I have frequently heard people cite John Ioannidis’ apparent claim that “90% of medical research is false”.
I think John Ioannidis is a brilliant person and I love his work and I think this statement points at a correct and important insight. But as phrased, I think this particular formulation when not paired with any caveats creates just a little more panic than is warranted.
Before I go further, Ioannidis’ evidence:
He starts with simple statistics. Most studies are judged to have “discovered” a result if they reach p < 0.05, that is, if there is 5% probability or less the findings are due to mere chance (this is the best case scenario, where the study is totally free from bias or methodological flaws).
Suppose you throw a dart at the Big Chart O’ Human Metabolic Pathways and supplement your experimental group with the chemical you hit. Then ten years later you come back and see how many of them died of heart attacks.
Most chemicals on the Big Chart probably don’t prevent heart attacks. Let’s say only one in a thousand do. Maybe your study will successfully find that 1/1000. But the 999 inactive chemicals will also throw up about 50 (999 * 5%) false positives significant at the 5% level. Therefore, even if you conduct your study perfectly, and it shows a significant decrease in heart attacks, there’s about a 98% chance it’s false.
One would hope medical scientists plan their studies with a little more care than throwing a dart at a metabolic chart. Yet many don’t; a lot of genetic research is conducted by checking every single gene against the characteristic of interest and seeing if any stick. And even when scientists have well-thought out theories, the inherent difficulty of medicine means they probably have less than a 50-50 chance of being right the first time, which means a 5% significance level has a less than 5% predictive value.
And this isn’t even counting publication bias or poor methodology or conflicts of interest or anything like that.
Disturbingly, this problem seems to be borne out in empirical tests. Amgen Pharmaceuticals says it repeated experiments in 53 important papers and was only able to confirm 6. And Ioannidis himself did a re-analysis which is quoted as finding that “41% of the most influential studies in medicine have been convincingly shown to be wrong or significantly exaggerated.”
So I don’t at all disagree with the general consensus that this is a huge problem. But I do disagree with the following statements:
1. 90% of all medical research is wrong
2. A given study you read, or your doctor reads, is 90% likely to be wrong.
3. 90% of the things doctors believe, presumably based on these medical findings, is wrong.
4. This proves the medical establishment is clueless and hopelessly irrational and that two smart people working in a basement for five minutes can discover a new medical science far better than what all doctors could have produced in seventy years.
Is 90% of all medical research wrong?
As far as I can tell, there is no source at all for the 90% figure. I can’t find it in any of Ioannidis’ studies and indeed they contradict it. His table of predictive values of different studies doesn’t have any entries that correspond to 90% (“underpowered exploratory epidemiological study” is relatively close with 88%, but this is just for that one type of study, which is known to be especially bad). The Atlantic sums it up as:
His model predicted, in different fields of medical research, rates of wrongness roughly corresponding to the observed rates at which findings were later convincingly refuted: 80 percent of non-randomized studies (by far the most common type) turn out to be wrong, as do 25 percent of supposedly gold-standard randomized trials, and as much as 10 percent of the platinum-standard large randomized trials.
Notice which number is conspicuously missing from that excerpt.
Now another study of his did show that in 90% of studies with very large effect sizes, later research eventually found the effect size to be smaller, but this was out of a pool of studies specifically selected for being surprising and likely to be false. I don’t think it’s the source of the number and if it were that would be terrible.
As far as I can tell, this started from a quote in an Atlantic article on Ioannidis which included the line “he charges that as much as 90 percent of the published medical information that doctors rely on is flawed”. This then got turned into the title of a Time article “A Researcher’s Claim: 90% of Medical Research Is Wrong”, which itself got perverted to 90% of Medical Research Is Completely False.
So an unsourced quote that up to 90% of studies are flawed has somehow turned into a rallying cry that it has been proven that at least 90% of studies are false. To take this seriously we would have to believe that the numbers for all research are the same as the numbers for the poorly conducted epidemiological studies or the studies specifically selected for surprising results. I guess having a nice round number is good insofar as it makes the public pay attention to this field, but as far as actual numbers go, it’s kind of made up.
