times):
Situation A:
I’m a clinically depressed patient. Unbeknownst to me, I have the ultrarapid mutation. I don’t know that, since it’s unbeknownst. All I really know is that I’m depressed as shit. I go to my psychiatrist – a thoroughly average man within the confines of the profession – who decides to start me on 10mg Prozac.
The remaining week is a fog. My girlfriend is increasingly sick of her lethargic, uninterested, boring shitlump of a partner. My boss is not terribly impressed with my lethargic, apathetic performance. My friends ask me if I want to go out. I decline.
Next week, I go back. He asks me how I feel. I don’t feel much better. Do I feel worse? No. Hmm. That’s interesting. Now, my psychiatrist is a very average man, about as risk-adverse as the rest of the medical profession. He keeps me at 10mg for now, let’s give it time to ramp up or whatever.
The remaining week is a fog. My girlfriend decides to pull some extra shifts at work, since she could use the money and honestly doesn’t really fancy the thought of suffering through nights with her wet blanket partner. My boss yells at me for missing my monthly deliverables. I’m too depressed to really offer more than a muttered “yeah, sorry”. One of my friends calls me in the middle of a minor life crisis. I’m too depressed to really think of anything beyond ending the conversation as quickly as possible.
Next week, I go back. How am I feeling? Well, not better. Let’s try upping me to 20mg.
The remaining week is a fog. Girlfriend decides to take a vacation in the Cayman islands – she has extra cash and she’s always wanted to go and it’s so cold here and, you know, not that she’d actually say it, but just being around me and these surroundings kinda put her in the doldrums. She doesn’t exactly invite me and, to my credit, I don’t exactly push to be invited either. Boss is increasingly sick of what he sees as a mediocre individual with an apathetic attitude. Some friend pings me on Facebook, I can’t bring myself to really offer much in the way of a reply.
Next week, no better, well, hmm, let’s bump you up to 40mg.
Remaining week fog. Girlfriend Cayman. Boss angry. Friends absentia.
No better. No Prozac. Now Zoloft.
Next week seems a bit less foggy than the previous several. For the first time in months I really feel like I could have an intimate cuddle with a significant other while watching some movie or other. Of course, just as I’m thinking that, my phone buzzes, my girlfriend happened to bump into one of her friend-of-friends in the Caymans, who introduced her to her brother, brother is a dreamy hunk who my girlfriend always kindasorta had a thing for, it’s a tropical paradise, one thing leads to another, long story short, she’ll be by sometime next week to collect her things, still friends, yeah? I try reaching out to a friend, but I get that slightly-impersonal “hey, can you call back later, right now I’m kinda busy” vibe. I stagger into work and my boss informs me that there’s going to be a meeting. One of “those” meetings. You know, the ones that involve the boss, a member of H.R., and one “lucky” employee. I want to kill myself, but on the bright side, at least it’s due to external factors that an unbiased outsider would probably understand, rather than just the usual depression shit, so, uh, progress?
But at least we know that Zoloft maybe possibly works!
Situation B:
I’m a clinically depressed patient. Unbeknownst to me, I have the ultrarapid mutation. I don’t know that, since it’s unbeknownst. All I really know is that I’m depressed as shit. I go to my psychiatrist – an unassuming Hero Psychiatrist whose initials happen to be “SA”. He has his on-site phlebotomist draw some blood, and then tells me a story about some people who take pills of various colors. I’m too depressed to really fully follow, but I gather that he ended the story on a bit of a cliffhanger. Ho hum.
The remaining week is a fog. My girlfriend is increasingly sick of her lethargic, uninterested, boring shitlump of a partner. My boss is not terribly impressed with my lethargic, apathetic performance. My friends ask me if I want to go out. I decline.
Next week, I go back. My Hero Psychiatrist greets me with a charismatic handshake. My DNA test results are in and, based on them, he plans to start me on Zoloft. He tells me a bit more about the people-pill-color story, and off I go.
The remaining week is a bit less foggy. My girlfriend decides to pull some extra shifts at work, but only a couple, as we have a rather passionate heart-to-heart – and arm-to-shoulder – and yangus-to-yingus – Hump Day rendezvous while watching some forgettable movie together. My boss yells at me for missing my monthly deliverables. I manage to channel just enough of my bullshit reservoir to emphatically apologize and promise to do better, while making a mental note to update my LinkedIn ASAP. One of my friends calls me in the middle of a minor life crisis. I’m a bit worn-out, but manage to offer a compassionate ear and friendly perspective.
The next week, my Hero Psychiatrist decides to bump me to 20mg, since there were no adverse side effects and 20mg is his standard dose. We have a very animated conversation about pills – namely, why would anyone willingly choose the Green pill over any of the others?