Is any given study you read, or your doctor reads, 90% likely to be wrong?
But let’s take the above number at face value and say that 90% of medical studies are wrong. Fine. Does that mean the last medical study you read about in Scientific American, or that your doctor used to recommend you a new drug, is wrong?
No. Let’s look at the Medical Evidence Pyramid.
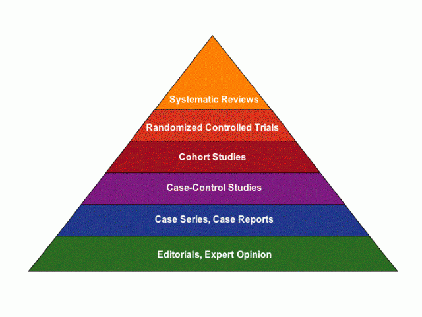
This plays out in the same table of Ioannidis’ speculations we saw before. While an in vitro study of the type used to identify possible drug targets might have a positive predictive value of 0.1%, a good meta-analysis or great RCT has a positive predictive value of 85%; that is, it’s 85% likely to be true.
There are only two reasons someone might hear about the studies on the snake-infested bottom levels of the pyramid. Number one, that person is a specialist in the field who is valiantly trying to read through the entire niche medical journal the paper was published in. Or number two, the study found something incredible like DONUTS CURE CANCER IN A SAMPLE OF THREE LAB RATS!!! and the media decided to pick up on it. Hopefully everyone already ignores studies of the DONUTS CURE CANCER IN A SAMPLE OF THREE LAB RATES!!! type studies; if not, there’s really not much I can say to you.
But most of the medical results that you hear about are the ones that get published in important journals and are trumpeted far and wide as important medical results. These are closer to the top of the pyramid than to the bottom. They’re usually big expensive studies on thousands of people. Since the universities, hospitals, and corporations sponsoring them aren’t idiots, they usually hire a decent statistician or two to make sure that they don’t spend $300,000 testing something only to have a letter to the editor of the NEJM point out that they forgot to blind their subjects so it’s totally worthless. And finally, in many cases you would only run a study that big and expensive if you had something plausible to test – you’re not going to spend $300,000 just to throw a dart at the Big Chart O’ Human Metabolic Pathways and see what happens.
So these studies that people actually hear about are bigger, they have more incentives to get their methodology right, and they’re testing propositions with high plausibility. How do they do?
I said above that one of Ioannidis’ studies was frequently quoted as saying that “41% of the most influential studies in medicine have been convincingly shown to be wrong or significantly exaggerated.”
This is from a great study I totally endorse, but the 41% number was maximized for scariness. If I wanted to bias my reporting the other direction, I could equally well report the same results as “Only about 5% of influential medical experiments with adequate sample size have later been contradicted.”
How? Ioannidis got his result by taking all medical studies with over 1000 citations in the ’90s, of which there were 49. Of these, 4 were negative results (ie “X doesn’t work”) so he threw them out. This is the first part I think is kind of unfair. Yes, negative results aren’t as sexy as positive results, but they’re still influential medical research, and if Ioannidis is quoted as saying that X% of medical findings are later contradicted when he means that X% of positive medical findings are, that’s not quite fair.
Annnnyway, of the 45 famous studies with positive findings, 11 didn’t really get tested and so we don’t know if they’re right or wrong. Eliminating these is also a potential bias, because we expect that studies which seem sketchy are more likely to be replicated so people can find out if they’re actually right. Ioannidis quite rightly set himself a higher bar by not eliminating them, but the quote about 41% of studies being wrong does seem to have gone back eliminated them – at least that’s the only way I can make the study numbers add up to 41% (the numbers given in the study actually say 32% of these studies failed to replicate).
So our 41% number is based off of 34 studies, best described as “34 famous medical studies that found positive findings ie the least believable kind of finding, plus were suspicious enough that someone wanted to replicate them”.