———-
The bottom line here is that, no matter how many trite reminders are given, I think very few of those in the medical profession truly internalize how things are for the patient. Even if it were patently obvious to a patient when a medication was working (and in reality it isn’t obvious!), a patient still can’t just switch medications on their own time – which is part of why self-medication is such a popular option. Each little bit of titration carries an opportunity cost of time, and life can’t be put on pause while we tinker through the trial-and-error.
Obviously, this doesn’t exactly matter, given that the current results from genetic testing seem to be of iffy merit at best – but the question was “why would [a perfect, clear-cut test] be any better?” and the answer is “the same reason you rinse your skinned knee with the stingy green soap in the nurse’s office right away, rather than waiting for it to turn funny colors and start oozing fluids”.
An a16z podcast on this topic just popped up in my feed – definitely more of an optimistic introductory piece though. it was interesting listening to it after being primed for scepticism by this post.
Anyway I think many people who read this blog may find this podcast enjoyable since there is a slightly overlapping cultural focus. Among other things they recently had Tyler Cowen on to talk about his book. The podcast is run by guys who work for the well known VC firm Andreessen Horowitz and tends to do a good job introducing me to tech subjects I wasn’t very aware of.
http://a16z.com/podcasts/
All of the above are what I would expect assuming current knowledge and technology, regardless of whether pharmacogenetics is a viable strategy.
Say this approach is viable, and eventually pans out. You’re working in the field 50 years from now, reviewing some genetics results while awaiting a lecture titled, “Phamacogenetics: Past perspectives and lessons learned”. You look over a dozen or so patients and notice the following:
1. A patient has a strong activating mutation for a cytochrome that metabolyzes Prozac. Recommend against Prozac.
2. A patient has a mutation that suppresses an inhibitor of the cytochrome mentioned above. Recommend against Prozac.
3. A patient has extra genomic copies of an miRNA that suppresses the cytochrome (or inhibitor) above. Transcription analysis reveals this miRNA is more active than in the general population. Recommend against Prozac.
4. A patient has a mutation in the histone modifier that enhances/decreases expression of genes from patient 1, 2, or 3 above. Recommend against Prozac.
5. A patient has a mutation that reduces binding of Prozac to SERT. Recommend an increased starting dose.
6. A patient has the histone modification from #4 above, but you notice that this patient also has lower blood brain barrier permeability to Prozac due to a rare SNP in a connexon gene. The first mutation increases the effective dose of Prozac in the blood stream, but the second mutation decreases the effective dose in the CSF. Recommend higher starting dose, but be vigilant for extra-cranial side-effects.
7-12. Patients have multiple combinations of the above; some are recommended against Prozac, while others in favor – possibly with special instructions. A combination of mutations that both increase and decrease Prozac efficacy produce an emergent effect that is not readily identifiable by looking at each gene in isolation. This represents a majority of your patients, while patients 1-6 – with only one or two individual differences are rare.
The lecture begins. The presenter’s first slide is nothing but the titles of analyses and meta-analyses published in the last five years. “Initial efforts at identifying pharmacogenetics targets focused on identifying single targets at a population level.” Everyone laughs.
The presentation continues, “The absurdity of doing population-level statistics on diverse patients whose responses are all multi-factorial seems obvious to us today. However it’s important for us to remember that before 2020 nearly ALL scientific data were based on population-level statistical inferences, both at the bench at in the clinic. This approach worked well enough for a century of medicine before the advent of the personalized approach. So it was natural there would be a rough transition period. This was a time where physicians tried to take personalized patient information and apply population-level lessons (without adequately factoring in what seem to us now to be obvious individual confounders). Or, equally troubling, scientists tried to take multiple individual – and limited, remember they didn’t have universal whole-genome/biome/epigenetic analysis – sequences and try to identify population-level effects of single mutations. They worked backward from positive signals in limited testing and blindly hoped all the targets they didn’t test for wouldn’t matter as much as those few they did test.
“It’s easy for us to look back in hindsight and ask, ‘What were they thinking? They should have been able to intuit that the effects they were looking for were distributed among multiple polymorphisms, and that no two patients would share the same genetic profile.’ But there is a more important lesson to be learned here. We have often heard the aphorism, ‘The plural of anecdote is not statistic.’ It is vital that we also learn the reverse corollary, ‘The singular of statistic is not patient.'”
This seems to be your claim regarding genetics :
I think it is too strong. Medicine utilizes dozens of scales and algorithms that take into account multiple disparate risk factors and/or measurables. They are based on statistics, population-level observations, and rational pathophysiology. None of these is perfect, but they are good enough to be useful practices. It is entirely plausible that genetic polymorphisms will be amenable to similar methods, IE, once we know enough about them, they can be organized algorithmically.