Of these 34 studies, 7 were outright contradicted. Bad? Definitely. But for example, one of them was a study with a sample size of nine patients. Another study may well have been correct, but the results were interpreted wrongly (it said that estrogen decreased lipoprotein levels which everyone assumed meant decreased heart disease, but in fact later studies found increased heart disease without necessarily disproving the lipoprotein levels). Five of the six others were epidemiological trials, firmly on the middle of the pyramid. Only two of these contradicted studies were a true experiment with a sample size of >10.
(even here, I am sort of skeptical. Three of these disproven studies, two epidemiologicals and an experimental, purported to show Vitamin E decreased heart disease. Then a single better trial showed that Vitamin E did not decrease heart disease. While recognizing the last trial was better, it does seem like something more complicated is going on here than “all three of the earlier trials were just wrong”, and I’ve recently been convinced antioxidant research is a huge minefield where tiny differences in protocol can cause big differences in results. But fine, let’s grant this one and say there were two outright-contradicted experiments.)
So aside from the seven that were outright wrong, another seven were listed as “overstating their results”.
There are a couple of problems that bothered me here. One of them was that Ioannidis decided to count studies as contradicting each other if relative risk in one study was half or less than in the other study, “regardless of whether confidence intervals might overlap or not”. So even if a study effectively said “Here is a wide range of possible results, we think it’s about here in the middle but our research is consistent with it being anywhere in this range”, if another study got somewhere else in that range, the first study was marked as “exaggerated”.
The second problem is, once again, poor studies versus poor interpretations. Ioannidis cites as an example of an exaggerated study one lasting a year and showing that the drug zidovudine helped slow the progression of HIV to AIDS. It concluded that giving HIV patients long-term zidovudine was probably a good idea. A later study lasted longer, and said that yes, zidovudine worked for a year, but then it stopped working. Because the earlier study had suggested longer-term zidovudine, it was marked as “exaggerated results”, even though the results of both studies were totally consistent with one another (both found that zidovudine worked for the first year). This is probably of little consolation to AIDS patients who were treated with a useless drug, but it seems pretty important if we’re investigating study methodology.
So the way I got my 5% figure was to take the two experimental studies with decent sample sizes which were actually contradicted and compare them to the 38 large experimental studies total that started the experiment.
So this suggests that if you see a large experimental study being trumpeted in the medical literature, the chance that it will be found to be totally false (as opposed to true but exaggerated) within ten years or so is only about 5% – which if you understand p-values is about what you should have believed already.
(I think. This requires quite a few assumptions, not the least of which is that my calculations above are correct!)
Also worth noting: Ioannidis’ experiment did not investigate the absolute highest level of the medical pyramid, systematic reviews and meta-analyses. I expect the best of these to be better than any individual study.
3. Are 90% of the things doctors believe, presumably based on medical findings, wrong?
After going through the steps above, it should be pretty obvious that the answer is no, because doctors are mostly reading famous influential studies like the ones mentioned above, which are at worst 40% and at best 5% wrong.
But there’s another factor to be taken into account, which is that why would you only read one study on something when lots of important findings have been investigated multiple times?
Suppose that you’re throwing darts at the Big Chart O’ Human Metabolic Pathways, with your 1/1000 base rate of true hypotheses. You run a very good methodologically sound study and find p = .05. But now there’s still only a 1/50 chance your hypothesis is correct.
But another team in China runs the same study, and they also find p = .05. We expect the Chinese to get false to true results at a rate of one to two (because the 1 in the 1/50 stays 1, but the 50 is divided by 20 to produce approximately 2. Wow, I’m even worse at explaining math than I am at doing it.)
Now a team in, oh, let’s say Turkey runs the same study, and they also find p = .05. We expect the Turks to get false to true results at a rate of one to ten, for, uh, the same math reasons as the Chinese. When the, um, Icelanders repeat the study, our odds go to one to two hundred.
So we started with 1000:1 odds, the first study brought us up to 50:1 odds, the second study to 2:1 odds, the third study to 1:10 odds, and the fourth study to 1:200 odds, ie we are now 99.5% sure we’re right.
Real medicine is both better and worse than this. It’s better in that we often have dozens of studies rather than just four. It’s worse in that the studies are not all so methodologically sound that we can multiply our odds by 20 each time (to put it lightly).
But some of them are, and once we get enough of them, the base rate problems which plague individual medical findings go away very quickly. Even if only one of the studies is methodologically sound, if the reason they’re studying their topic is because a bunch of other less believable studies all got positive results, that’s a much better base rate than “because I hit it with my dart”.
When doctors say that, for example, iron supplements help anaemia, it’s not because they hit iron on their Big Chart O’ Human Metabolic Pathways, then ran a single study, got p = .05, and rushed off to publish a medical textbook. It’s because they knew hemoglobin had iron in it, there are at least 21 randomized controlled studies, probably some had p-values closer to .001 than to .05 even though I don’t have any of them in front of me to check, and eventually some really really smart statisticians at the Cochrane Collaboration gave it their seal of approval. Most doctors’ beliefs aren’t on quite this high a level, but most doctors’ beliefs aren’t on the “Someone threw a dart, then did one study” level either.
4. Does this prove the medical establishment is clueless and hopelessly irrational and that two smart people working in a basement for five minutes can discover a new medical science far better than what all doctors could have produced in seventy years?
A lot of people seem to go from Ioannidis’ experiment to something like “So I guess everyone in medicine is just clueless about how science and statistics work. I’ll go read a couple of medical studies and then be able to outperform everyone in this totally flawed field.”
(important note: I’m not accusing MetaMed of this! They seem pretty sane. I am accusing some people I come across in the community who are much more enthusiastic than the relatively sober MetaMed people of doing something like this.)
But the problem isn’t that no one in medicine is familiar with Ioannidis’ research. It’s that they’re not really sure what to do about it and figuring out a plan and implementing it will take time and effort.
Ioannidis’ work isn’t exactly secret. I’ve hung out with groups of residents (ie trainee doctors) who have discussed Ioannidis’ findings over the dinner table. According to The Atlantic
To say that Ioannidis’s work has been embraced would be an understatement. His PLoS Medicine paper is the most downloaded in the journal’s history, and it’s not even Ioannidis’s most-cited work—that would be a paper he published in Nature Genetics on the problems with gene-link studies. Other researchers are eager to work with him: he has published papers with 1,328 different co-authors at 538 institutions in 43 countries, he says. Last year he received, by his estimate, invitations to speak at 1,000 conferences and institutions around the world, and he was accepting an average of about five invitations a month until a case last year of excessive-travel-induced vertigo led him to cut back.
So if so many people are aware of this, why isn’t the problem getting fixed more quickly?
An optimist could say the problem isn’t getting fixed because there is no problem. A vast volume of embarassingly wrong medical literature gets published, inflates the publishers’ resumes, and everyone else ignores it and concentrates on the not-really-so-bad large randomized trials. To the post-cynic it is all a smooth, well-functioning machine.
A pessimist might say that the problem isn’t getting fixed because it’s impossible. The average medical hypothesis is always going to have a low base rate of being true – in fact, if we force scientists to only study high base-rate hypotheses, by definition everything we discover will be boring. There will never be enough resources to apply huge rigorous trials to every one of the millions of things worth studying. So we’re always going to have weak studies about low-base rate hypotheses, which is what Ioannidis is attacking as the recipe for failure.
A realist might point out there are some things we can do, but it involves coordinating a huge and complicated system with many moving parts. Journals can force trials to register before they conduct their experiments to avoid publication bias. The scientific community can give more status to people who perform important replications and especially important negative replications. Study authors and the media can come up with better ways to report their results to doctors and the public without blowing them out of proportion. Statisticians can…actually, anything I say statisticians can do is just going to be a mysterious answer, along the lines of “do better statistics stuff”, so I’m not going to embarass myself by completing this sentence except to postulate that I’ll bet there’s some recommendation that could complete it usefully.
But all these things involve vague entities who aren’t really actors (“the scientific community”, “the media”) acting in ways that are kind of against their immediate incentives. This is hard to make people do and usually involves a lot of grassroots coordination effort. Which is going on. But it takes time.