Moreover, this is the sequence of events in your brief story :
1. Physicians employ successive therapeutic trials, relatively indiscriminately, based on very broad population-level statistics.
2. Unsatisfied, physicians try to refine their approach by examining genetic polymorphisms
3. Genetic polymorphisms don’t pan out because population-level statistics don’t work
4. Everyone has a good laugh at how silly it is to use population-level statistics
5. Having failed in attempts to refine their approach, physicians employ successive therapeutic trials, relatively indiscriminately, based on very broad population-level statistics
What, exactly, are you trying to say about population-level statistics? Do they apply to everything except genetics? What do you propose as an alternative for the foundation of medical praxis?
Great post. I too get a little bit sick of all the genomics-hype. Obviously there is something there, so it can’t be dismissed as easily as homeopathy, though we still need to be skeptical.
That said, Ionnadis quote is a bit too far. I work in cancer genomics, specifically in the use of genetic testing to guide treatment. This area definitely has shown results. The earliest targeted cancer therapies were approved in the late 90s (trastuzumab, gefitinib, imatinib) and they work really well. The genomics revolution has produced a lot of hype and also a lot of potential; if a drug is known to be (in)effective in patients with a loss-of-function mutation A in gene X, it’s also likely that drug is (in)effective for a different loss-of-function mutation B in the same gene X, we don’t need an RCT to say so.
The fact that next-generation sequencing means we can test large sections of the genome (rather than one mutation at a time) makes it much easier to discover actionable mutations; common clinical tests look at the most common cancer genes and are able to detect any mutation contained therein. Plus NGS makes possible a new biomarker, the total mutational burden found in a tumor. Sounds like a somewhat random number, but it’s a good predictor of response to immunotherapy (http://science.sciencemag.org/content/348/6230/124).
You asked what’s different between a) genetic testing for the best anti-depressant and b) guess-and-check? In that case, not much. If the patient has cancer, the cost of wrong/ineffective therapy is that the tumor grows for weeks or months while the patient still experiences all the negative side-effects, so the “therapy” is doing more harm than good.
tl;dr Cancer genomics matter
Has anyone looked at transport across the blood-brain barrier? If CNS levels are thought to be related to efficacy, and plasma levels are demonstrably not, then there may be deficient transport into the CNS.
Maybe those mechanisms are saturable?
…I’ve had terrible antidepressant side effects. It sucks. Your example is worse than a (functional) genetic test because your example involves a patient (who, given their, you know, depression, is probably suffering already) having really sucky symptoms as a result of a drug you gave them. If that wasn’t bad enough, their trust in psychiatry to help them has been eroded by the experience. When Escitalopram messed me up real good, I didn’t try something else, I freaked out and stopped messing around with antidepressants entirely and I’m only now finally trying again. That was years ago, and I’d done my reading on how I would probably react completely differently (and likely better) to some other antidepressant. I just didn’t care any more.
I feel slightly ridiculous pointing this out, like I’ve missed a joke or something.
You wrote:
Or suppose I have a patient with a mutation that makes them an ultrarapid metabolizer; no matter how much Prozac I give them, zero percent ever reaches their brain. I start them on Prozac 10 mg, nothing happens, go up to 20, then 40, then 60, then 80, nothing happens, finally I say “Screw this” and switch them to Zoloft. Once again, how is this worse than the genetic test?
Well, how many months does it take before you get them on Zoloft? that Wisconsin test was “success at 8 weeks”. W/o the pharmacogenomic data, I would guess that in a lot of cases at 8 weeks you would still be hunting for the right dose / drug.
As for John Ioannidis, we’ve learned a hell of a lot since May of 2013. Relying on a paper from then about what we can do now is roughly the same as making statements about how useless computers are in March 1997, based on data from May 1993.
Or, maybe, dismissing talk about what can be done with Desktop Publishing in March of 1987, based on a paper on the subject written in May of 1983.
In section V, re: how is the genetic test better than the clinical method, I think there’s a clear answer.
Trying a bunch of different antidepressants until you find one that works is, from the patient’s point of view, expensive, varying degrees of unpleasant depending on the side effects, demoralising (prompting some people to give up on finding a treatment, especially given that they are already depressed), and it takes months – time in which a patient is continuing to live with untreated-ish depression and at risk of hard-to-reverse complications like blowing up their jobs and relationships, self-harm and suicide.
So if it worked, it would be very good. I don’t know how much money it would save because I don’t know the cost of the test versus all the doctor’s appointments and trial prescriptions, but it would save a lot of time and unpleasantness. ADs are a drug where this would be particularly useful because of how long it takes to figure out if a given drug or dose is doing anything.
Also it seems very likely that trial and error diagnosing will settle on the first drug/dosage that’s good enough, which means it may never find the ideal drug/dosage for most patients.