But no matter what happens, I think a useful epistemic habit is to be very skeptical of individual studies, and skeptical but not too skeptical of large randomized trials, good meta-analyses, and general medical consensus when supported by an evidence base.
> It’s only after you reach the top few levels that you get the gold and jewels and precious, precious mummy powder. This plays out in the same table of Ioannides’ speculations we saw before.
The hyperlink in both seems to be the same.
> Also worth noting: Ioannides’ experiment did not investigate the absolute highest level of the medical pyramid, systematic reviews and meta-analyses. I expect the best of these to be better than any individual study.
Isn’t that covered in http://www.plosmedicine.org/article/fetchObject.action?uri=info:doi/10.1371/journal.pmed.0020124.t004&representation=PNG_M ? Second row, ‘Confirmatory meta-analysis of good-quality RCTs’, PPV=0.85
(Hm, I wonder why that meta-analysis has the same PPV as a ‘Adequately powered RCT with little bias and 1:1 pre-study odds’… Maybe adequately powered here means a study sample size equivalent to that of meta-analyses pooling many underpowered studies.)
Speaking of which, I can’t believe I missed that chart while reading that paper originally. That changes everything: it is our priors for medical research!
> After going through the steps above, it should be pretty obvious that the answer is no, because doctors are mostly reading famous influential studies like the ones mentioned above, which are at worst 40% and at best 5% wrong.
I don’t think this is obvious at all. If doctors did not take a step without consulting a Cochran Review, then yeah, any individual therapy or treatment will have that nice 5-40% chance of being wrong. But is that the case? I was under the impression that the evidence-based medicine folks had made lists and examined old standard treatments with actual RCTs and found that many treatments or medicines had never been genuinely tested, and when they were, often failed.
Table link fixed.
When I said he doesn’t include meta-analyses, I meant in that particular study of the top 49 most cited medical studies. I agree he certainly considers them from a theoretical perspective.
“I was under the impression that the evidence-based medicine folks had made lists and examined old standard treatments with actual RCTs and found that many treatments or medicines had never been genuinely tested, and when they were, often failed.”
I am under the impression that most medicine now is evidence-based medicine.
> I am under the impression that most medicine now is evidence-based medicine.
One would certainly like to think that, but given the long track record of medicine, it’s not something I would believe until I saw a paper asserting that the majority of operations performed in some sample had evidence-based medicine backing…
Is that itself a medical claim? An EBM claim?
How did you conclude this?
I really really think you should talk with some of the doctors MetaMed works with about this. I certainly don’t think anu of them agree
Side note: How many times has MetaMed changed its name?
I mean, they seem like smart people and the basic idea is good, but constant renames is one of those classic signs of a Silicon Valley startup that has no idea what they’re doing and is stuck bikeshedding–it doesn’t give me a particularly good feeling about it.
They’ve had legitimate reasons for most of the times they’ve changed their name, and they’re not really public yet so it doesn’t really count.
1. I, for one, don’t go around claiming that 90% of research studies are false; I believe Ioannides only found a little more than half of medical studies were disconfirmed by later experiments. 90% of all *pre-clinical cancer studies* are later disconfirmed, which does mean that if you see a study that says “X cures cancer” but X hasn’t made it to clinical trials yet, X probably *doesn’t* cure cancer.
2. I don’t expect most things doctors believe to be wrong. I don’t even expect most things *people* believe to be wrong; after all, most things people believe are of the form “water is wet,” so uncontroversial that we barely notice them as beliefs. What I *do* expect to be wrong are beliefs that aren’t based on a model of the world.
“Iron supplements cure anemia” is a belief that depends on knowing how hemoglobin works, knowing how digestion works, having observed iron supplements cure anemia…lots of different kinds of evidence, at different scales of complexity, confirm the prediction.
“Antioxidants reduce cancer risk” is an example of the kind of belief we should be skeptical about. Free radical damage may lead to cancer; antioxidants stabilize free radicals; so one might think antioxidants prevent cancer. Some early clinical trials found that taking antioxidants reduced cancer risk; but further study found that they probably don’t. And there’s some evidence that “free radicals” (or reactive oxygen species) are the mechanism by which the immune system and chemotherapy drugs attack cancers, so antioxidants, if anything, protect cancer cells. We don’t have a clear model of what antioxidants do, in the same way that we have a clear model of what role iron plays in the blood, so we ought to be skeptical of any conclusions about “antioxidants are good for you” or “antioxidants are bad for you.” And we ought to be *especially* skeptical of anything that assumes a cause (eating antioxidants) will result in a health outcome (less cancer) merely because the cause affects an intermediate biochemical step (stabilizing free radicals).
If there’s a prevailing theory in the biomedical sciences that a.) relies on complex chains of genetic and biochemical causation, and b.) hasn’t shown measurable results in the form of lower death rates, then I’m going to tag it as improbable.
1. I didn’t mean to “accuse” you of saying this. I meant other, less reputable sources like Time Magazine and most of the rest of the media.
2. I’m not sure to what degree I agree with your emphasis on understanding mechanism. There are a lot of things that work in biology without us having any idea how. Natural selection was a pretty good example until we discovered genes. Another good example would be digitalis, which was used to treat heart failure since the 1700s but whose full mechanism of action was only discovered in the last few decades. I prefer good experimental results to good theoretical explanation, but the caveat which I think you’re trying to point out is that they had better be *good* experimental results, as opposed to marginal experimental results.
3. The last part wasn’t meant as an attack on MetaMed, just on some of the people who try to talk about this on Less Wrong. I’ve edited the post to try to make this slightly clearer.
A defense of MetaMed:
1. We are not a Silicon Valley startup, we aren’t even based in Silicon Valley, we don’t belong to that culture, we’re not a consumer web app, there’s really no sense in which that’s the right reference class.
2. We are only doing a major publicity launch at the end of this *month*, so name changes are not really a practical issue. The name changes were in response to market research — people are bad at intuiting what kinds of names appeal to customers, and we finally settled on MetaMed after our marketing team put a lot of effort into finding out what made the best impression. It’s just window dressing; the internal structure of the company has stayed the same.
3. It’s useless to promise we can fix a hard problem until we succeed in fixing it. But we’re not claiming to be able to waltz in and fix medicine with no effort. One of the main things we’re doing is a bit more modest: just *assembling* a coherent model out of the research that already exists.
We know, for example, that statistical prediction rules work very well for medical diagnosis and risk prediction; these are just simple little combinations of a few known risk factors for, say, heart attacks, that give each patient a score rating their hart attack risk. But these rules only exist for a few special cases in medicine. For most diseases, nobody has gone around combining all the risk factors and all the signs and symptoms into a single statistical model that says “if we know X, Y, and Z about you, here’s how likely you are to have disease A.”
One way of looking at MetaMed’s job is that we combine, reorganize, and quantify the existing scientific literature.
Now, in a sense, you could say that medical culture already does this; doctors pretty much know, from some combination of their clinical experience, their med school education, and whatever research they have time to read, how to diagnose diseases and choose treatments. But this is, one has to admit, an imperfect process. Human minds are very bad at intuitively putting disparate pieces of information together. That’s *why* things like checklists and statistical prediction rules can outperform clinicians; using intuitive judgment, you’ll forget things. Formally organizing the research literature into prediction models is a kind of safeguard on expert judgment, and I think it’s quite likely to catch things doctors miss.
(1) Statistics are much more complicated than people (even smart, educated, knowledgeable in their field people) think.
(2) Medicine is an art more than a science.
(3) Confusion reigns. For family reasons, I’ve been scouring online resources for information on diabetes diets, and I’m getting confusing recommendations even on the very same website; e.g. carbohydrates increase blood sugar – well and good; starch as well as sugar needs to be watched – fine, tell me more; eat vegetables rather than fruit – okay, what vegetables; eat carrots, they’re low-GI – no, don’t eat carrots, they’re loaded with sugar! Eat peas – no, don’t eat peas, they’re full of starch and starch is bad!
The conclusion I am left with is that the only safe diet (for anything) is rainwater and moss 🙁
This is exactly what MetaMed is for. It’s definitely the case that 1, 2, and 3 are true, but 1 is largely a consequence of accepting too low a standard when seeing people as smatt and knowledgeable
So this suggests that if you see a large experimental study being trumpeted in the medical literature, the chance that it will be found to be totally false (as opposed to true but exaggerated) within ten years or so is only about 5% – which if you understand p-values is about what you should have believed already.
(I think. This requires quite a few assumptions, not the least of which is that my calculations above are correct!)
Not really. P-values are not how likely something is to be wrong or invalid. Rather, they’re how likely this data was to show up if you were wrong, i.e., they’re P(E | not H) rather than P(not H | E). (Except they’re not really that, either — they’re just how likely this data was to show up if you were wrong in a particular way, i.e. the null hypothesis.)
And yes this is very counterintuitive and hence why everybody gets them wrong.
The 5% number comes not from p-values but from the empirical observation that of 40 studies analyzed, 2 were wrong. This matches the number of studies that would be wrong merely by chance if we only took the p-value into account rather than the base rate.
Yes, but you say that the empirical 5% matches what you’d expect from a p-value of 5%, and I don’t think that’s correct. Unless you just mean “hey look these numbers are the same!” which doesn’t really mean anything by itself.
I mean, you talk about just taking the p-value into account rather than the base rate, but it’s not at all clear to me that the way you do so is meaningful. Just considering the equation P(H|E)=P(E|H)*P(H)/P(E), you’re suggesting that we “don’t take into account base rate” by assuming P(H)/P(E) is about 1? I really don’t see what makes such an assumption reasonable.
Now if you want to say, “Let’s not worry about what P(H) is, and so just assume P(H)/P(E) is some constant”, that might make more sense. But then you can’t get any particular number out of it.
Thanks for looking into the 90% thing. Ioannidis misspelled as “Ioannides” throughout.
What percentage of studies that contradict famous studies with positive findings are false?
I believe, although I haven’t checked, that the studies Ioannidis looked at were always larger or otherwise better than the original studies being tested (since this would be the only sensible approach); hence, if there’s a disagreement, either way….
For what it’s worth, I don’t know of anyone at MetaMed who has ever claimed that 90% of studies are false, so I think your first sentence might be straw manning. Me and several others have claimed that 80% are false, but that’s much more in line with his actual results, as you note.
Do feel like I’ve heard the 90% number somewhere, possibly somewhere nonofficial in private conversation with someone, but unless I can track down a source I’ll edit it out with apologies. I still think “80% of non-experimental studies” is a pretty big caveat compared to “80% of research” but I have no idea how this was phrased and for all I know you said it that way. Sorry about that.
Your link to the 90% claim:
http://therefusers.com/refusers-newsroom/90-of-peer-reviewed-clinical-research-is-completely-false-greenmedinfo/#.VmLbj_mrTNN
I thought a lot of the point of MetaMed was to find sound but neglected research– at least as much that as debunking bad research.
Pingback: Future tense | Slate Star Codex
Pingback: MetaMed launch day | Slate Star Codex
> Of these, 4 were negative results (ie “X doesn’t work”) so he threw them out. This is the first part I think is kind of unfair.
I disagree. Negative results don’t show that an effect isn’t there. They just show that it’s too small to see with that sample size. A negative result being later disproven does not show a flaw in the original study.
If you show that the effect is there and is large enough that the first study shouldn’t have missed it, that’s a problem, but it makes this way more complicated so it’s easier just to ignore those studies.
Thinking about this more, I guess they’d have to do something like this either way, to show that the study didn’t just fail to replicate because the second study had a false negative. Either they’d have to look at ones where the study where it fails is much more powerful, or they’d have to use a two-tailed T-test and show that the two studies shouldn’t result from the same effect.
Pingback: The Problem With Connection Theory | The Rationalist Conspiracy
Here is a 90% number: “He (Ioannidis) charges that as much as 90 percent of the published medical information that doctors rely on is flawed.”
http://www.theatlantic.com/magazine/archive/2010/11/lies-damned-lies-and-medical-science/308269/